Conditionally Combining Robot Skills using Large Language Models
CoRR(2023)
摘要
This paper combines two contributions. First, we introduce an extension of the Meta-World benchmark, which we call "Language-World," which allows a large language model to operate in a simulated robotic environment using semi-structured natural language queries and scripted skills described using natural language. By using the same set of tasks as Meta-World, Language-World results can be easily compared to Meta-World results, allowing for a point of comparison between recent methods using Large Language Models (LLMs) and those using Deep Reinforcement Learning. Second, we introduce a method we call Plan Conditioned Behavioral Cloning (PCBC), that allows finetuning the behavior of high-level plans using end-to-end demonstrations. Using Language-World, we show that PCBC is able to achieve strong performance in a variety of few-shot regimes, often achieving task generalization with as little as a single demonstration. We have made Language-World available as open-source software at https://github.com/krzentner/language-world/.
更多查看译文
关键词
combining robot skills,large language models,language models,large language
AI 理解论文
溯源树
样例
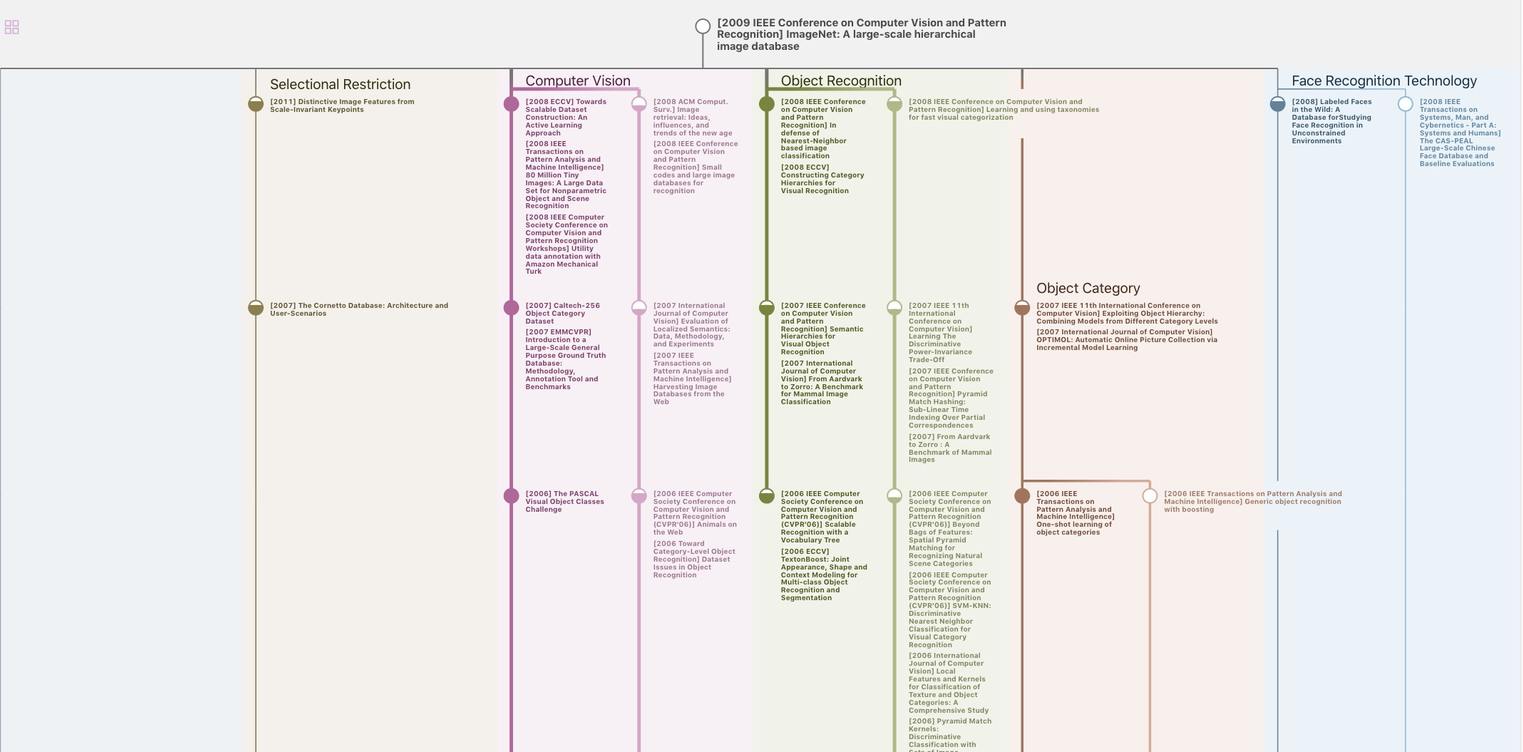
生成溯源树,研究论文发展脉络
Chat Paper
正在生成论文摘要