A parallel-hierarchical neural network (PHNN) for motor imagery EEG signal classification
BIOMEDICAL SIGNAL PROCESSING AND CONTROL(2024)
摘要
Motor imagery brain-computer interfaces (MI-BCIs) play a crucial role in fields such as robot control and stroke rehabilitation. With the flourishing development of deep learning, there has been a continuous emergence of motor imagery deep learning models with higher decoding accuracy. Activation of specific brain regions, such as the motor regions in the frontal and parietal lobes, carries information about motor imagery. However, most studies only extract features from the entire brain region, neglecting the potential features of specific brain regions. This may lead to poorer decoding performance. Therefore, this article proposes a parallel-hierarchical neural network (PHNN), which implements a hierarchical approach to feature learning from brain region level to multi-level fusion. Learning at the brain region level mainly explores the key information of specific brain regions (region-level) and the overall features of the entire brain (global-level), to obtain region-level features (RLF) and global-level features (GLF). Furthermore, given that region-level features contain crucial information within specific brain regions, multi-level fusion is employed to capture and fully utilize the differences and connections between the RLF and GLF, resulting in more discriminative multi-level fusion features (MLFF). We evaluate the model performance on the publicly available BCI Competition IV-2a dataset and High Gamma dataset, achieving recognition accuracies of 84.67% and 94.02%, respectively.
更多查看译文
关键词
Brain-computer interface,Motor imagery,Deep learning,Electroencephalography
AI 理解论文
溯源树
样例
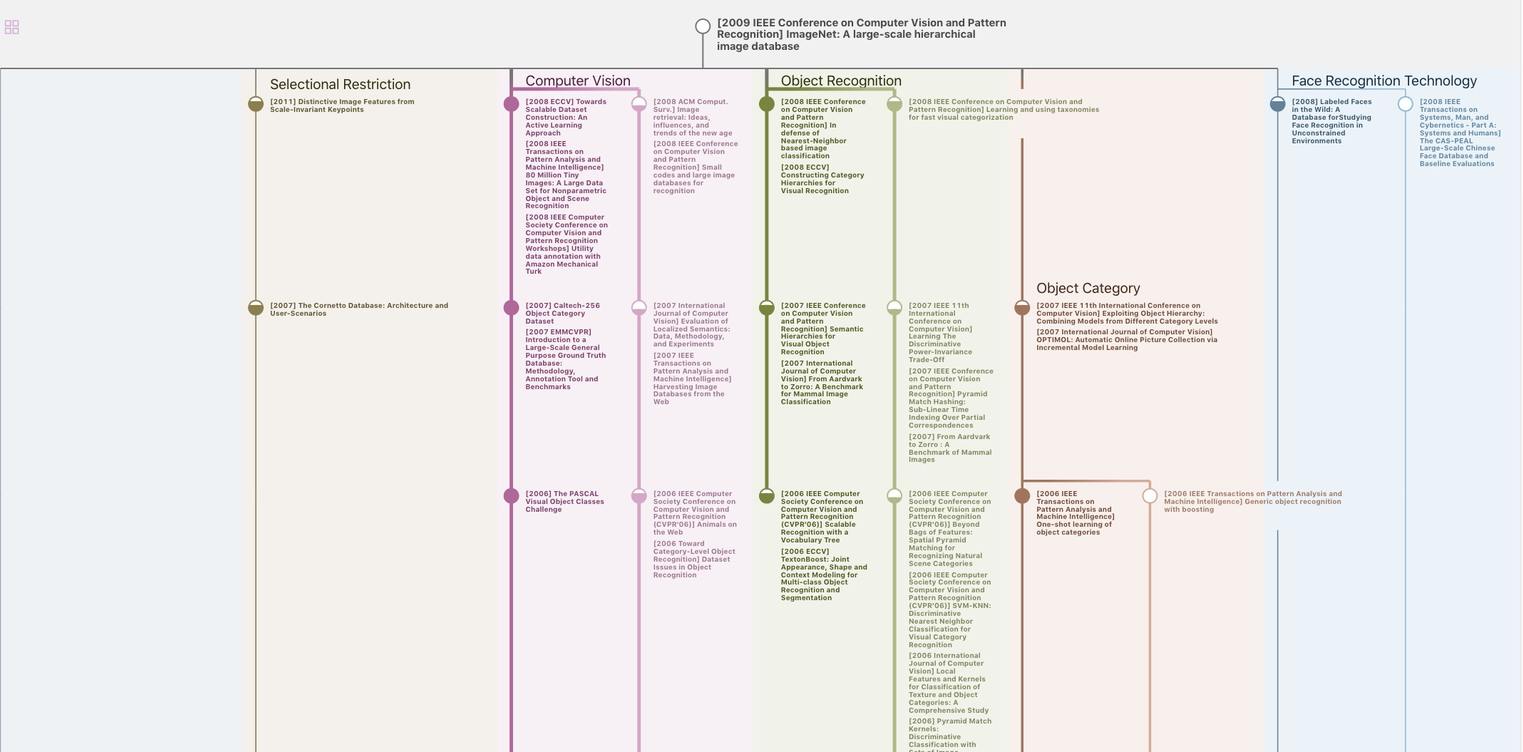
生成溯源树,研究论文发展脉络
Chat Paper
正在生成论文摘要