Dual-Branch Fourier-Mixing Transformer Network for Hyperspectral Target Detection
Remote Sensing(2023)
摘要
In recent years, transformers have shown great potential in hyperspectral image processing and have also been gradually applied in hyperspectral target detection (HTD). Nonetheless, applying a typical transformer to HTD remains challenging. The heavy computation burden of the multi-head self-attention (MSA) in transformers limits its efficient HTD, while the limited ability to extract local spectral features can reduce the discrimination of the learned spectral features. To further explore the potential of transformers for HTD, for balance of representation ability and computational efficiency, we propose a dual-branch Fourier-mixing transformer network for hyperspectral target detection (DBFTTD). First, this work explores a dual-branch Fourier-mixing transformer network. The transformer-style network replaces the MSA sublayer in the transformer with a Fourier-mixing sublayer, which shows advantages in improving computational efficiency and learning valuable spectral information effectively for HTD. Second, this work proposes learnable filter ensembles in the Fourier domain that are inspired by ensemble learning to improve detection performance. Third, a simple but efficient dropout strategy is proposed for data augmentation. Sufficient and balanced training samples are constructed for training the dual-branch network, and training samples for balanced learning can further improve detection performance. Experiments on four data sets indicate that our proposed detector is superior to the state-of-the-art detectors.
更多查看译文
关键词
dual-branch network, Fourier-mixing transformer, ensemble method, hyperspectral target detection
AI 理解论文
溯源树
样例
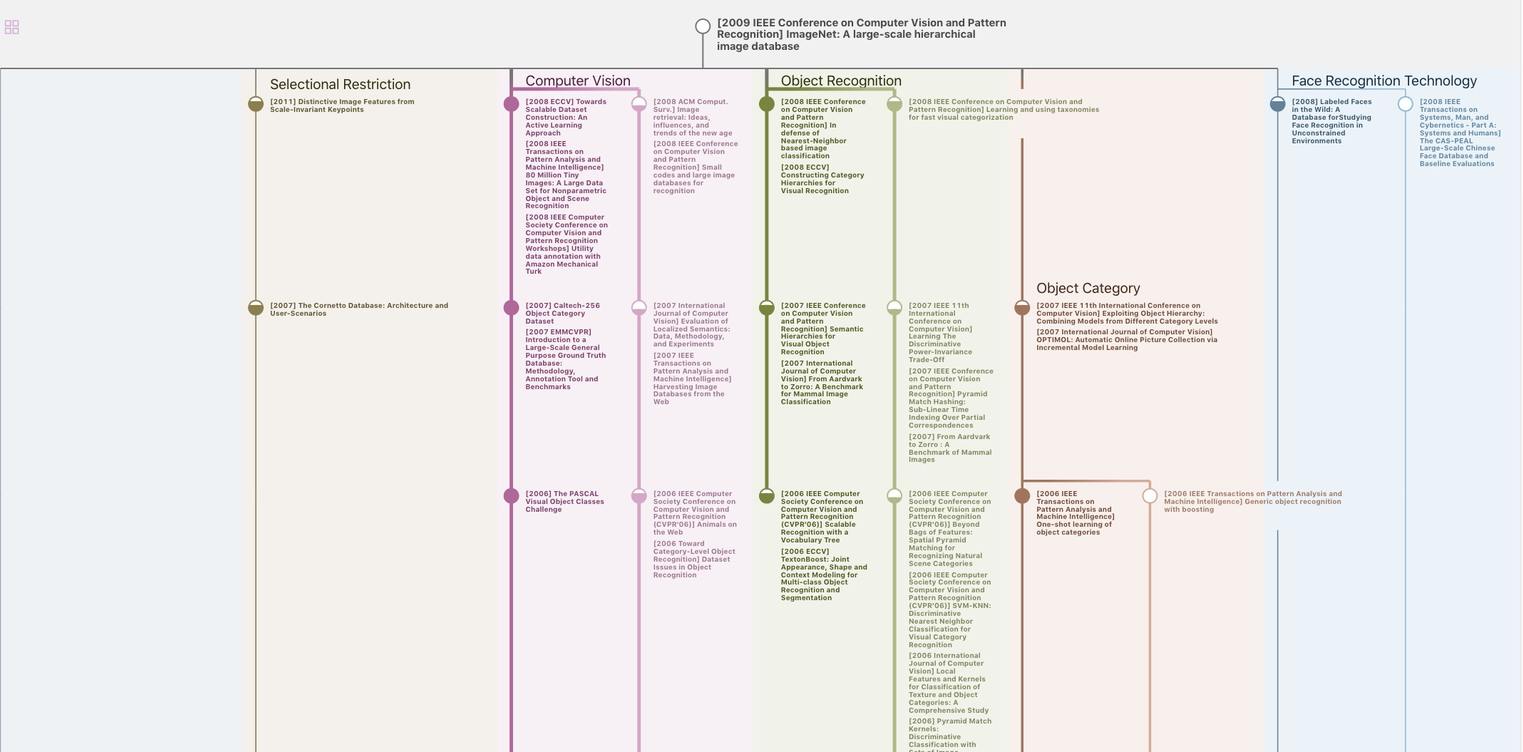
生成溯源树,研究论文发展脉络
Chat Paper
正在生成论文摘要