Pixel State Value Network for Combined Prediction and Planning in Interactive Environments
CoRR(2023)
摘要
Automated vehicles operating in urban environments have to reliably interact with other traffic participants. Planning algorithms often utilize separate prediction modules forecasting probabilistic, multi-modal, and interactive behaviors of objects. Designing prediction and planning as two separate modules introduces significant challenges, particularly due to the interdependence of these modules. This work proposes a deep learning methodology to combine prediction and planning. A conditional GAN with the U-Net architecture is trained to predict two high-resolution image sequences. The sequences represent explicit motion predictions, mainly used to train context understanding, and pixel state values suitable for planning encoding kinematic reachability, object dynamics, safety, and driving comfort. The model can be trained offline on target images rendered by a sampling-based model-predictive planner, leveraging real-world driving data. Our results demonstrate intuitive behavior in complex situations, such as lane changes amidst conflicting objectives.
更多查看译文
关键词
planning,combined prediction,environments
AI 理解论文
溯源树
样例
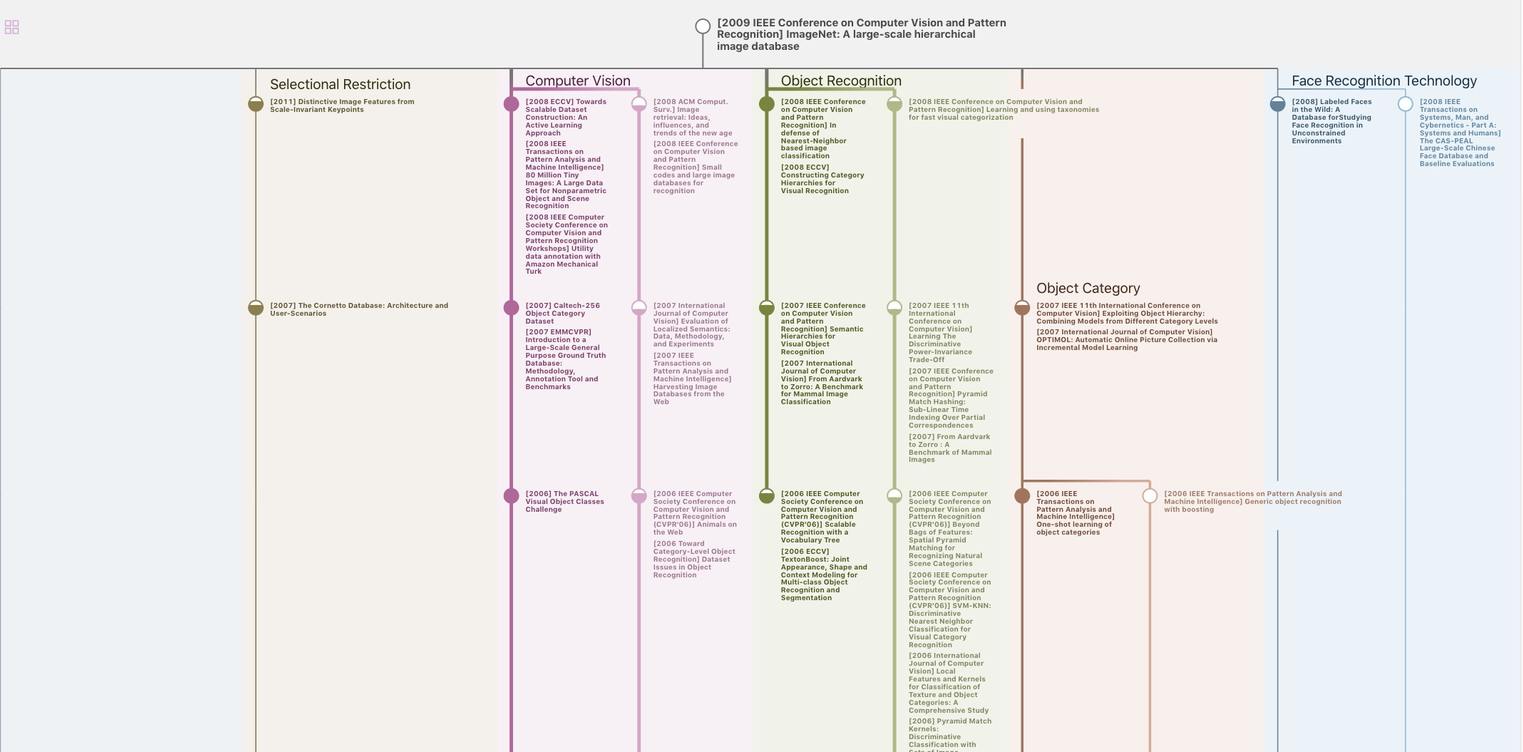
生成溯源树,研究论文发展脉络
Chat Paper
正在生成论文摘要