EKGNet: A 10.96{\mu}W Fully Analog Neural Network for Intra-Patient Arrhythmia Classification
arXiv (Cornell University)(2023)
摘要
We present an integrated approach by combining analog computing and deep learning for electrocardiogram (ECG) arrhythmia classification. We propose EKGNet, a hardware-efficient and fully analog arrhythmia classification architecture that archives high accuracy with low power consumption. The proposed architecture leverages the energy efficiency of transistors operating in the subthreshold region, eliminating the need for analog-to-digital converters (ADC) and static random access memory (SRAM). The system design includes a novel analog sequential Multiply-Accumulate (MAC) circuit that mitigates process, supply voltage, and temperature variations. Experimental evaluations on PhysioNet's MIT-BIH and PTB Diagnostics datasets demonstrate the effectiveness of the proposed method, achieving average balanced accuracy of 95% and 94.25% for intra-patient arrhythmia classification and myocardial infarction (MI) classification, respectively. This innovative approach presents a promising avenue for developing low-power arrhythmia classification systems with enhanced accuracy and transferability in biomedical applications.
更多查看译文
关键词
analog neural network,arrhythmia,neural network,classification,intra-patient
AI 理解论文
溯源树
样例
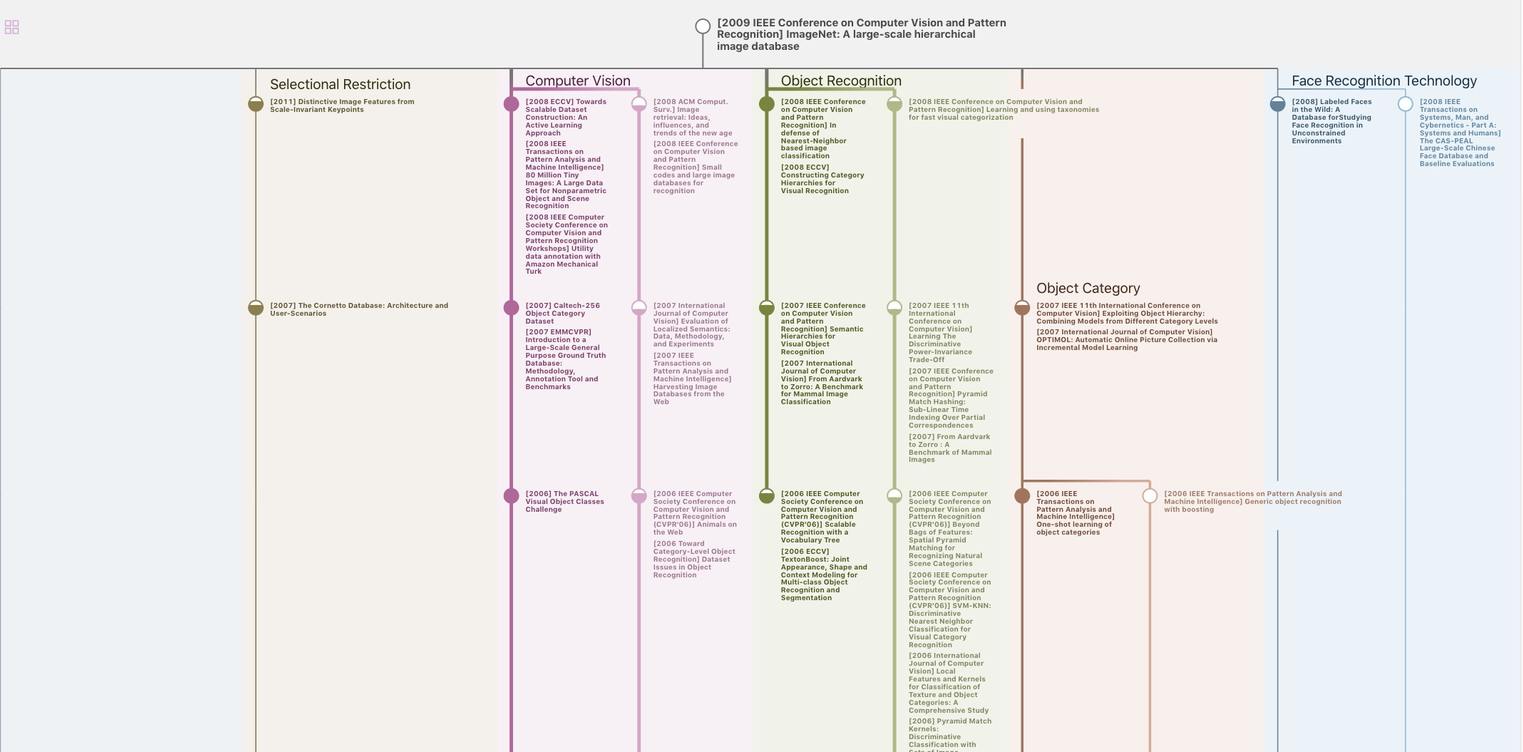
生成溯源树,研究论文发展脉络
Chat Paper
正在生成论文摘要