Gaussian Embedding of Temporal Networks
IEEE Access(2023)
摘要
Representing the nodes of continuous-time temporal graphs in a low-dimensional latent space has wide-ranging applications, from prediction to visualization. Yet, analyzing continuous-time relational data with timestamped interactions introduces unique challenges due to its sparsity. Merely embedding nodes as trajectories in the latent space overlooks this sparsity. However, a natural way to account for this sparsity is to model the uncertainty around the latent positions. In this paper, we propose TGNE (Temporal Gaussian Network Embedding), an innovative method that bridges two distinct strands of literature: the statistical analysis of networks via Latent Space Models (LSM) and temporal graph machine learning. TGNE embeds nodes as piece-wise linear trajectories of Gaussian distributions in the latent space, capturing both structural information and uncertainty around the trajectories. We evaluate TGNE’s effectiveness in reconstructing the original graph and modelling uncertainty. The results demonstrate that TGNE generates time-varying embedding locations that can accurately reconstruct missing parts of the network based on observed ones. Furthermore, the uncertainty estimates align experimentally with the time-varying degree distribution in the network, providing valuable insights into the temporal dynamics of the graph. To facilitate reproducibility, we provide an open-source implementation of TGNE at https://github.com/aida-ugent/tgne/.
更多查看译文
关键词
Trajectory, Uncertainty, Statistical analysis, Knowledge graphs, Bayes methods, Task analysis, Random variables, Representation learning, Temporal networks, latent space models, variational inference, representation learning, dimensionality reduction, networks, random graphs
AI 理解论文
溯源树
样例
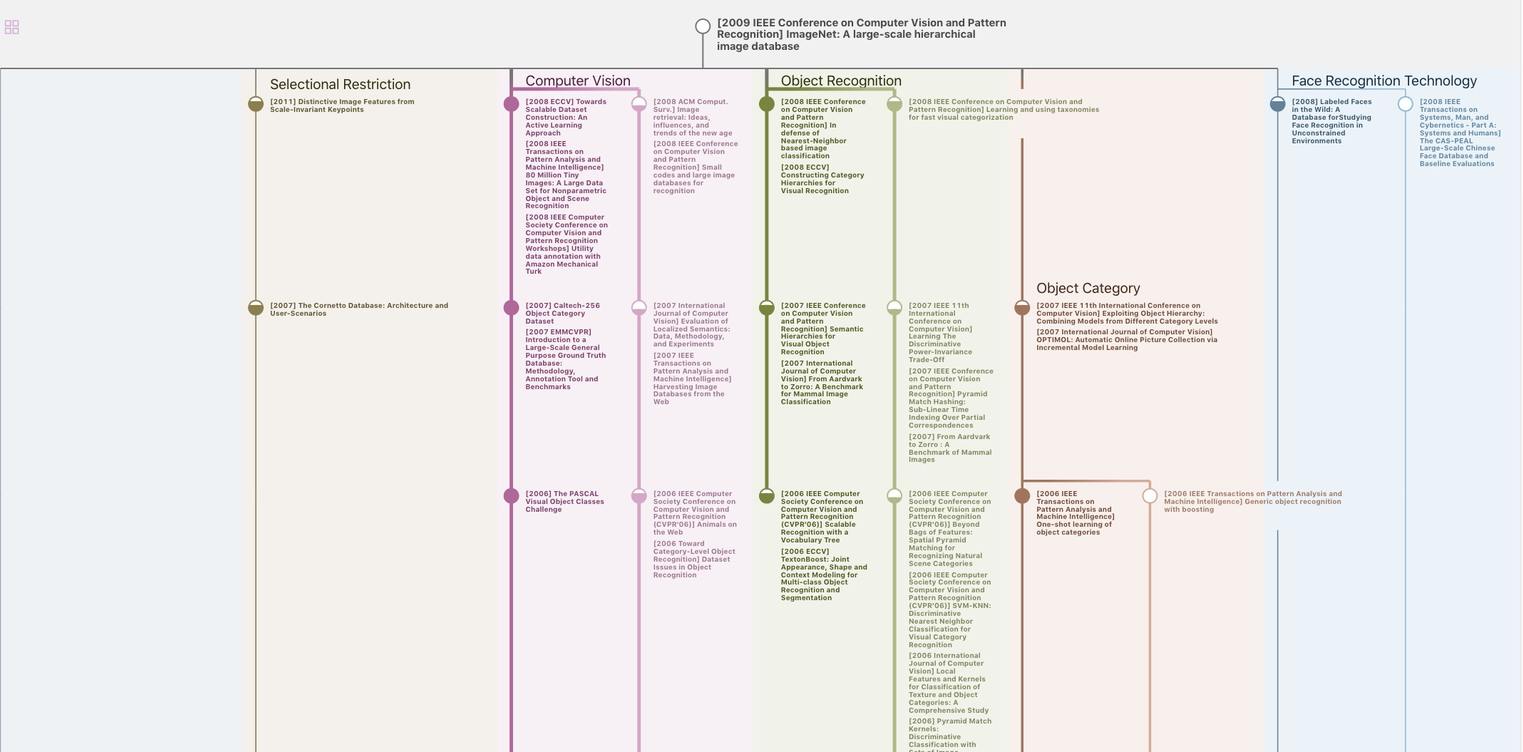
生成溯源树,研究论文发展脉络
Chat Paper
正在生成论文摘要