(AF)2-S3Net: Attentive Feature Fusion with Adaptive Feature Selection for Sparse Semantic Segmentation Network
2021 IEEE/CVF Conference on Computer Vision and Pattern Recognition (CVPR)(2021)
摘要
Autonomous robotic systems and self driving cars rely on accurate perception of their surroundings as the safety of the passengers and pedestrians is the top priority. Semantic segmentation is one of the essential components of road scene perception that provides semantic information of the surrounding environment. Recently, several methods have been introduced for 3D LiDAR semantic segmentation. While they can lead to improved performance, they are either afflicted by high computational complexity, therefore are inefficient, or they lack fine details of smaller object instances. To alleviate these problems, we propose (AF)
2
-S3Net, an end-to-end encoder-decoder CNN network for 3D LiDAR semantic segmentation. We present a novel multibranch attentive feature fusion module in the encoder and a unique adaptive feature selection module with feature map re-weighting in the decoder. Our (AF)
2
-S3Net fuses the voxel-based learning and point-based learning methods into a unified framework to effectively process the potentially large 3D scene. Our experimental results show that the proposed method outperforms the state-of-the-art approaches on the large-scale nuScenes-lidarseg and SemanticKITTI benchmark, ranking 1
st
on both competitive public leaderboard competitions upon publication.
更多查看译文
关键词
sparse semantic segmentation network,self driving cars,road scene perception,3D LiDAR semantic segmentation,end-to-end encoder-decoder CNN network,multibranch attentive feature fusion,feature map re-weighting,adaptive feature selection,voxel-based learning,3D scene,(AF)2-S3Net fusion,point-based learning,autonomous robotic systems,passenger safety,pedestrian safety,large-scale nuScenes-lidarseg,SemanticKITTI benchmark,high computational complexity
AI 理解论文
溯源树
样例
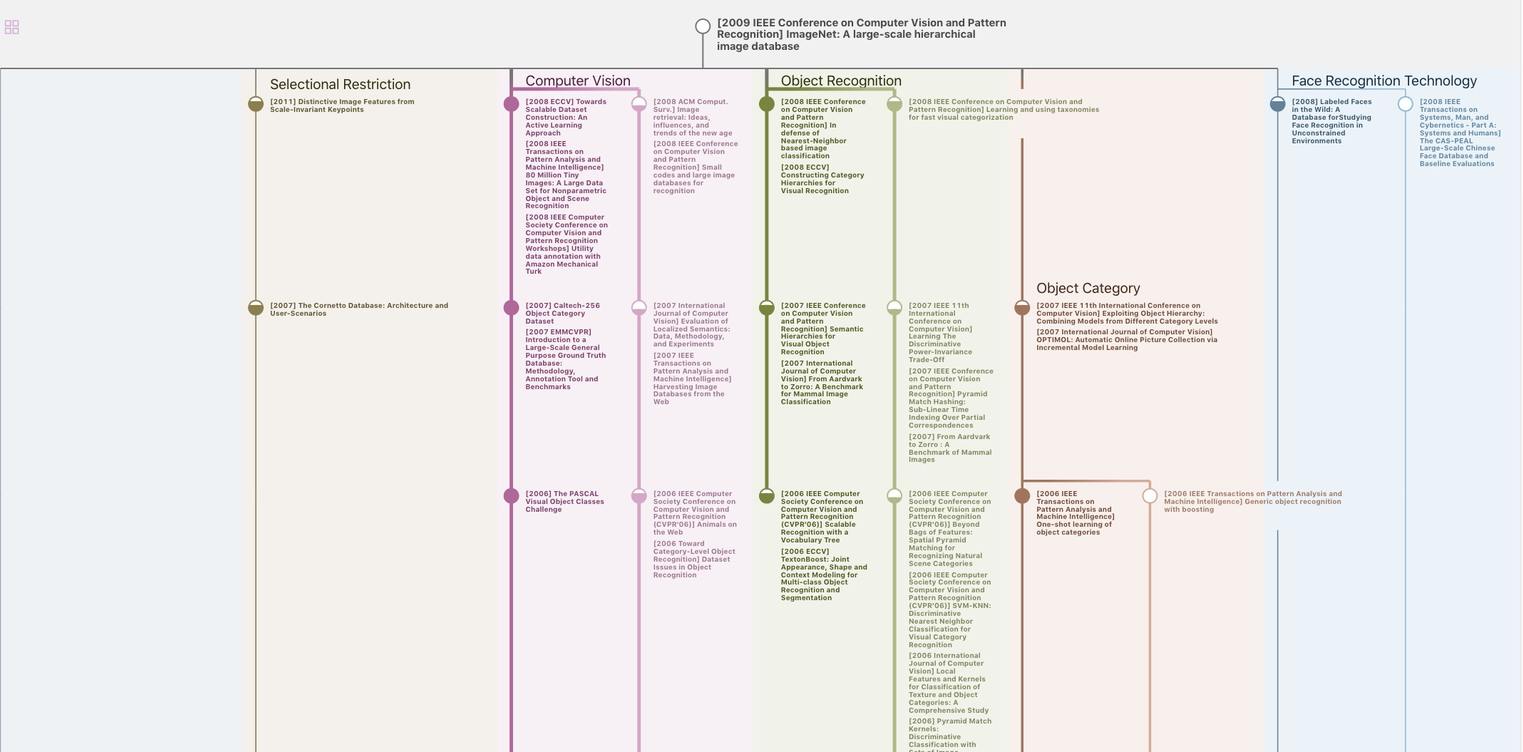
生成溯源树,研究论文发展脉络
Chat Paper
正在生成论文摘要