Generating Optimized Molecules without Patent Infringement
PROCEEDINGS OF THE 32ND ACM INTERNATIONAL CONFERENCE ON INFORMATION AND KNOWLEDGE MANAGEMENT, CIKM 2023(2023)
摘要
Molecular optimization seeks to improve a given molecule's therapeutic profile. It is a key challenge in drug development, but it is difficult due to the constraints of molecular similarity to the original molecule and the size of the chemical space to explore. Numerous works tackled this problem with initial success. Unlike previous works that focus on generating molecules that optimize chemical properties, we focus on the optimization while attempting to "move away" from patented molecules. We present a novel loss function and its utilization in numerous types of molecular optimization algorithms. The loss allows to improve molecular properties while decreasing patent-infringement. We perform empirical evaluation showing superior performance of state-of-the-art models when using the novel loss function. The deployment of the system is underway at the Targeted Drug Delivery and Personalized Medicine labs. It will be utilized to generate targeted carriers of mRNA, providing a new method of drug delivery. The system is also producing non-patented candidates for industrial use, making it a valuable tool in the field of personalized medicine.
更多查看译文
关键词
AI Lead Optimization,Drug Discovery,ML for Healthcare
AI 理解论文
溯源树
样例
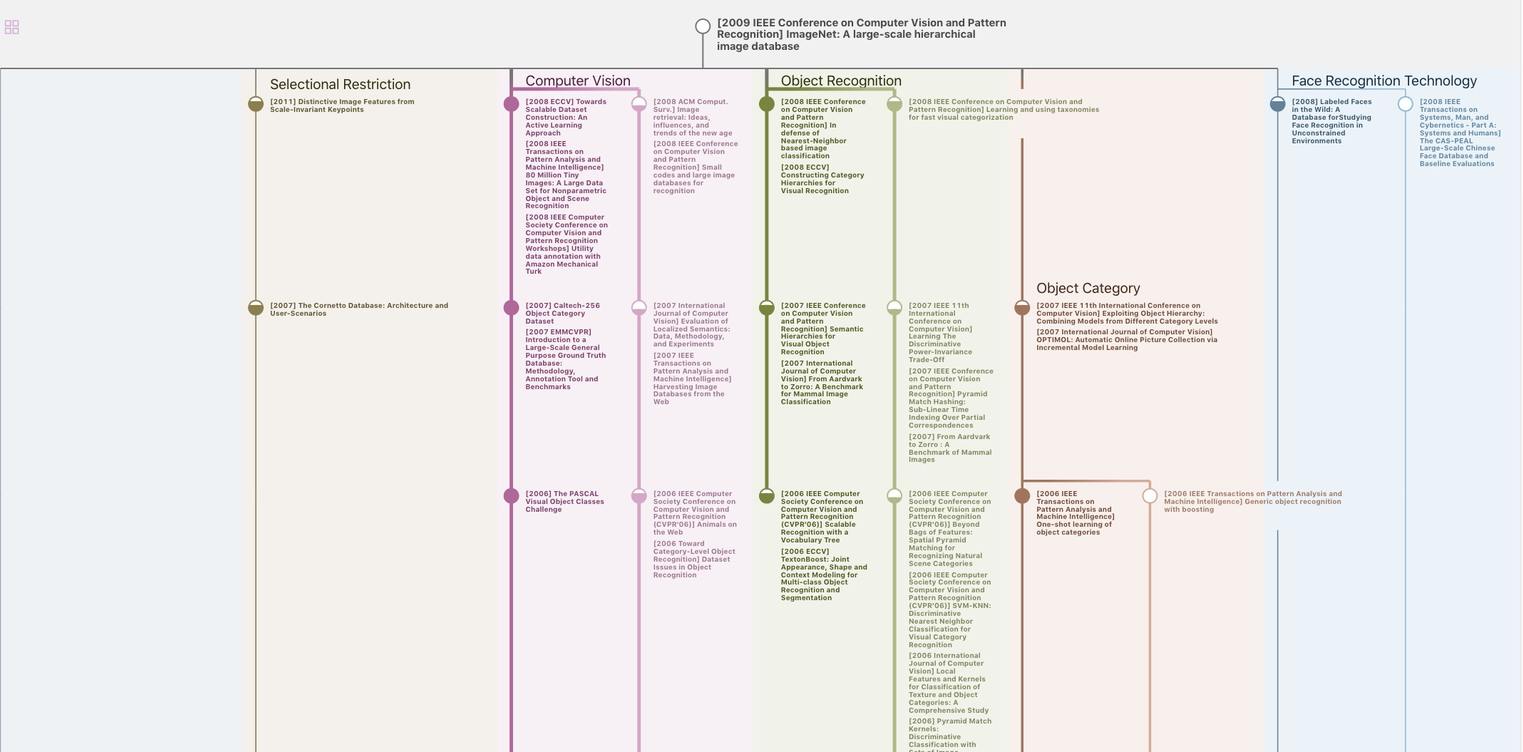
生成溯源树,研究论文发展脉络
Chat Paper
正在生成论文摘要