A Cross-Entropy Motion Planning Framework for Hybrid Continuum Robots
IEEE ROBOTICS AND AUTOMATION LETTERS(2023)
摘要
The sampling-based motion planners, including the Rapidly-exploring Random Trees (RRT) algorithms, are widely utilized in continuum robots, enabling efficient search for feasible motion plans in constrained environments. In surgical robotics, complex mapping among the high-dimensional kinematics of continuum robots, trajectory parameterization, and path redundancy may lead to non-optimal motion path, which in turn affects their efficiency and surgical task performance (e.g. path following), and ultimately the patient outcome. In this letter, a cross-entropy (CE) motion planning framework is proposed for continuum robots, wherein the RRT* planner is equipped with a CE estimation method serving as a probabilistic model to sample elite trajectories with optimal computation costs. It can asymptotically optimize the sampling distributions among individuals in terms of either robot states or parameterized trajectories. The presented CE motion planners were implemented on a hybrid continuum robot to enable obstacle avoidance, approximate follow-the-leader (FTL) motion, and navigation in a clinical scenario. They are shown to offer lower sampling cost and higher computational efficiency compared to existing approaches.
更多查看译文
关键词
Robots,Robot kinematics,Electron tubes,Planning,Motion segmentation,Kinematics,Trajectory,Continuum robots,sampling-based motion planning,cross-entropy (CE) motion planning,Rapidly-exploring Random Trees (RRT*) approach
AI 理解论文
溯源树
样例
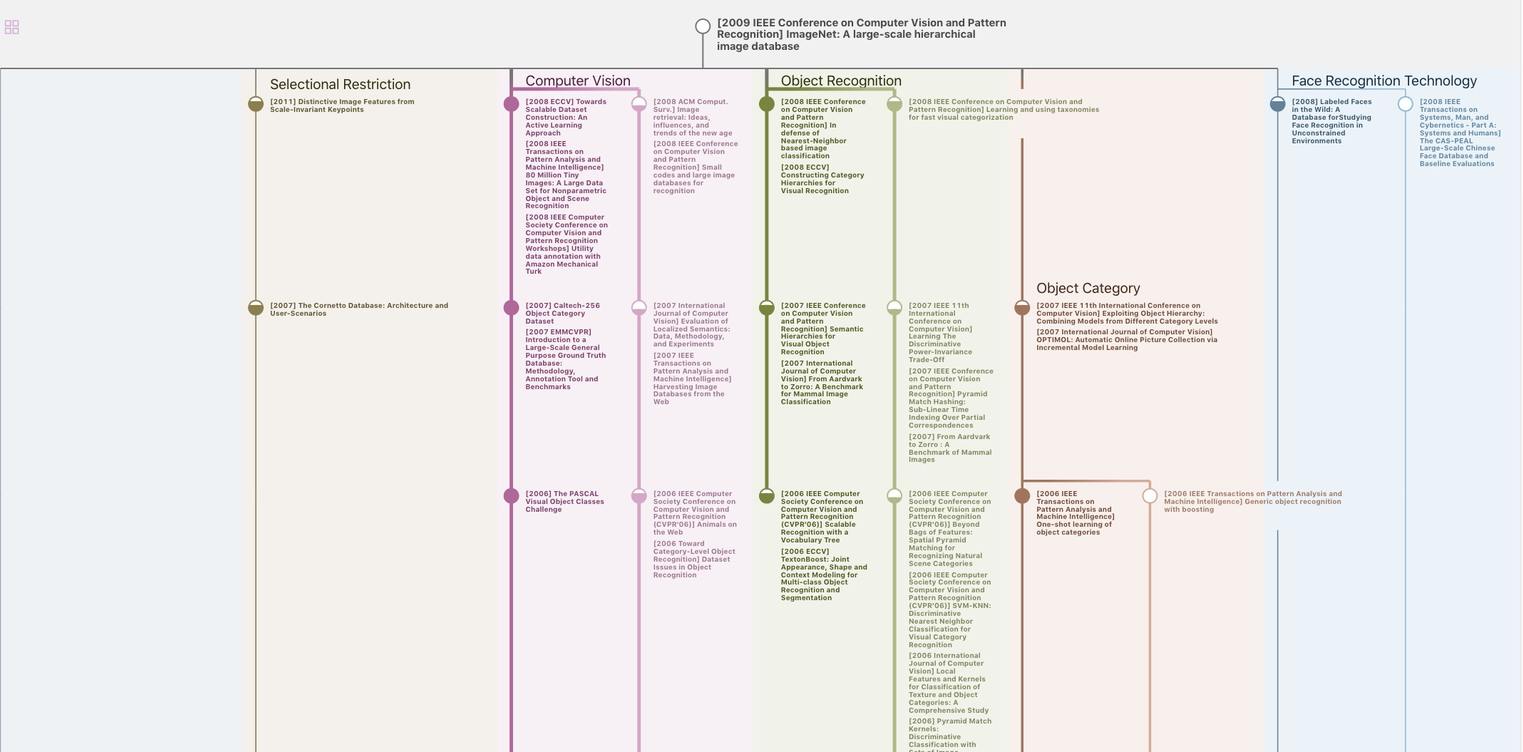
生成溯源树,研究论文发展脉络
Chat Paper
正在生成论文摘要