33.4 A 28nm 2Mb STT-MRAM Computing-in-Memory Macro with a Refined Bit-Cell and 22.4 - 41.5TOPS/W for AI Inference
2023 IEEE International Solid-State Circuits Conference (ISSCC)(2023)
摘要
Emerging non-volatile memory-based computing-in-memory (CIM) is an excellent fit for resource-constrained edge-AI devices [1–6]. MRAM-CIM macros for MAC operations, at present, rely on a crossbar structure or a peripheral circuit modification [2], [3]. It remains a great challenge for bottom-up design of MRAM-CIM macro using the standard one transistor - one magnetic tunnel junction (1T-1MTJ) bit-cell: (1) The mainstream spin-transfer-torque (STT) switching mechanism with a standard foundry bit-cell cannot fulfill CIM operation requirements in binary neural networks (BNN). (2) The excessive multi-row/column activation method suffers from a limited read window due to the limited tunnel magnetoresistance ratio and process variation [1], [2]. (3) Prior MRAM-CIMs rely on analog domain computing, for which an analog-to-digital converter is required with it's associated high energy consumption and area overhead [3], [6].
更多查看译文
关键词
1T-1MTJ bit-cell,AI inference,analog domain computing,analog-to-digital converter,binary neural networks,BNN,current 33.4 A,energy consumption,MAC operations,MRAM-CIM macro,nonvolatile memory,one transistor-one magnetic tunnel junction bit-cell,peripheral circuit modification,refined bit-cell,resource-constrained edge-AI devices,size 28.0 nm,spin-transfer-torque switching mechanism,standard foundry bit-cell,STT-MRAM computing-in-memory macro,tunnel magnetoresistance ratio
AI 理解论文
溯源树
样例
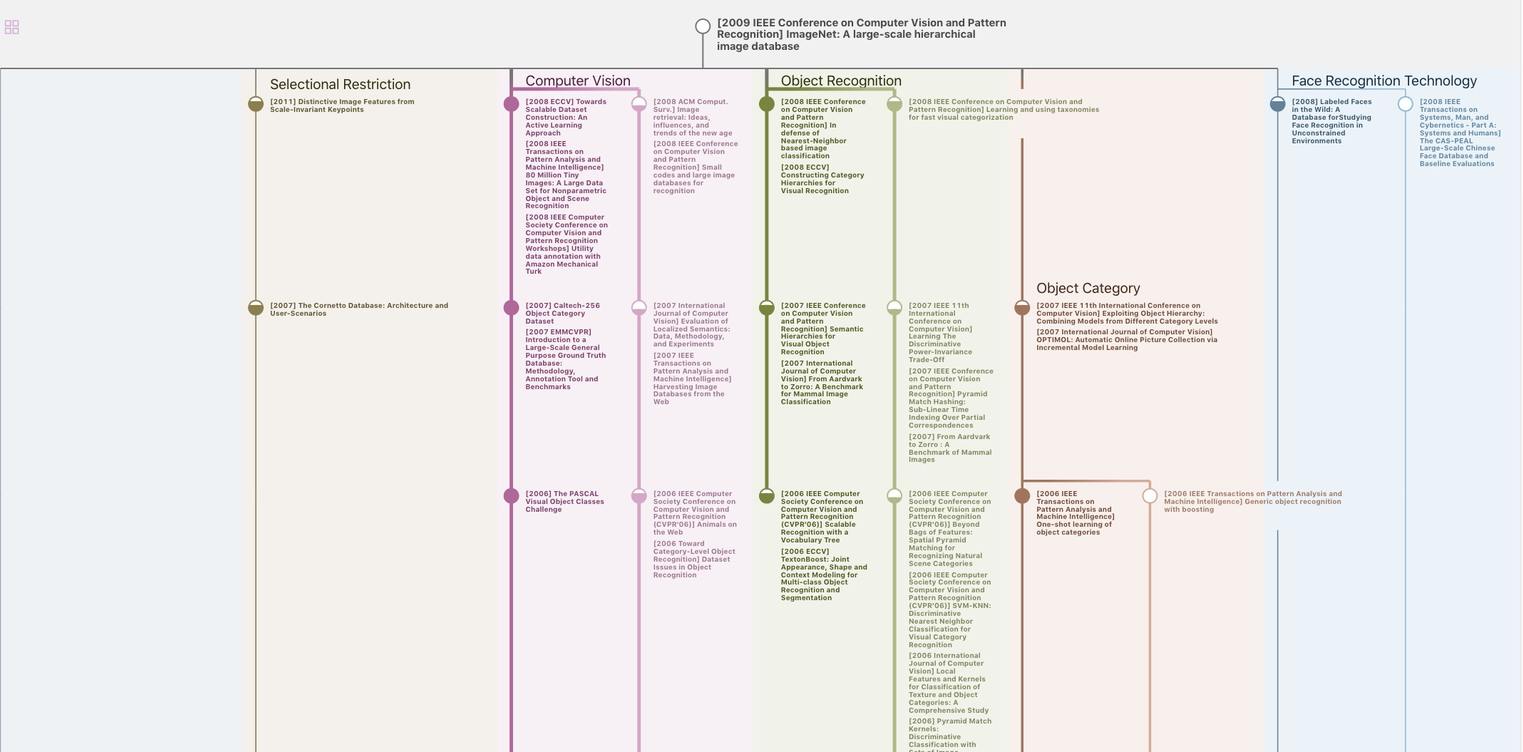
生成溯源树,研究论文发展脉络
Chat Paper
正在生成论文摘要