FEIR: Quantifying and Reducing Envy and Inferiority for Fair Recommendation of Limited Resources.
HR@RecSys(2023)
摘要
Recommendation in settings such as e-recruitment and online dating involves distributing limited opportunities, which differs from recommending practically unlimited goods such as in e-commerce or music recommendation. This setting calls for novel approaches to quantify and enforce fairness. Indeed, typical recommender systems recommend each user their top relevant items, such that desirable items may be recommended simultaneously to more and to less qualified individuals. This is arguably unfair to the latter. Indeed, when they pursue such a desirable recommendation (e.g. by applying for a job), they are unlikely to be successful. To quantify fairness in such settings, we introduce inferiority : a novel (un)fairness measure that quantifies the competitive disadvantage of a user for their recommended items. Inferiority is complementary to envy : a previously-proposed fairness notion that quantifies the extent to which a user prefers other users’ recommendations over their own. We propose to use both inferiority and envy in combination with an accuracy-related measure called utility : the aggregated relevancy scores of the recommended items. Unfortunately, none of these three measures are differentiable, making it hard to optimize them, and restricting their immediate use to evaluation only. To remedy this, we reformulate them in the context of a probabilistic interpretation of recommender systems, resulting in differentiable versions. We show how these loss functions can be combined in a multi-objective optimization problem that we call FEIR (Fairness through Envy and Inferiority Reduction), used as a post-processing of the scores from any standard recommender system. Experiments on synthetic and real-world data show that the proposed approach effectively improves the trade-offs between inferiority, envy and utility, compared to the naive recommendation and the state of the art method for the related problem of congestion alleviation in job recommendation. We discuss and enhance the practical impact of our findings on a wide range of real-world recommendation scenarios, and we offer implementations of visualization tools to render the envy and inferiority metrics more accessible.
更多查看译文
关键词
envy,inferiority,recommendation,resources,fair
AI 理解论文
溯源树
样例
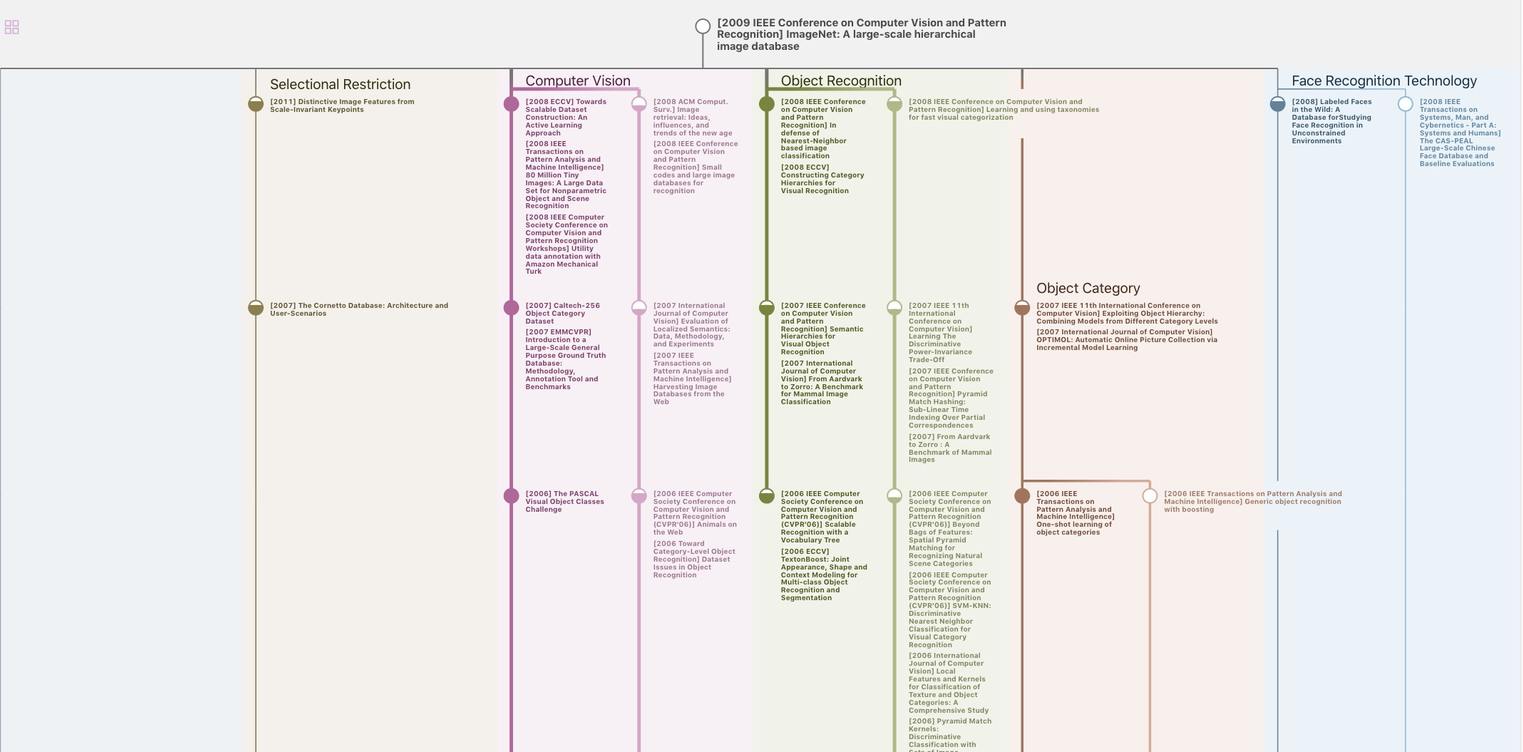
生成溯源树,研究论文发展脉络
Chat Paper
正在生成论文摘要