Optimization of EMG-Derived Features for Upper Limb Prosthetic Control.
Living Machines (1)(2023)
摘要
Polyarticulated active prostheses constitute a promising solution for upper limb amputees. The bottleneck for their adoption though, is the lack of intuitive control. In this context, machine learning algorithms based on pattern recognition from electromyographic (EMG) signals represent a great opportunity for naturally operating prosthetic devices, but their performance is strongly affected by the selection of input features. In this study, we investigated different combinations of 13 EMG-derived features obtained from EMG signals of healthy individuals performing upper limb movements and tested their performance for movement classification using an Artificial Neural Network. We found that input data (i.e., the set of input features) can be reduced by more than 50% without any loss in accuracy, while diminishing the computing time required to train the classifier. Our results indicate that input features must be properly selected in order to optimize prosthetic control.
更多查看译文
关键词
emg-derived
AI 理解论文
溯源树
样例
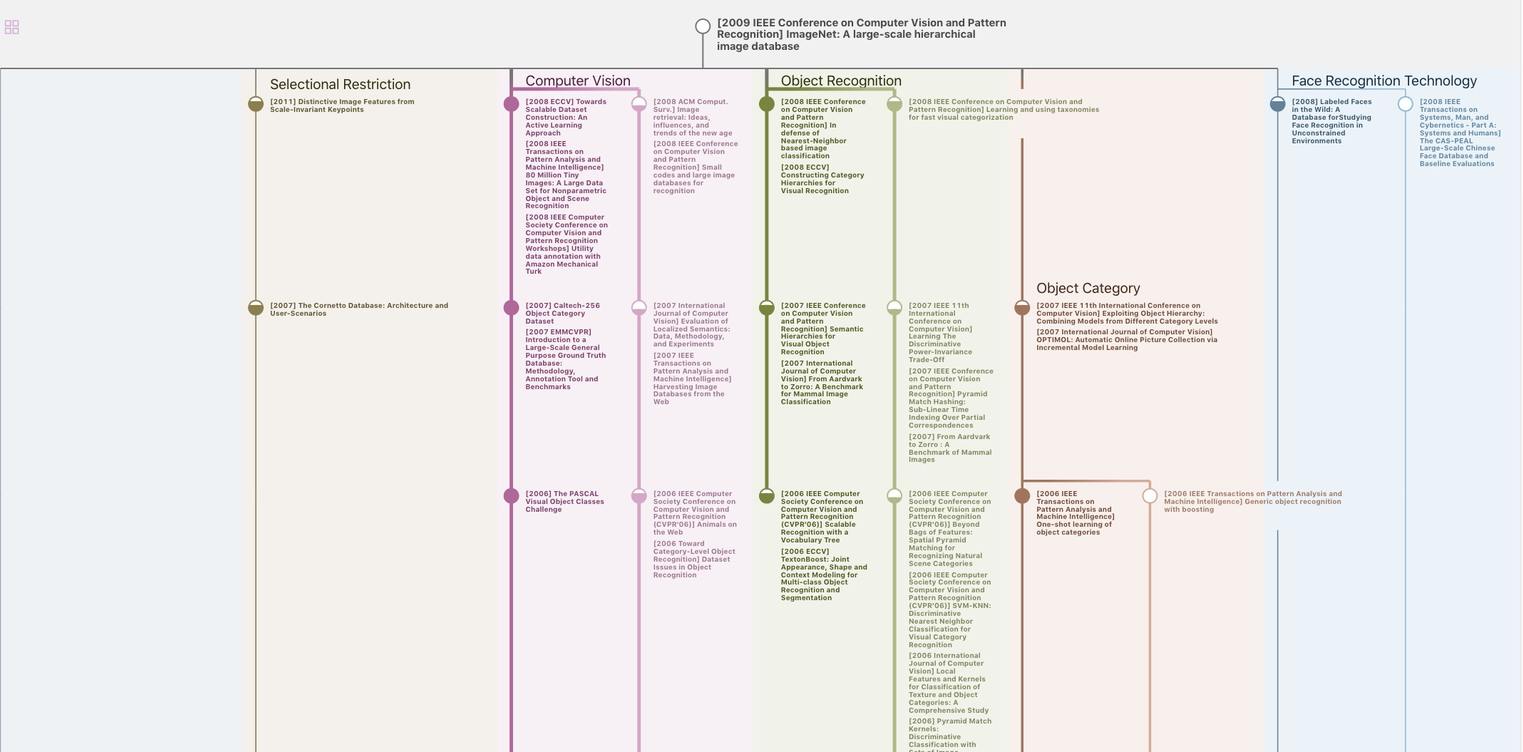
生成溯源树,研究论文发展脉络
Chat Paper
正在生成论文摘要