A Novel Temporal Channel Enhancement and Contextual Excavation Network for Temporal Action Localization
MM '23: Proceedings of the 31st ACM International Conference on Multimedia(2023)
摘要
The temporal action localization (TAL) task aims to locate and classify action instances in untrimmed videos. Most previous methods use classifiers and locators to act on the same feature; thus, the classification and localization processes are relatively independent. Therefore, if the classification results and localization results are fused, there will be a problem that the classification results are correct while the localization results are wrong, resulting in inaccurate final results, and vice versa. To solve this problem, we propose a novel temporal channel enhancement and contextual excavation network (TCN) for the TAL task, which generates robust classification and localization features and refines the final localization results. Specifically, a temporal channel enhancement module is designed to enhance the temporal and channel information of the feature sequence. Then, the temporal semantic contextual excavation module is developed to establish relationships between similar frames. Finally, the features with enhanced contextual information are transferred to a classifier. While executing the classification process, we obtain powerful classification features. Most importantly, with the robust classification features, the final localization features are produced by the refine localization module, which is applied to obtain the final localization results. Extensive experiments show that TCN can outperform all the SOTA methods on the THUMOS14 dataset, and achieves a comparable performance on the ActivityNet1.3 dataset. Compared with ActionFormer (ECCV 2022) and BREM (MM 2022) on the THUMOS14 dataset, the proposed TCN can achieve improvements of 1.8% and 5.0%, respectively.
更多查看译文
AI 理解论文
溯源树
样例
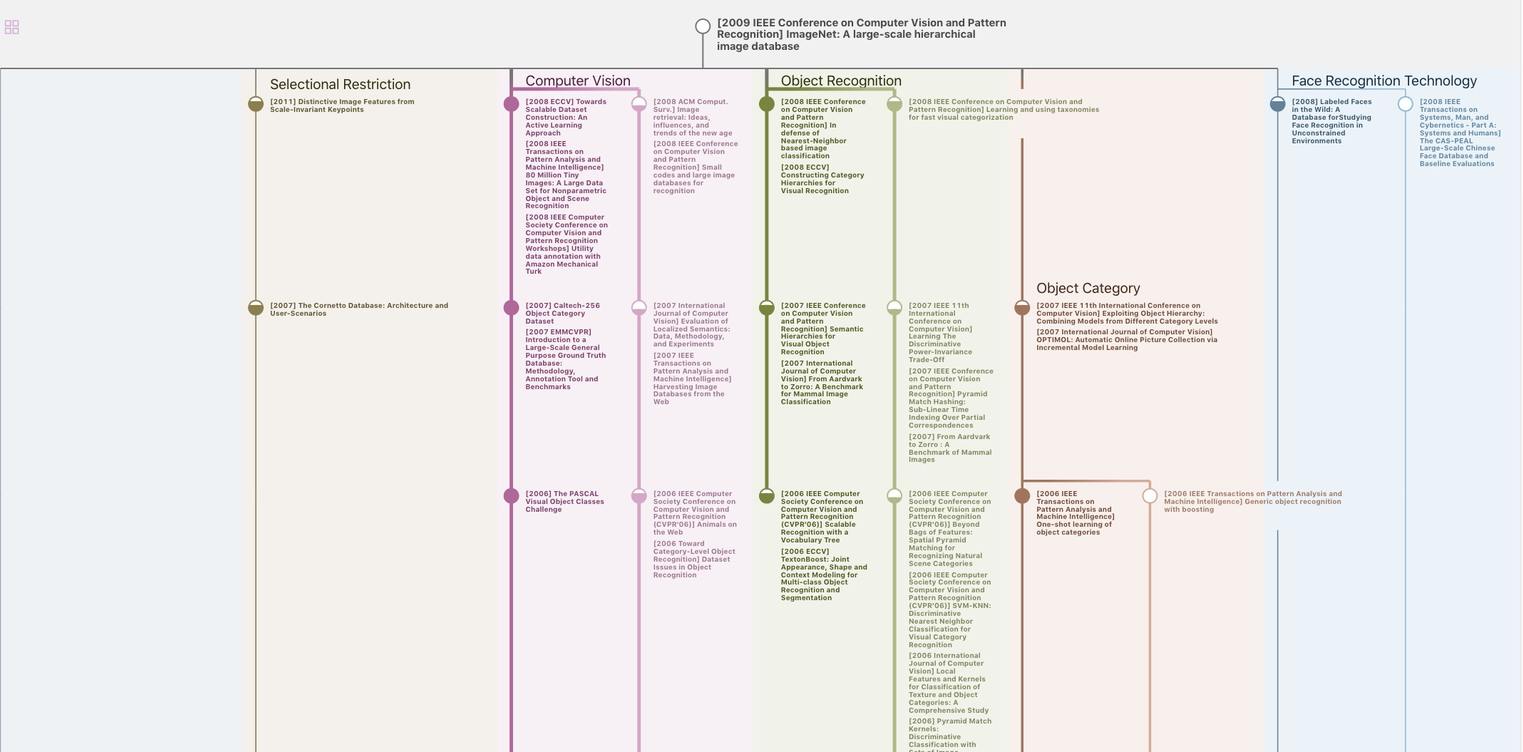
生成溯源树,研究论文发展脉络
Chat Paper
正在生成论文摘要