Going Beyond Neural Network Feature Similarity: The Network Feature Complexity and Its Interpretation Using Category Theory
ICLR 2024(2023)
摘要
The behavior of neural networks still remains opaque, and a recently widely noted phenomenon is that networks often achieve similar performance when initialized with different random parameters. This phenomenon has attracted significant attention in measuring the similarity between features learned by distinct networks. However, feature similarity could be vague in describing the same feature since equivalent features hardly exist. In this paper, we expand the concept of equivalent feature and provide the definition of what we call functionally equivalent features. These features produce equivalent output under certain transformations. Using this definition, we aim to derive a more intrinsic metric for the so-called feature complexity regarding the redundancy of features learned by a neural network at each layer. We offer a formal interpretation of our approach through the lens of category theory, a well-developed area in mathematics. To quantify the feature complexity, we further propose an efficient algorithm named Iterative Feature Merging. Our experimental results validate our ideas and theories from various perspectives. We empirically demonstrate that the functionally equivalence widely exists among different features learned by the same neural network and we could reduce the number of parameters of the network without affecting the performance.The IFM shows great potential as a data-agnostic model prune method. We have also drawn several interesting empirical findings regarding the defined feature complexity.
更多查看译文
关键词
Category Theory,Neural Networks Feature Complexity
AI 理解论文
溯源树
样例
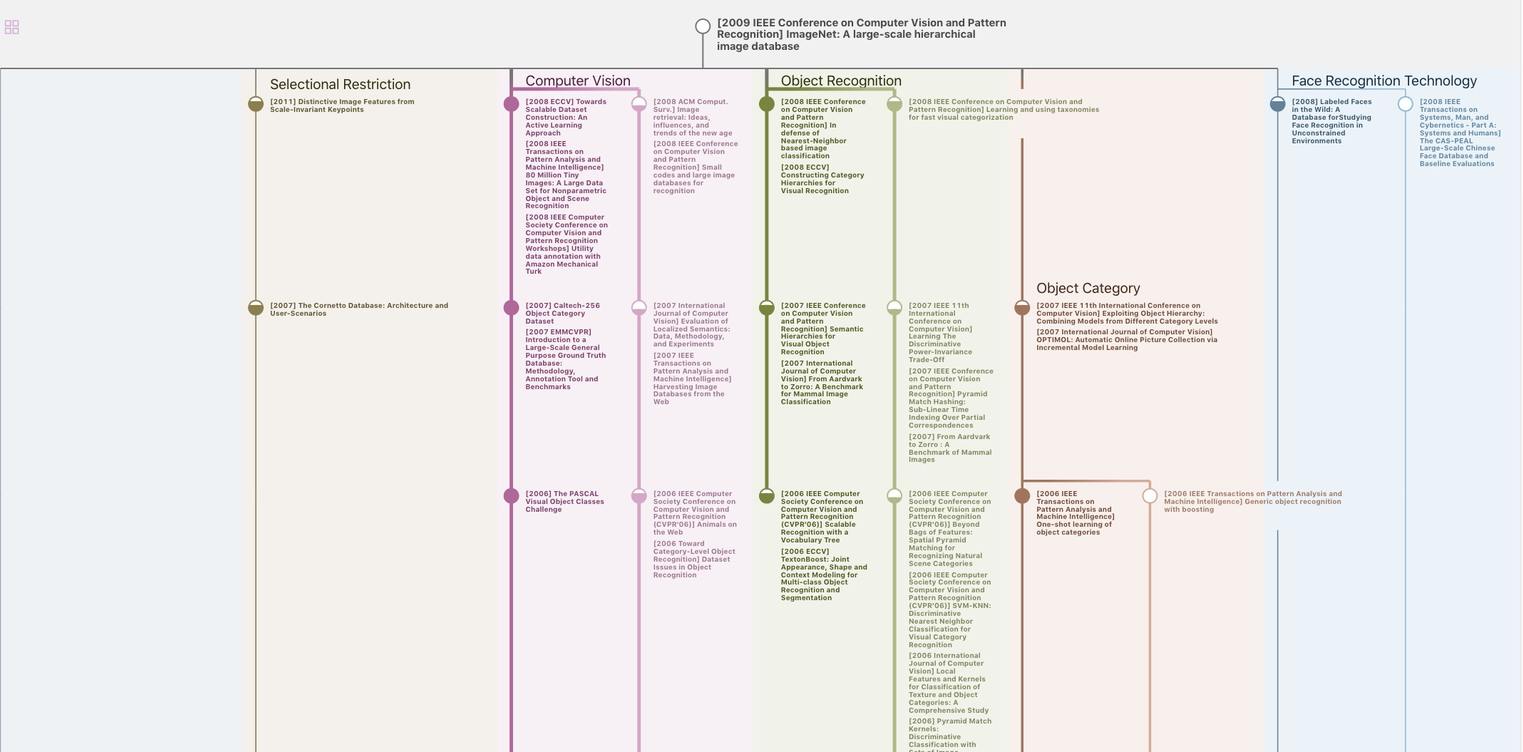
生成溯源树,研究论文发展脉络
Chat Paper
正在生成论文摘要