Deep learning strategy for small dataset from atomic force microscopy mechano-imaging on macrophages phenotypes
Frontiers in Bioengineering and Biotechnology(2023)
摘要
The cytoskeleton is involved during movement, shaping, resilience, and functionality in immune system cells. Biomarkers such as elasticity and adhesion can be promising alternatives to detect the status of cells upon phenotype activation in correlation with functionality. For instance, professional immune cells such as macrophages undergo phenotype functional polarization, and their biomechanical behaviors can be used as indicators for early diagnostics. For this purpose, combining the biomechanical sensitivity of atomic force microscopy (AFM) with the automation and performance of a deep neural network (DNN) is a promising strategy to distinguish and classify different activation states. To resolve the issue of small datasets in AFM-typical experiments, nanomechanical maps were divided into pixels with additional localization data. On such an enlarged dataset, a DNN was trained by multimodal fusion, and the prediction was obtained by voting classification. Without using conventional biomarkers, our algorithm demonstrated high performance in predicting the phenotype of macrophages. Moreover, permutation feature importance was employed to interpret the results and unveil the importance of different biophysical properties and, in turn, correlated this with the local density of the cytoskeleton. While our results were demonstrated on the RAW264.7 model cell line, we expect that our methodology could be opportunely customized and applied to distinguish different cell systems and correlate feature importance with biophysical properties to unveil innovative markers for diagnostics.
更多查看译文
关键词
deep learning, small dataset, atomic force microscopy, mechano-imaging, macrophages
AI 理解论文
溯源树
样例
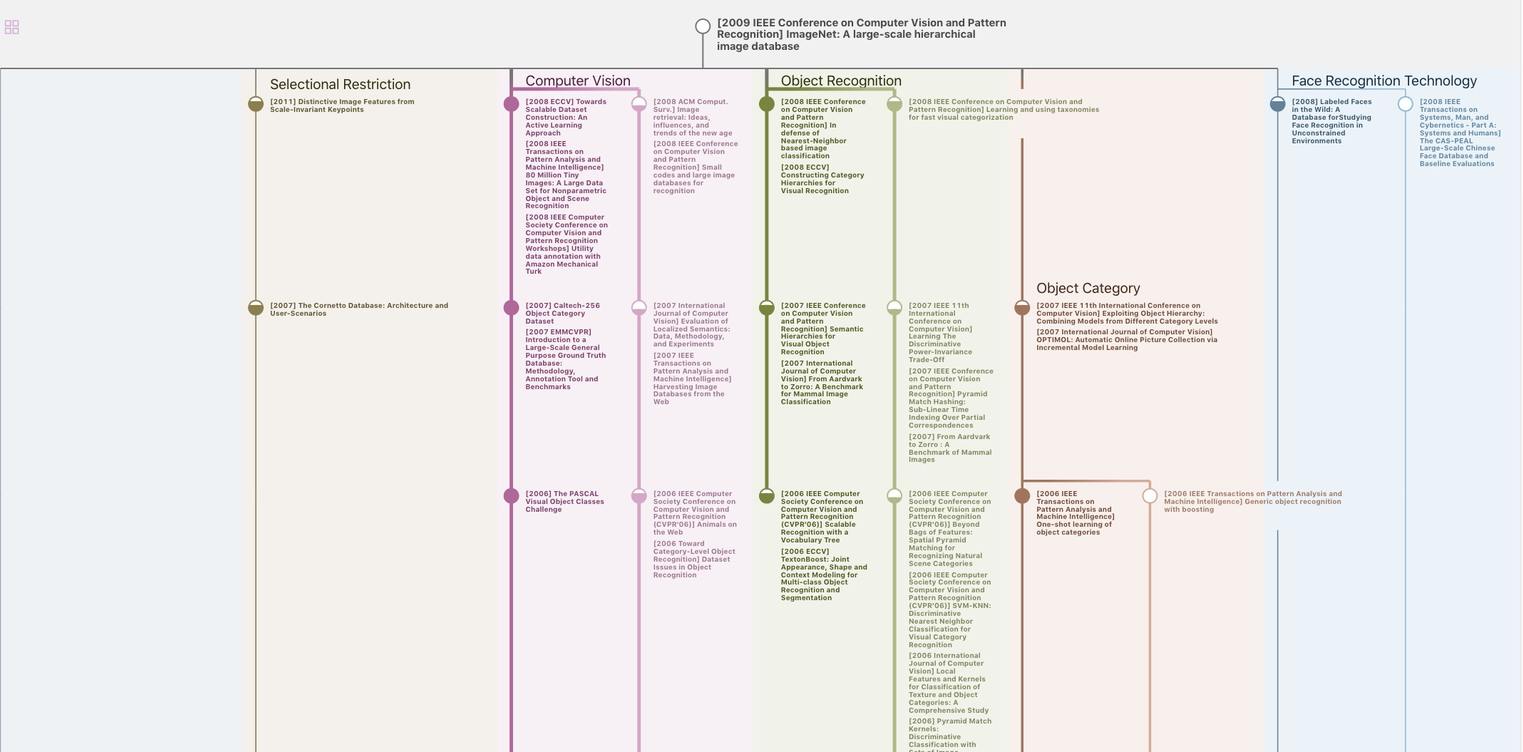
生成溯源树,研究论文发展脉络
Chat Paper
正在生成论文摘要