A Unified Framework of Deep Neural Networks and Gappy Proper Orthogonal Decomposition for Global Field Reconstruction.
IJCNN(2023)
摘要
Full-state estimation with a limited number of sensors is a valuable and challenging task in monitoring and controlling complex physical systems. Supervised learning methods based on deep neural networks have shown excellent performance by learning the nonlinear mapping from sparse observations to global field. However, The neural network is a black box with weak explanation for physical processes, and the reconstruction performance is limited to the architecture and optimization of neural network. This paper aims to leverage the structure and laws inherent in data to reconstruct the global field by solving optimization problems instead of single network learning. We propose a unified global field reconstruction framework consisting of neural network prediction, proper orthogonal decomposition (POD), and linear optimization problem solving. The deep neural network is first trained to provide referenced global fields, which are combined with exact observations and the reference modes extracted by POD to establish a linear optimization problem. The objective of optimization problem is to superpose POD modes to satisfy the values of observations and referenced fields. The experiments conducted on fluid and thermal field reconstruction problems show that the proposed unified framework can significantly improve the reconstruction accuracy of neural networks and boost the performance of directly solving optimization problems without referenced fields.
更多查看译文
关键词
complex physical systems control,complex physical systems monitoring,deep neural network,fluid reconstruction problems,full-state estimation,gappy proper orthogonal decomposition,linear optimization problem solving,neural network prediction,nonlinear mapping,POD modes,reconstruction performance,referenced global fields,supervised learning methods,thermal field reconstruction problems,unified global field reconstruction framework
AI 理解论文
溯源树
样例
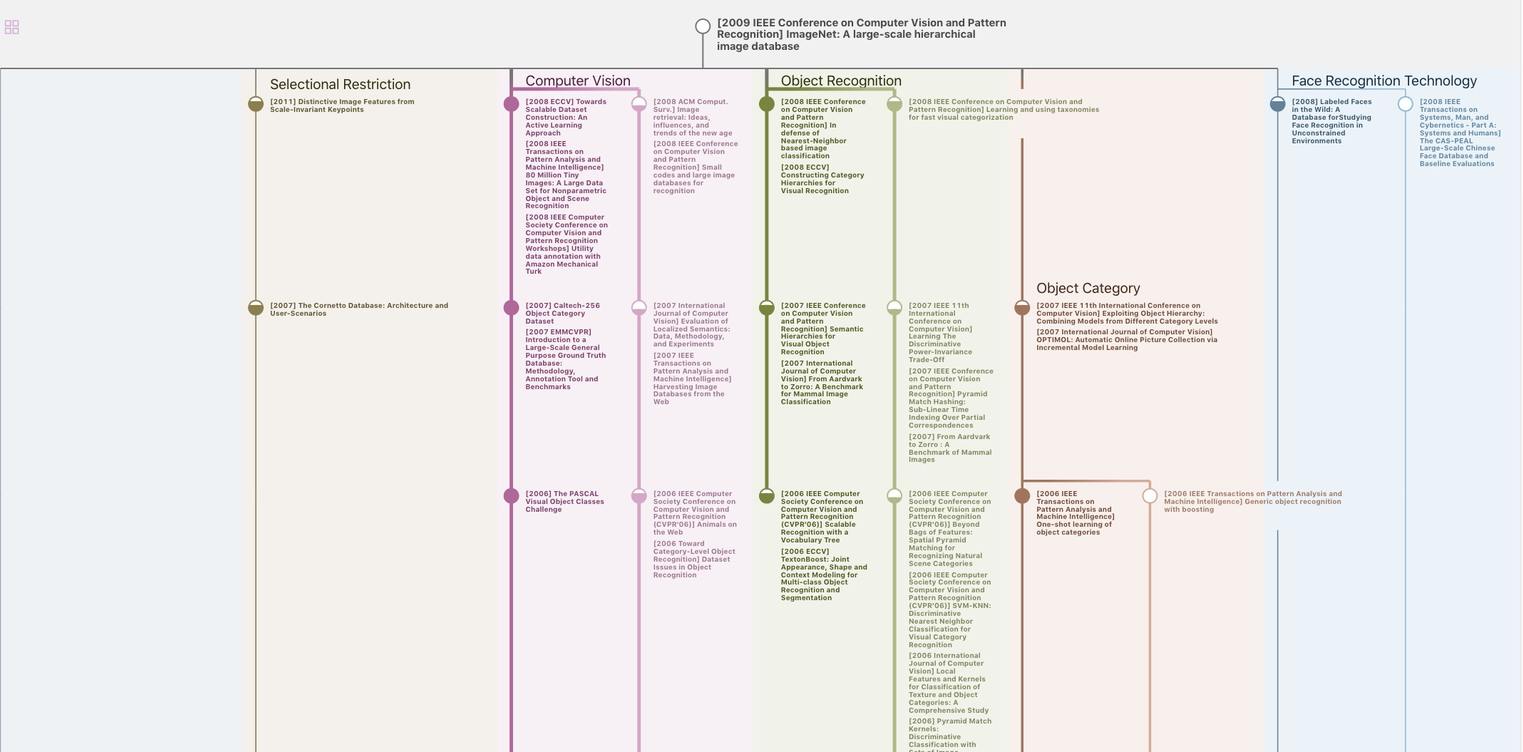
生成溯源树,研究论文发展脉络
Chat Paper
正在生成论文摘要