Exploration of DPP-IV Inhibitory Peptide Design Rules Assisted by the Deep Learning Pipeline That Identifies the Restriction Enzyme Cutting Site
ACS OMEGA(2023)
摘要
The mining of antidiabetic dipeptidyl peptidase IV (DPP-IV) inhibitory peptides (DPP-IV-IPs) is currently a costly and laborious process. Due to the absence of rational peptide design rules, it relies on cumbersome screening of unknown enzyme hydrolysates. Here, we present an enhanced deep learning model called bidirectional encoder representation (BERT)-DPPIV, specifically designed to classify DPP-IV-IPs and explore their design rules to discover potent candidates. The end-to-end model utilizes a fine-tuned BERT architecture to extract structural/functional information from input peptides and accurately identify DPP-IV-Ips from input peptides. Experimental results in the benchmark data set showed BERT-DPPIV yielded state-of-the-art accuracy and MCC of 0.894 and 0.790, surpassing the 0.797 and 0.594 obtained by the sequence-feature model. Furthermore, we leveraged the attention mechanism to uncover that our model could recognize the restriction enzyme cutting site and specific residues that contribute to the inhibition of DPP-IV. Moreover, guided by BERT-DPPIV, proposed design rules for DPP-IV inhibitory tripeptides and pentapeptides were validated, and they can be used to screen potent DPP-IV-IPs.
更多查看译文
关键词
inhibitory peptide design rules,deep learning pipeline,enzyme,restriction
AI 理解论文
溯源树
样例
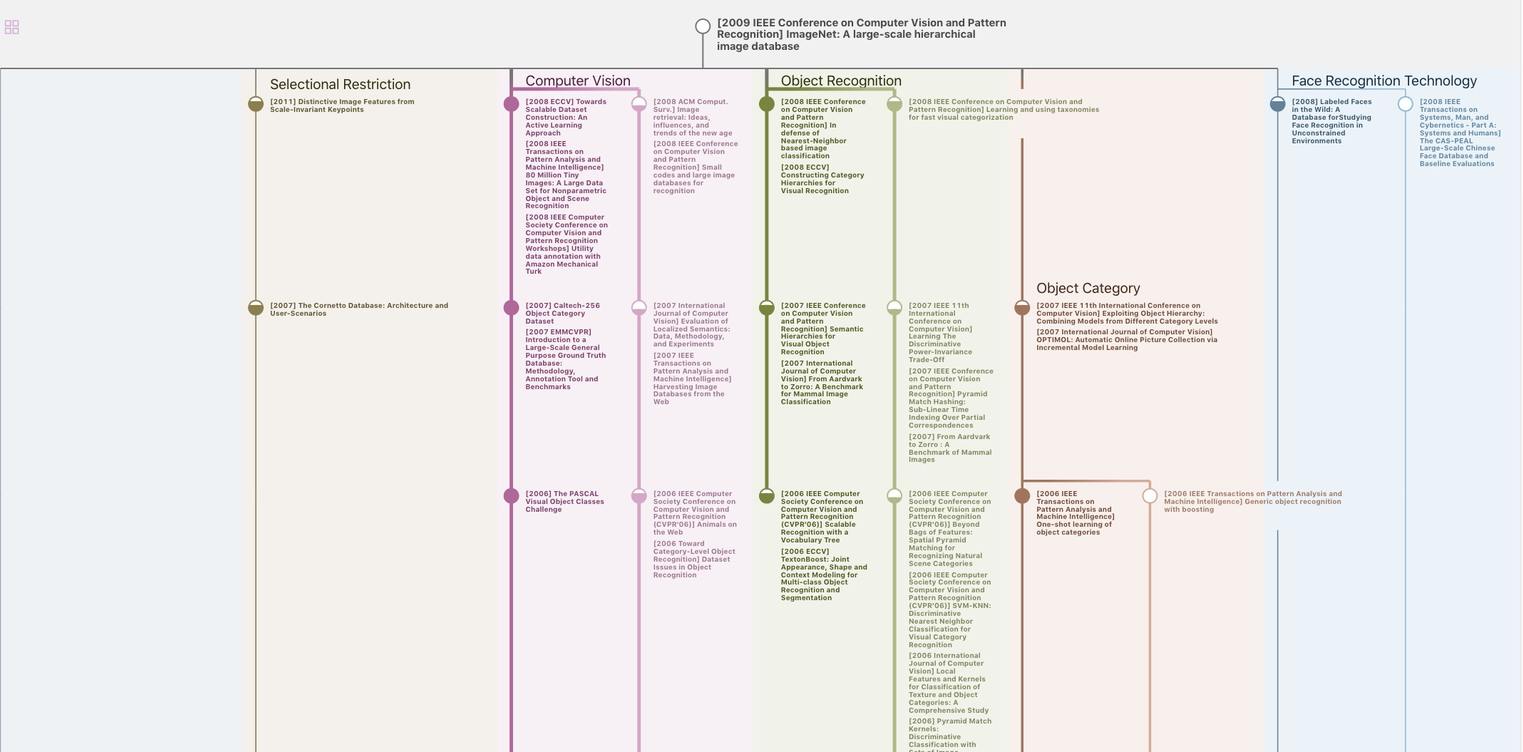
生成溯源树,研究论文发展脉络
Chat Paper
正在生成论文摘要