Generative Adversarial Networks in Digital Histopathology: Current Applications, Limitations, Ethical Considerations, and Future Directions
MODERN PATHOLOGY(2024)
摘要
Generative adversarial networks (GANs) have gained significant attention in the field of image synthesis, particularly in computer vision. GANs consist of a generative model and a discriminative model trained in an adversarial setting to generate realistic and novel data. In the context of image synthesis, the generator produces synthetic images, whereas the discriminator determines their authenticity by comparing them with real examples. Through iterative training, the generator allows the creation of images that are indistinguishable from real ones, leading to high-quality image generation. Considering their success in computer vision, GANs hold great potential for medical diagnostic applications. In the medical field, GANs can generate images of rare diseases, aid in learning, and be used as visualization tools. GANs can leverage unlabeled medical images, which are large in size, numerous in quantity, and challenging to annotate manually. GANs have demonstrated remarkable capabilities in image synthesis and have the potential to significantly impact digital histopathology. This review article focuses on the emerging use of GANs in digital histopathology, examining their applications and potential challenges. Histopathology plays a crucial role in disease diagnosis, and GANs can contribute by generating realistic microscopic images. However, ethical considerations arise because of the reliance on synthetic or pseudogenerated images. Therefore, the manuscript also explores the current limitations and highlights the ethical considerations associated with the use of this technology. In conclusion, digital histopathology has seen an emerging use of GANs for image enhancement, such as color (stain) normalization, virtual staining, and ink/marker removal. GANs offer significant potential in transforming digital pathology when applied to specific and narrow tasks (preprocessing enhancements). Evaluating data quality, addressing biases, protecting privacy, ensuring accountability and transparency, and developing regulation are imperative to ensure the ethical application of GANs.(c) 2023 United States & Canadian Academy of Pathology. Published by Elsevier Inc. All rights reserved.
更多查看译文
关键词
artificial intelligence,deep learning,image segmentation,generative adversarial networks,histopathology,digital histopathology,ethical considerations
AI 理解论文
溯源树
样例
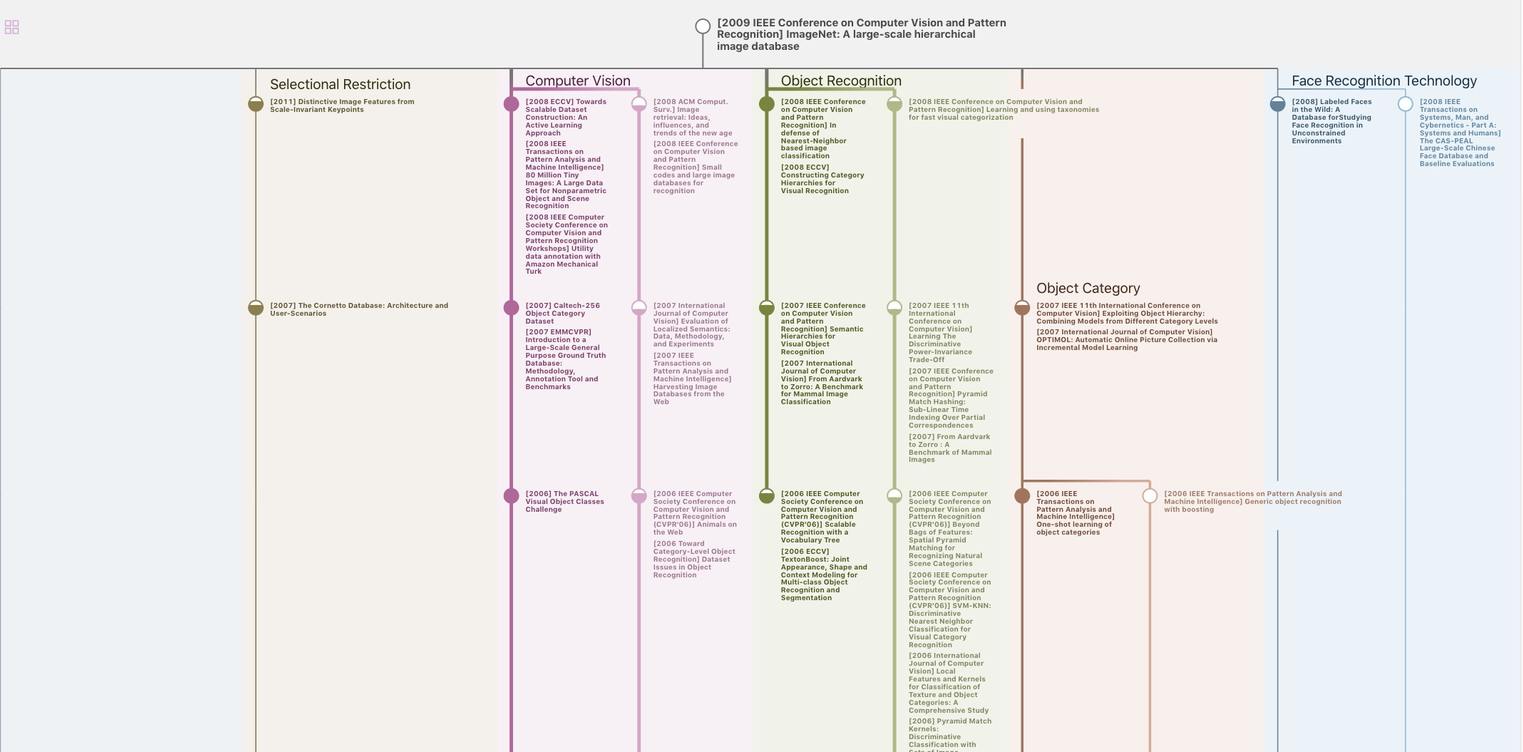
生成溯源树,研究论文发展脉络
Chat Paper
正在生成论文摘要