EasySO: Exploration-enhanced Reinforcement Learning for Logic Synthesis Sequence Optimization and a Comprehensive RL Environment
2023 IEEE/ACM INTERNATIONAL CONFERENCE ON COMPUTER AIDED DESIGN, ICCAD(2023)
摘要
Optimizing the quality of results (QoR) of a circuit during the logic synthesis (LS) phase in chip design is critical yet challenging. While most existing methods often mitigate the computational hardness by restricting the action space to a small set of operators and fixing the operator's parameters, they are susceptible to local minima and may not meet the high demand from industrial cases. In this paper, we develop a more comprehensive optimization approach via sample-efficient reinforcement learning (RL). Specifically, we first build a complete logic synthesis-RL environment, where the action space consists of three types of operators: logic optimization, technology mapping, and post-mapping, along with their associated continuous/binary parameters for optimization as well. Based on this environment, we devise a hybrid proximal policy optimization (PPO) model to handle both discrete operators and parameters and design a distributed architecture to improve sample collection efficiency. Furthermore, we devise a dynamic exploration module to improve the exploration efficiency under the constraint of limited samples. We term our method as Exploration-enhanced RL for Logic Synthesis Sequence Optimization(EasySO). Results on the EPFL benchmark show that our method significantly outperforms current state-of-the-art models based on Bayesian optimization (BO) and the previous RL-based methods. Compared to resyn2, our EasySO achieves an average of 25.4% LUT-6 count optimization without sacrificing level values. Moreover, as of the time for this submission, we rank 26 first places among 40 optimization targets in the EPFL competition.
更多查看译文
AI 理解论文
溯源树
样例
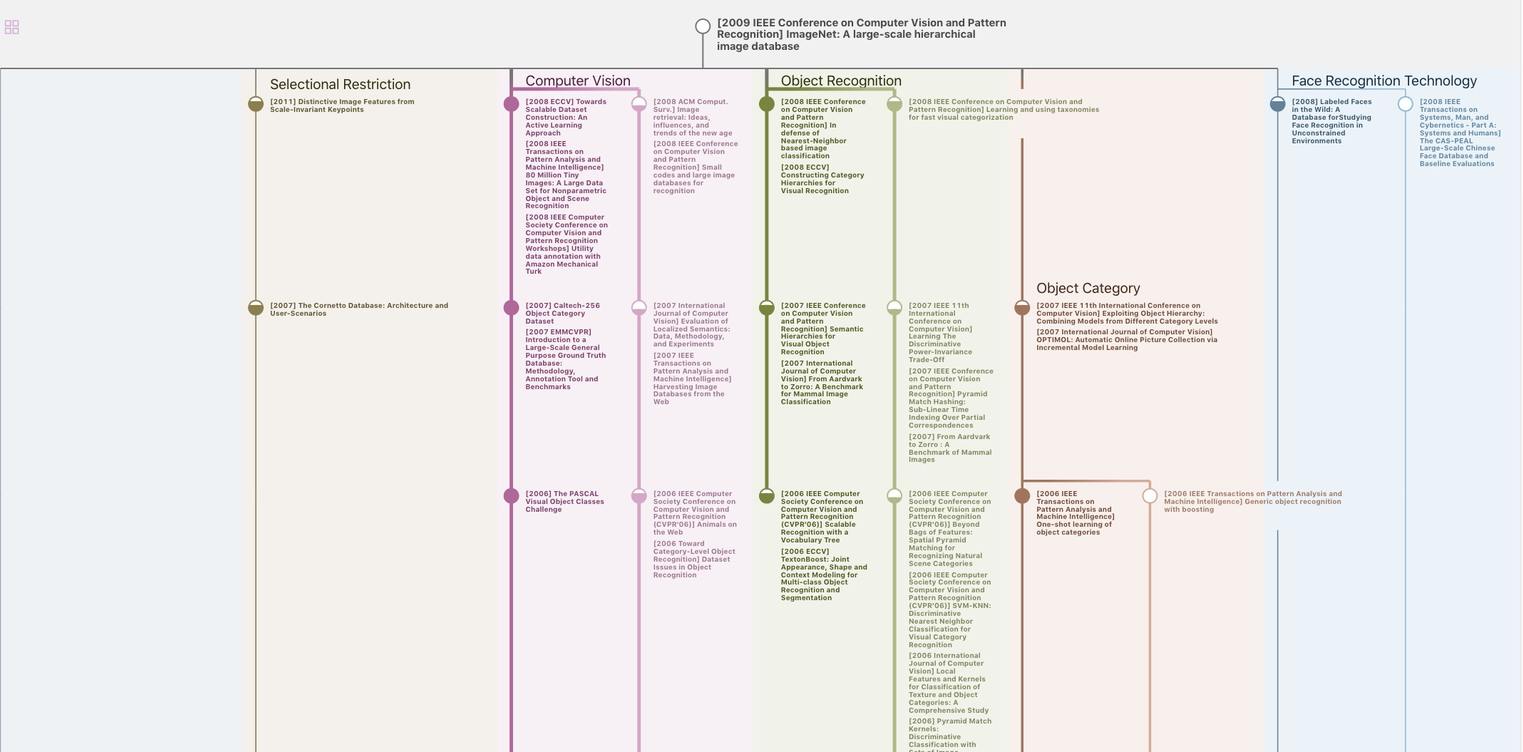
生成溯源树,研究论文发展脉络
Chat Paper
正在生成论文摘要