Learning Custom Experience Ontologies via Embedding-based Feedback Loops.
UIST '23: Proceedings of the 36th Annual ACM Symposium on User Interface Software and Technology(2023)
摘要
Organizations increasingly rely on behavioral analytics tools like Google Analytics to monitor their digital experiences. Making sense of the data these tools capture, however, requires manual event tagging and filtering — often a tedious process. Prior approaches have trained machine learning models to automatically tag interaction data, but draw from fixed digital experience vocabularies which cannot be easily augmented or customized. This paper introduces a novel machine learning interaction pattern that generates customized tag predictions for organizations. The approach employs a general user experience word embedding to bootstrap an initial set of predictions, which can then be refined and customized by users to adapt the underlying vector space, iteratively improving the quality of future predictions. The paper presents a needfinding study that grounds the design choices of the system, and describes a real-world deployment as part of UserTesting.com that demonstrates the efficacy of the approach.
更多查看译文
AI 理解论文
溯源树
样例
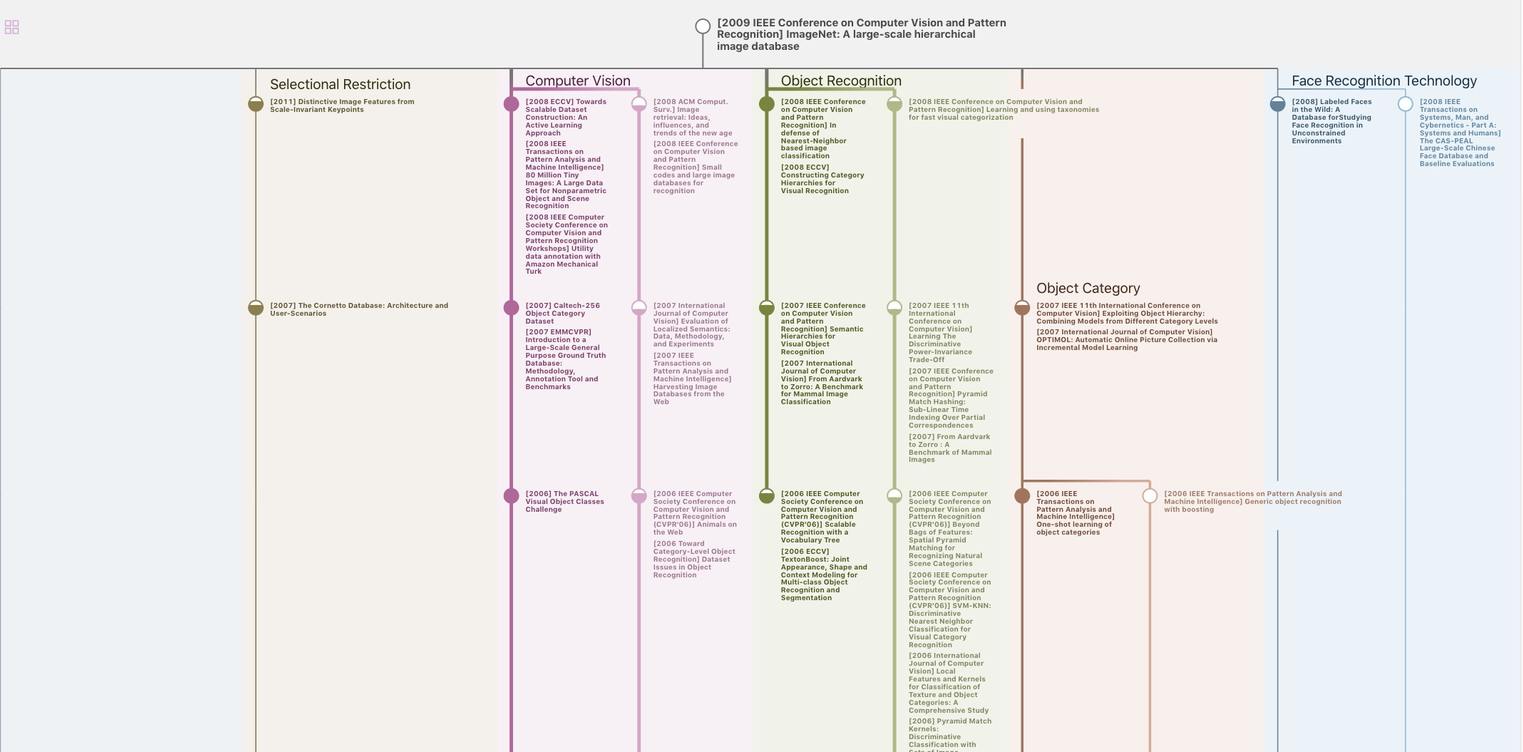
生成溯源树,研究论文发展脉络
Chat Paper
正在生成论文摘要