Leveraging World Model Disentanglement in Value-Based Multi-Agent Reinforcement Learning
CoRR(2023)
摘要
In this paper, we propose a novel model-based multi-agent reinforcement learning approach named Value Decomposition Framework with Disentangled World Model to address the challenge of achieving a common goal of multiple agents interacting in the same environment with reduced sample complexity. Due to scalability and non-stationarity problems posed by multi-agent systems, model-free methods rely on a considerable number of samples for training. In contrast, we use a modularized world model, composed of action-conditioned, action-free, and static branches, to unravel the environment dynamics and produce imagined outcomes based on past experience, without sampling directly from the real environment. We employ variational auto-encoders and variational graph auto-encoders to learn the latent representations for the world model, which is merged with a value-based framework to predict the joint action-value function and optimize the overall training objective. We present experimental results in Easy, Hard, and Super-Hard StarCraft II micro-management challenges to demonstrate that our method achieves high sample efficiency and exhibits superior performance in defeating the enemy armies compared to other baselines.
更多查看译文
关键词
world model disentanglement,learning,value-based,multi-agent
AI 理解论文
溯源树
样例
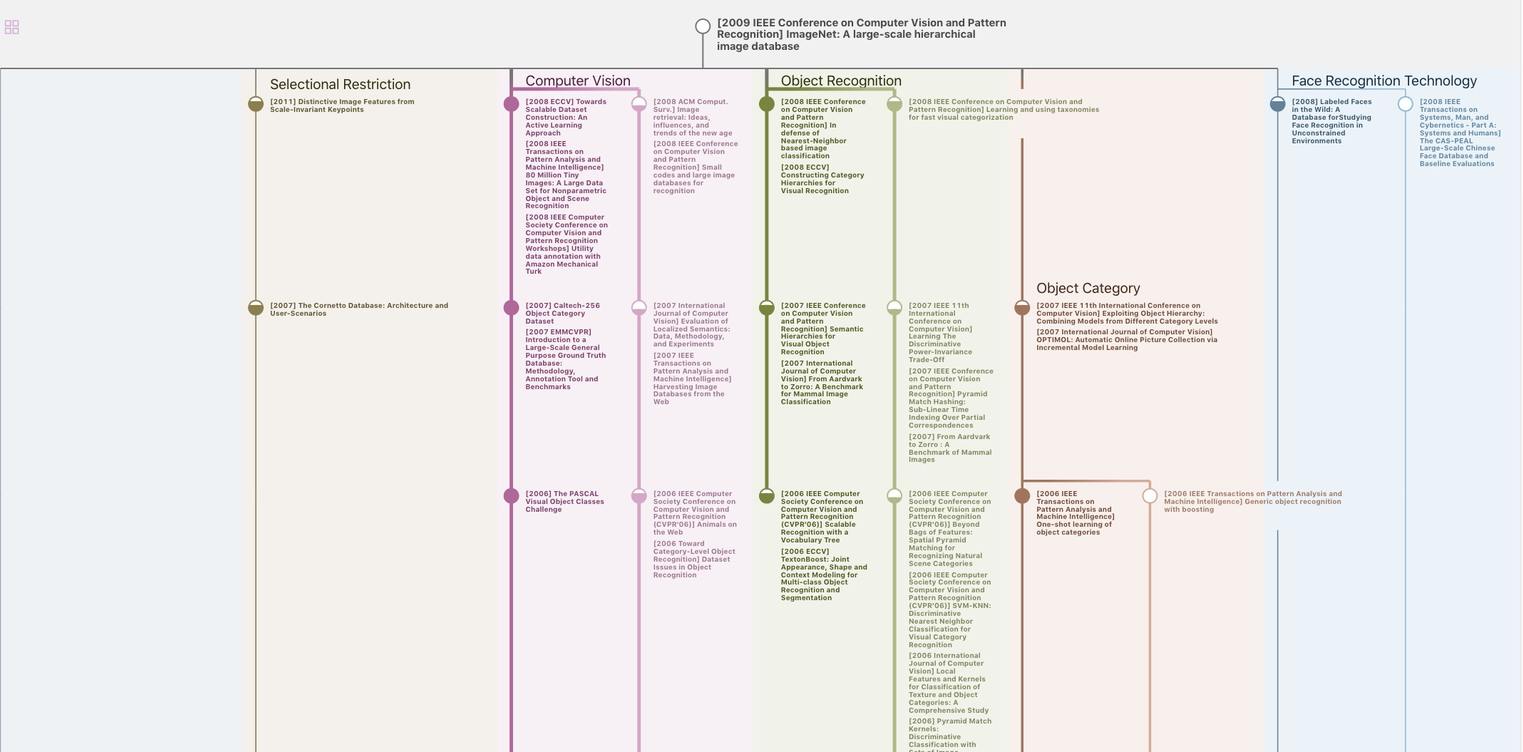
生成溯源树,研究论文发展脉络
Chat Paper
正在生成论文摘要