Non-IID always Bad? Semi-Supervised Heterogeneous Federated Learning with Local Knowledge Enhancement
PROCEEDINGS OF THE 32ND ACM INTERNATIONAL CONFERENCE ON INFORMATION AND KNOWLEDGE MANAGEMENT, CIKM 2023(2023)
摘要
Federated learning (FL) is important for privacy-preserving services by training models without collecting raw user data. Most FL algorithms assume all data is annotated, which is impractical due to the high cost of labeling data in real applications. To alleviate the reliance on labeled data, semi-supervised federated learning (SSFL) has been proposed to utilize unlabeled data on clients to improve model performance. However, most existing methods either have privacy issues which share models trained on other clients, or generate pseudo-labels for unlabeled local datasets with the global model, which is usually biased towards the global data distribution. The latter may lead to sub-optimal accuracy of pseudo-labels, due to the gap between the local data distribution and the global model, especially in non-IID settings. In this paper, we propose a semi-supervised heterogeneous federated learning method with local knowledge enhancement, called FedLoKe, which aims to train an accurate global model from both labeled and unlabeled local data with non-IID distributions. Specifically, in FedLoKe, the server maintains a global model to capture global data distribution, and each client learns a local model to capture local data distribution. Since the distribution captured by the local model is aligned with the local data distribution, we utilize it to generate high-accuracy pseudo-labels of the unlabeled dataset for global model training. To prevent the local model from severely overfitting local labeled data, we further use the exponential moving average and apply the global model to generate pseudo-labels for local modeling training. Experiments on four datasets show the effectiveness of FedLoKe. Our code is available at: https://github.com/zcfinal/FedLoKe.
更多查看译文
关键词
Federated Learning,Semi-Supervised,Heterogeneity,Pseudo-Labeling
AI 理解论文
溯源树
样例
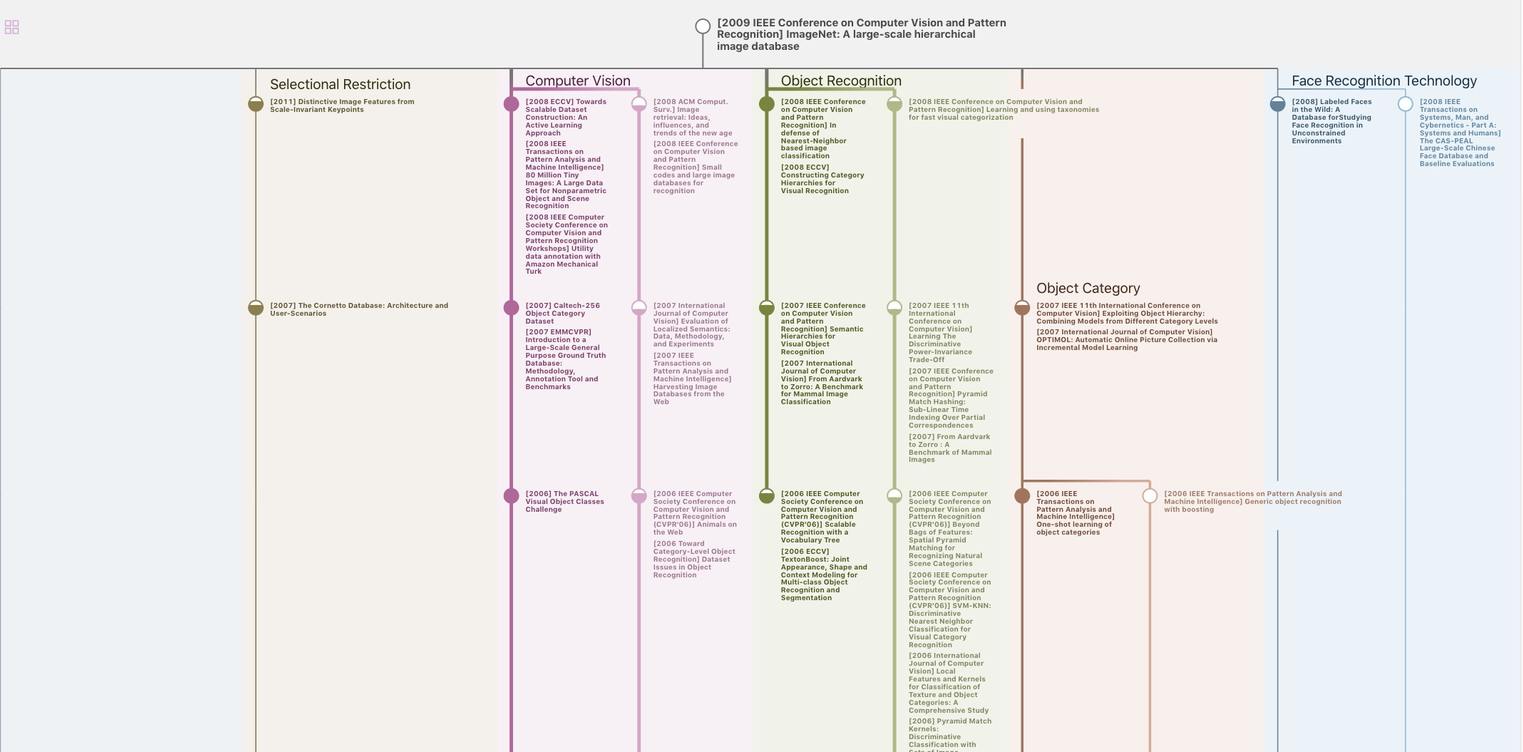
生成溯源树,研究论文发展脉络
Chat Paper
正在生成论文摘要