Radar Instance Transformer: Reliable Moving Instance Segmentation in Sparse Radar Point Clouds
IEEE TRANSACTIONS ON ROBOTICS(2024)
摘要
The perception of moving objects is crucial for autonomous robots performing collision avoidance in dynamic environments. LiDARs and cameras tremendously enhance scene interpretation but do not provide direct motion information and face limitations under adverse weather. Radar sensors overcome these limitations and provide Doppler velocities, delivering direct information on dynamic objects. In this article, we address the problem of moving instance segmentation in radar point clouds to enhance scene interpretation for safety-critical tasks. Our radar instance transformer enriches the current radar scan with temporal information without passing aggregated scans through a neural network. We propose a full-resolution backbone to prevent information loss in sparse point cloud processing. Our instance transformer head incorporates essential information to enhance segmentation but also enables reliable, class-agnostic instance assignments. In sum, our approach shows superior performance on the new moving instance segmentation benchmarks, including diverse environments, and provides model-agnostic modules to enhance scene interpretation.
更多查看译文
关键词
Radar,Point cloud compression,Feature extraction,Transformers,Task analysis,Encoding,Sensors,Deep learning in robotics and automation,object detection,radar perception,segmentation and categorization,semantic scene understanding
AI 理解论文
溯源树
样例
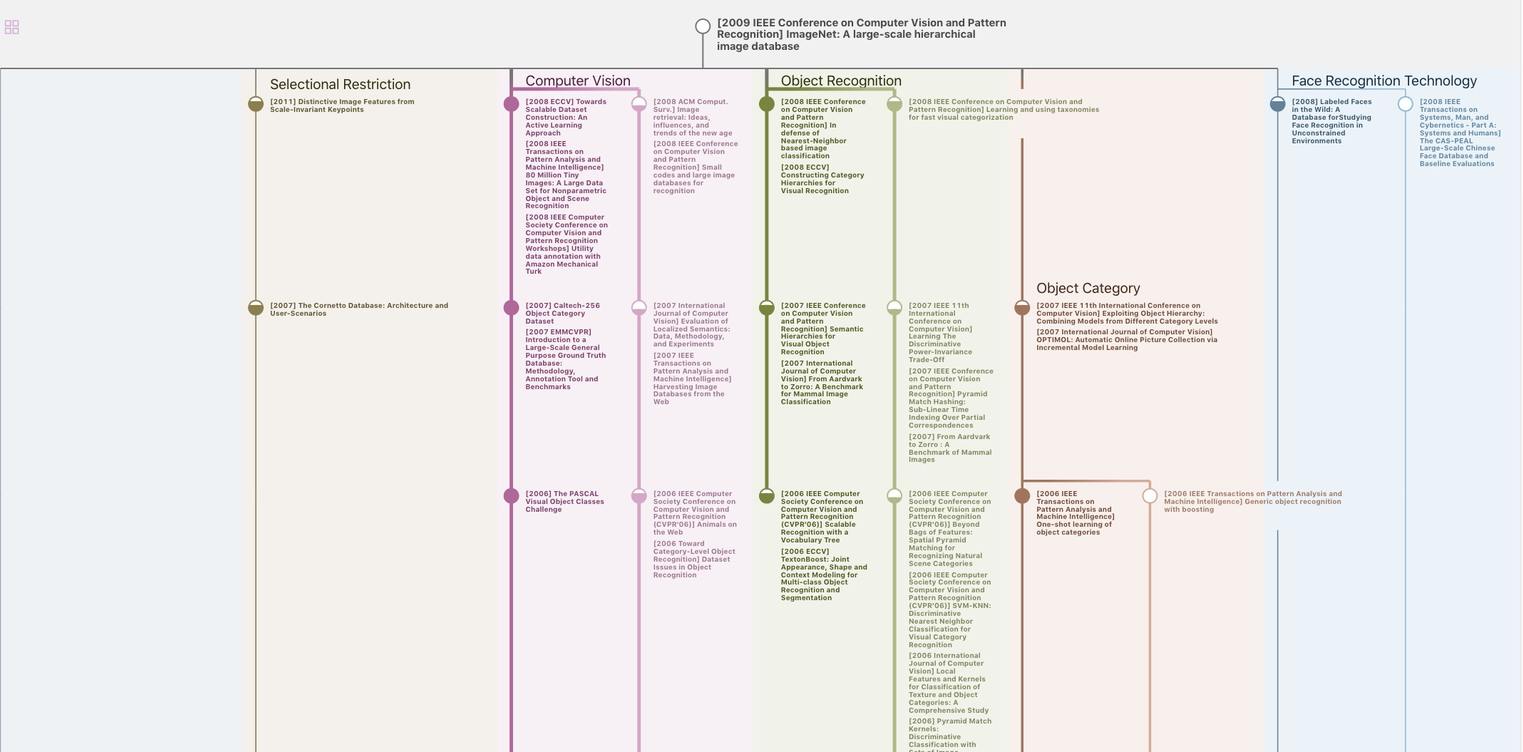
生成溯源树,研究论文发展脉络
Chat Paper
正在生成论文摘要