SPOT: Scalable 3D Pre-training via Occupancy Prediction for Autonomous Driving
CoRR(2023)
摘要
Annotating 3D LiDAR point clouds for perception tasks including 3D object detection and LiDAR semantic segmentation is notoriously time-and-energy-consuming. To alleviate the burden from labeling, it is promising to perform large-scale pre-training and fine-tune the pre-trained backbone on different downstream datasets as well as tasks. In this paper, we propose SPOT, namely Scalable Pre-training via Occupancy prediction for learning Transferable 3D representations, and demonstrate its effectiveness on various public datasets with different downstream tasks under the label-efficiency setting. Our contributions are threefold: (1) Occupancy prediction is shown to be promising for learning general representations, which is demonstrated by extensive experiments on plenty of datasets and tasks. (2) SPOT uses beam re-sampling technique for point cloud augmentation and applies class-balancing strategies to overcome the domain gap brought by various LiDAR sensors and annotation strategies in different datasets. (3) Scalable pre-training is observed, that is, the downstream performance across all the experiments gets better with more pre-training data. We believe that our findings can facilitate understanding of LiDAR point clouds and pave the way for future exploration in LiDAR pre-training. Codes and models will be released.
更多查看译文
关键词
occupancy prediction,3d,autonomous,driving,pre-training
AI 理解论文
溯源树
样例
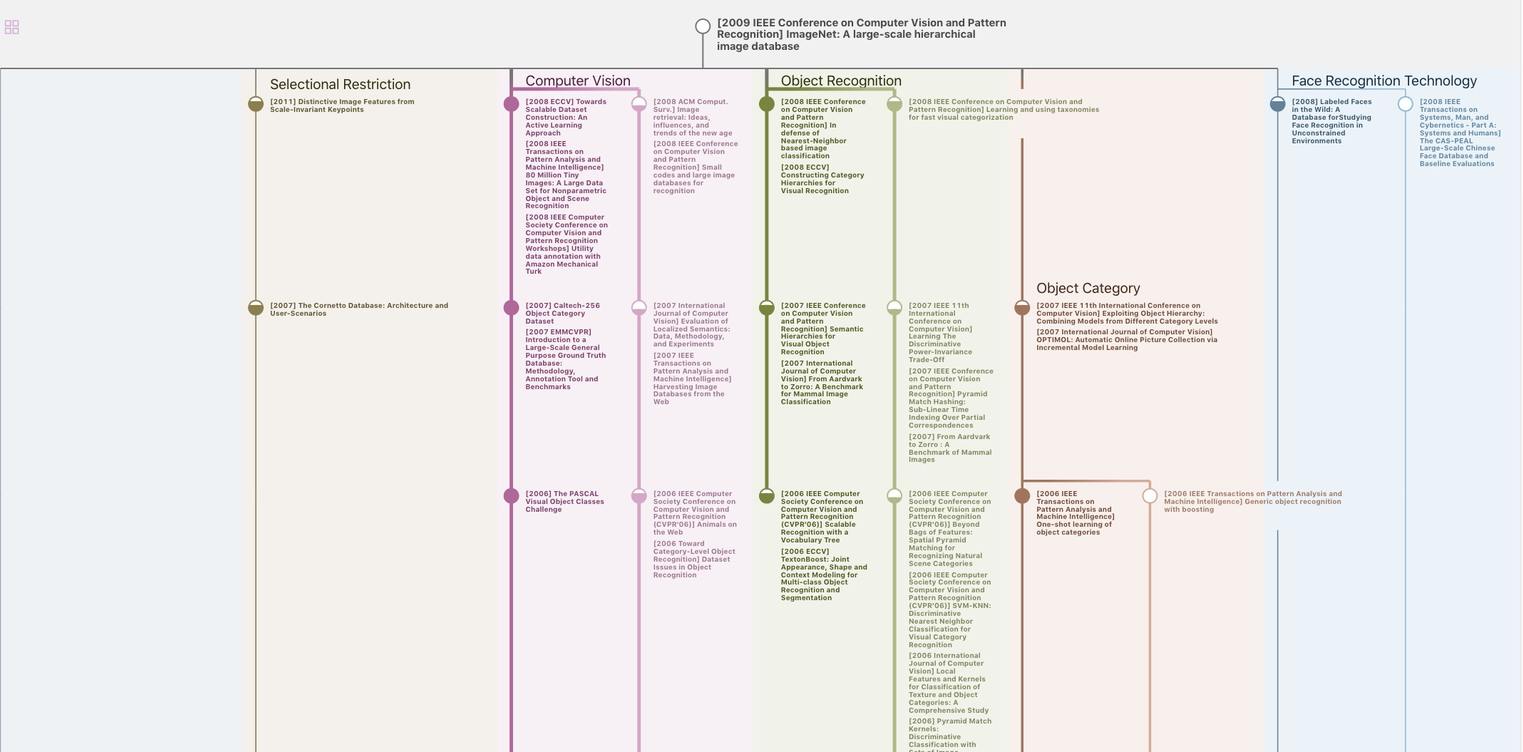
生成溯源树,研究论文发展脉络
Chat Paper
正在生成论文摘要