GAIT: Generating Aesthetic Indoor Tours with Deep Reinforcement Learning.
Proceedings of the IEEE/CVF International Conference on Computer Vision (ICCV)(2023)
摘要
Placing and orienting a camera to compose aesthetically meaningful shots of a scene is not only a key objective in real-world photography and cinematography but also for virtual content creation. The framing of a camera often significantly contributes to the story telling in movies, games, and mixed reality applications. Generating single camera poses or even contiguous trajectories either requires a significant amount of manual labor or requires solving high-dimensional optimization problems, which can be computationally demanding and error-prone. In this paper, we introduce GAIT, a framework for training a Deep Reinforcement Learning (DRL) agent, that learns to automatically control a camera to generate a sequence of aesthetically meaningful views for synthetic 3D indoor scenes. To generate sequences of frames with high aesthetic value, GAIT relies on a neural aesthetics estimator, which is trained on a crowed-sourced dataset. Additionally, we introduce regularization techniques for diversity and smoothness to generate visually interesting trajectories for a 3D environment, and to constrain agent acceleration in the reward function to generate a smooth sequence of camera frames. We validated our method by comparing it to baseline algorithms, based on a perceptual user study, and through ablation studies. Code and visual results are available on the project website: https://desaixie.github.io/gait-rl
更多查看译文
AI 理解论文
溯源树
样例
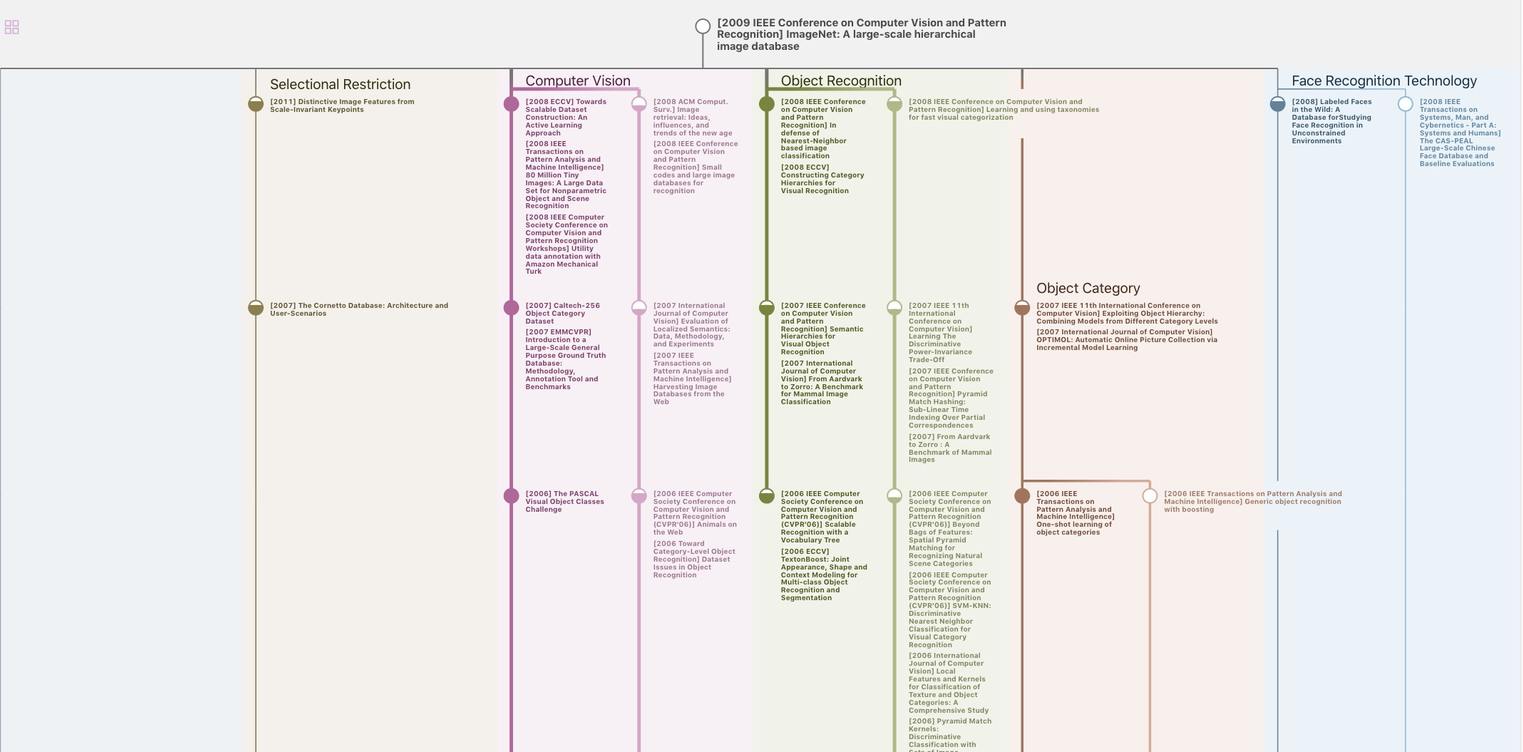
生成溯源树,研究论文发展脉络
Chat Paper
正在生成论文摘要