Large Language Models as Analogical Reasoners
ICLR 2024(2023)
摘要
Chain-of-thought (CoT) prompting for language models demonstrates impressive performance across reasoning tasks, but typically needs labeled exemplars of the reasoning process. In this work, we introduce a new prompting approach, Analogical Prompting, designed to automatically guide the reasoning process of large language models. Inspired by analogical reasoning, a cognitive process in which humans draw from relevant past experiences to tackle new problems, our approach prompts language models to self-generate relevant exemplars or knowledge in the context, before proceeding to solve the given problem. This method presents several advantages: it obviates the need for labeling or retrieving exemplars, offering generality and convenience; it can also tailor the generated exemplars and knowledge to each problem, offering adaptability. Experimental results show that our approach outperforms 0-shot CoT and manual few-shot CoT in a variety of reasoning tasks, including math problem solving in GSM8K and MATH, code generation in Codeforces, and other reasoning tasks in BIG-Bench.
更多查看译文
关键词
large language model,prompting,analogical reasoning
AI 理解论文
溯源树
样例
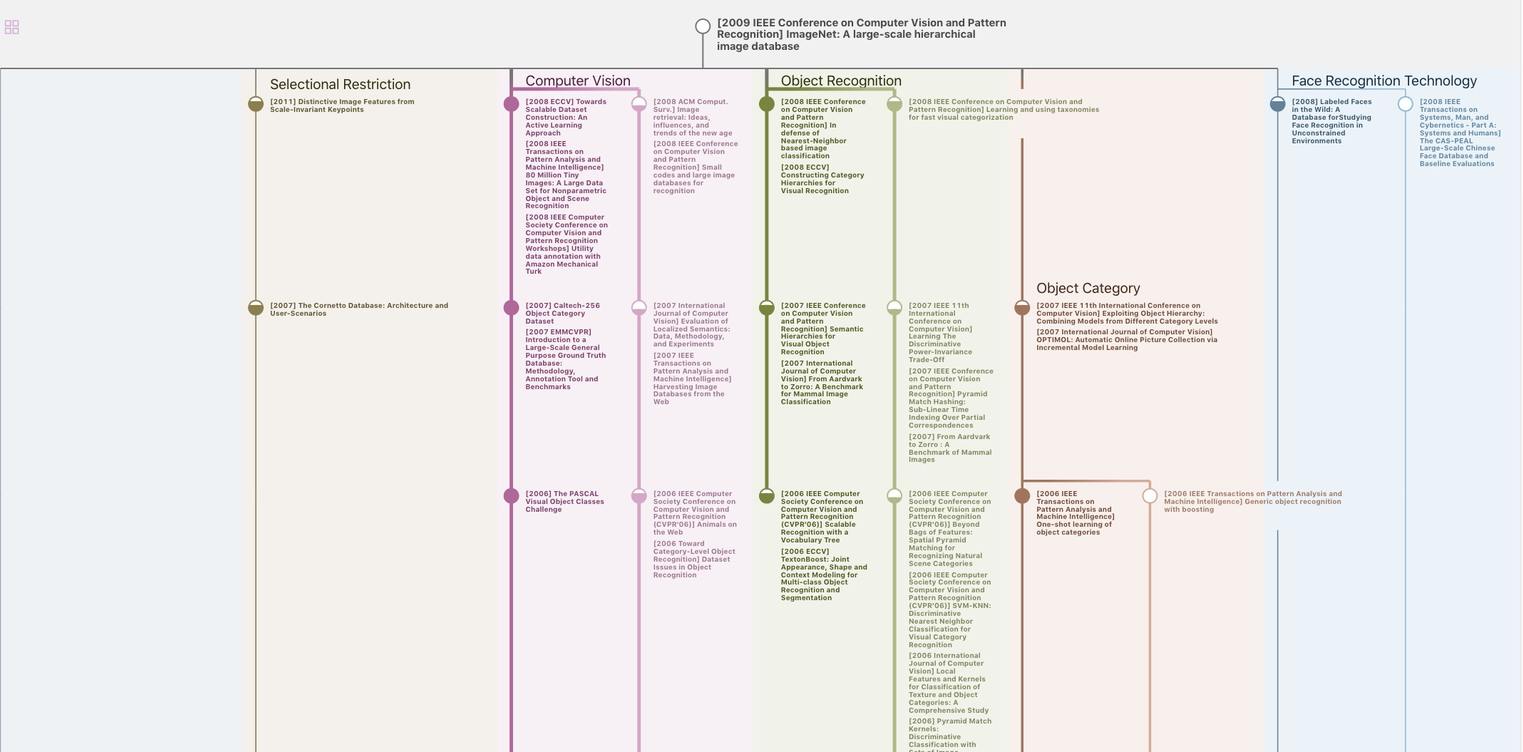
生成溯源树,研究论文发展脉络
Chat Paper
正在生成论文摘要