AttentionVote: A coarse-to-fine voting network of anchor-free 6D pose estimation on point cloud for robotic bin-picking application
ROBOTICS AND COMPUTER-INTEGRATED MANUFACTURING(2024)
摘要
Current state-of-the-art pose estimation methods are almost launched on segmented RGB-D images. However, these methods may not apply to more general industrial parts due to a lack of texture information and highocclusion of stacked objects. This article establishes an end-to-end pipeline to synchronously regress all potential object poses from an unsegmented point cloud. The point pair features (PPFs) are first extracted and then fed into a PointNet-like backbone for obtaining the point-wise features. Based on the center voting, a coarse-to-fine voting architecture is proposed to extract instance features instead of implementing instance segmentation. A lightweight three-dimensional (3D) heatmap is leveraged to cluster votes and generate center seeds. Further, an attention voting module is constructed to fuse point-wise features into instance-wise features adaptively. Ultimately, the suggested network regresses object poses with a quaternion loss to handle the symmetric puzzle. The network holds the advantage of producing the final pose prediction without any post-processing steps like nonmaximum suppression (NMS) or any pose refinement modules like iterative closest point (ICP). The proposed network is evaluated on the public Fraunhofer IPA dataset, which demonstrates the robustness of the pose estimation network with much better performance. Meanwhile, the network is further validated on our synthetic and real-world datasets of industrial parts for robotic bin-picking.
更多查看译文
关键词
Pose estimation,Voting network,Point cloud,Industrial parts,Robotic bin-picking
AI 理解论文
溯源树
样例
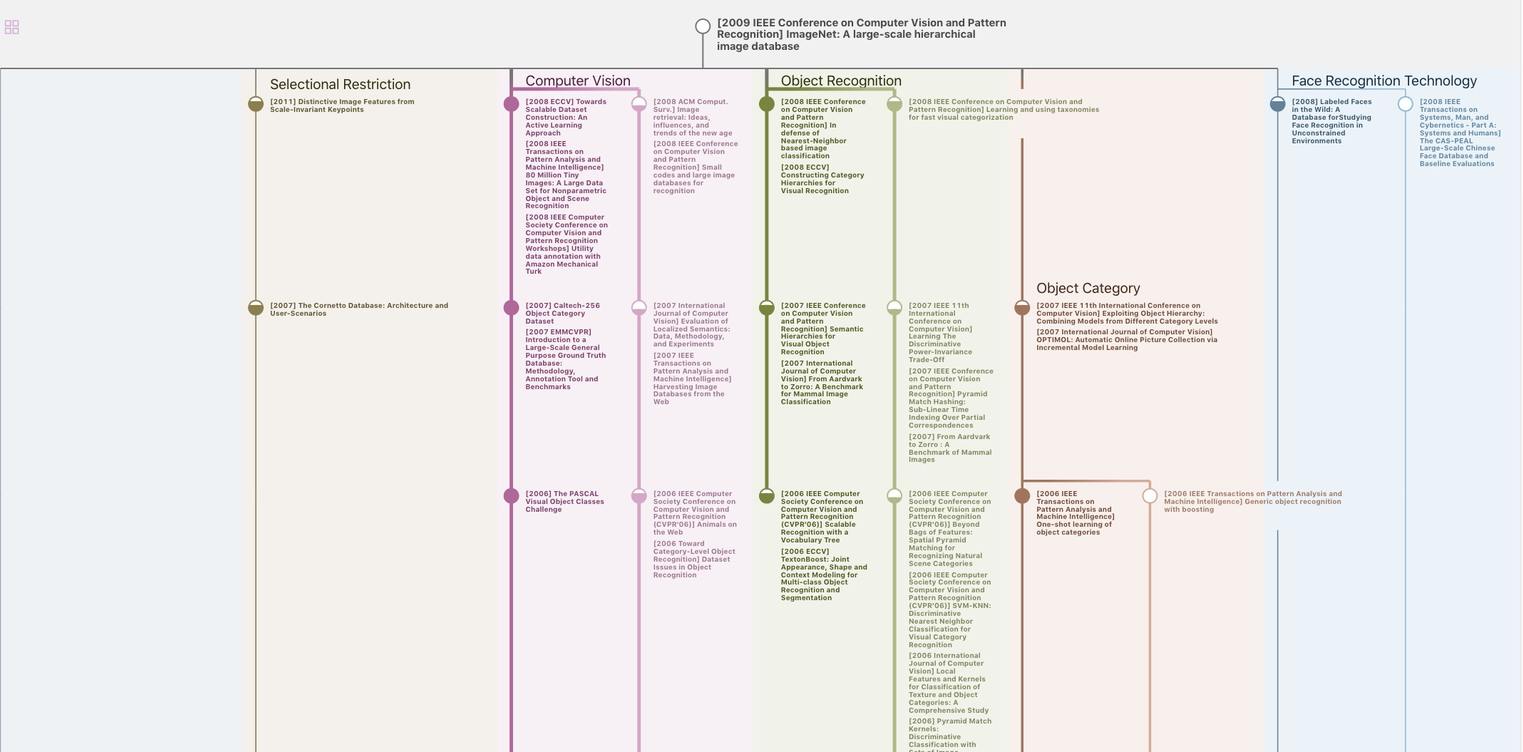
生成溯源树,研究论文发展脉络
Chat Paper
正在生成论文摘要