Which Examples to Annotate for In-Context Learning? Towards Effective and Efficient Selection
CoRR(2023)
摘要
Large Language Models (LLMs) can adapt to new tasks via in-context learning (ICL). ICL is efficient as it does not require any parameter updates to the trained LLM, but only few annotated examples as input for the LLM. In this work, we investigate an active learning approach for ICL, where there is a limited budget for annotating examples. We propose a model-adaptive optimization-free algorithm, termed AdaICL, which identifies examples that the model is uncertain about, and performs semantic diversity-based example selection. Diversity-based sampling improves overall effectiveness, while uncertainty sampling improves budget efficiency and helps the LLM learn new information. Moreover, AdaICL poses its sampling strategy as a Maximum Coverage problem, that dynamically adapts based on the model's feedback and can be approximately solved via greedy algorithms. Extensive experiments on nine datasets and seven LLMs show that AdaICL improves performance by 4.4% accuracy points over SOTA (7.7% relative improvement), is up to 3x more budget-efficient than performing annotations uniformly at random, while it outperforms SOTA with 2x fewer ICL examples.
更多查看译文
关键词
annotate,learning,examples,in-context
AI 理解论文
溯源树
样例
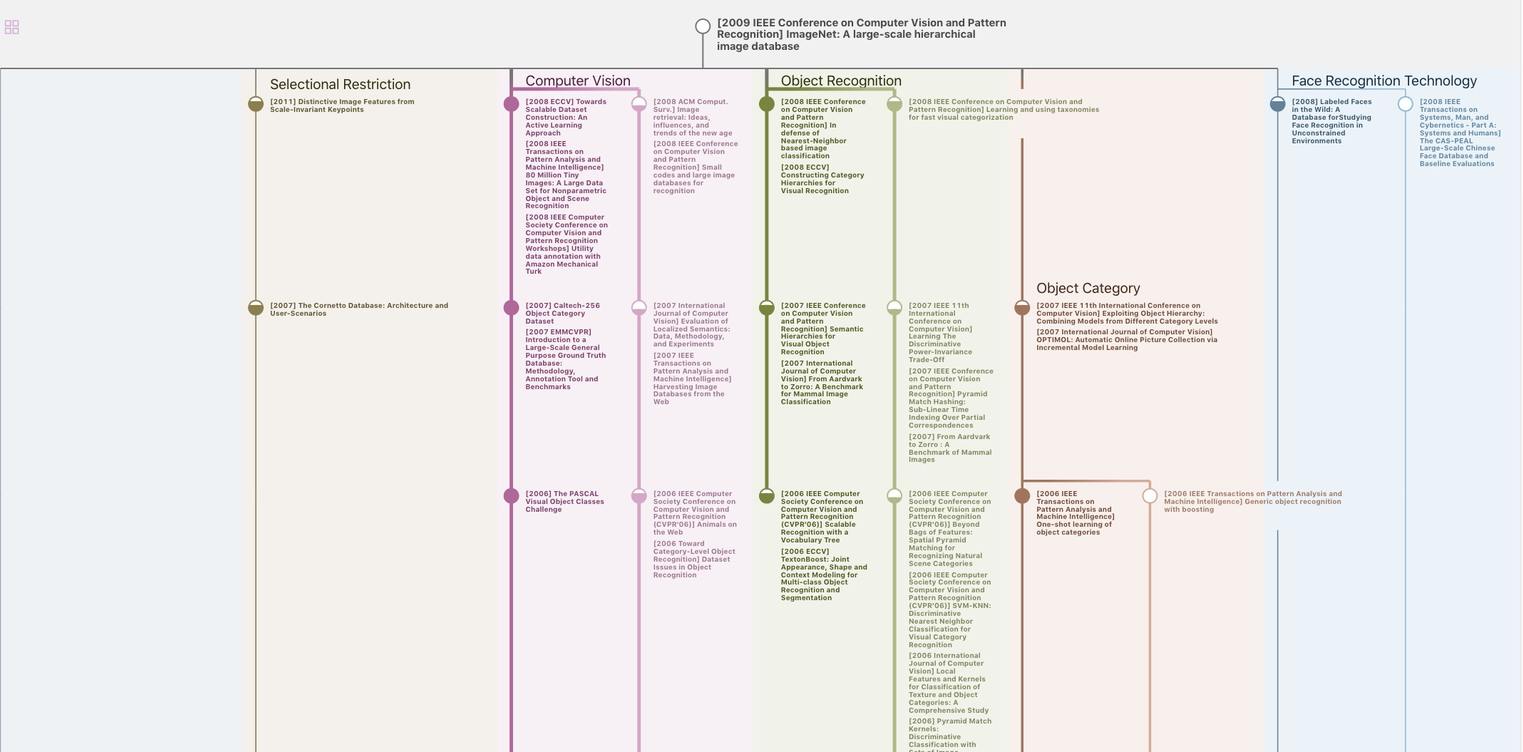
生成溯源树,研究论文发展脉络
Chat Paper
正在生成论文摘要