RigLSTM: Recurrent Independent Grid LSTM for Generalizable Sequence Learning.
CoRR(2023)
摘要
Sequential processes in real-world often carry a combination of simple subsystems that interact with each other in certain forms. Learning such a modular structure can often improve the robustness against environmental changes. In this paper, we propose recurrent independent Grid LSTM (RigLSTM), composed of a group of independent LSTM cells that cooperate with each other, for exploiting the underlying modular structure of the target task. Our model adopts cell selection, input feature selection, hidden state selection, and soft state updating to achieve a better generalization ability on the basis of the recent Grid LSTM for the tasks where some factors differ between training and evaluation. Specifically, at each time step, only a fraction of cells are activated, and the activated cells select relevant inputs and cells to communicate with. At the end of one time step, the hidden states of the activated cells are updated by considering the relevance between the inputs and the hidden states from the last and current time steps. Extensive experiments on diversified sequential modeling tasks are conducted to show the superior generalization ability when there exist changes in the testing environment. Source code is available at \url{https://github.com/ziyuwwang/rig-lstm}.
更多查看译文
关键词
recurrent independent grid riglstm,generalizable sequence
AI 理解论文
溯源树
样例
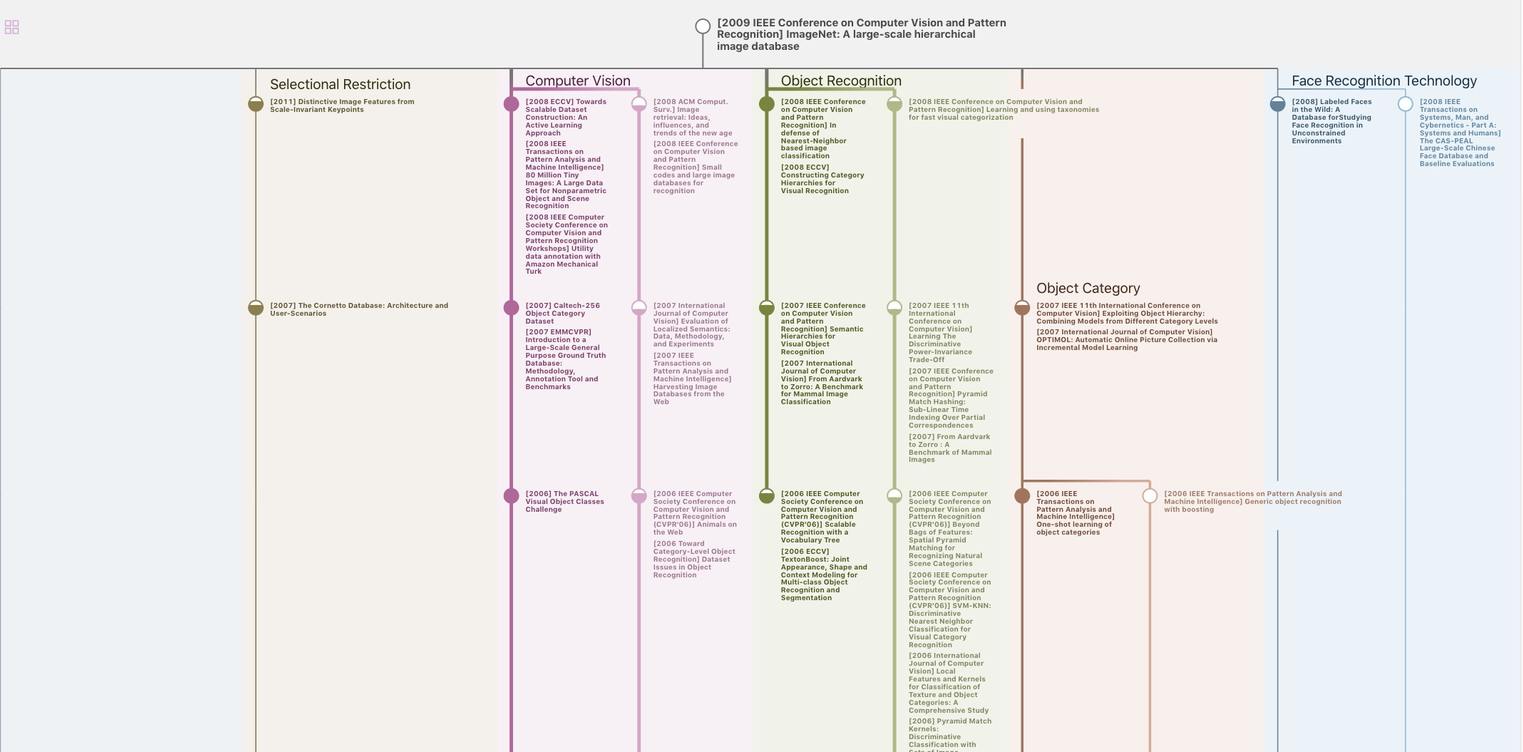
生成溯源树,研究论文发展脉络
Chat Paper
正在生成论文摘要