Multi-Modal Neural Radiance Field for Monocular Dense SLAM with a Light-Weight ToF Sensor
CoRR(2023)
摘要
Light-weight time-of-flight (ToF) depth sensors are compact and cost-efficient, and thus widely used on mobile devices for tasks such as autofocus and obstacle detection. However, due to the sparse and noisy depth measurements, these sensors have rarely been considered for dense geometry reconstruction. In this work, we present the first dense SLAM system with a monocular camera and a light-weight ToF sensor. Specifically, we propose a multi-modal implicit scene representation that supports rendering both the signals from the RGB camera and light-weight ToF sensor which drives the optimization by comparing with the raw sensor inputs. Moreover, in order to guarantee successful pose tracking and reconstruction, we exploit a predicted depth as an intermediate supervision and develop a coarse-to-fine optimization strategy for efficient learning of the implicit representation. At last, the temporal information is explicitly exploited to deal with the noisy signals from light-weight ToF sensors to improve the accuracy and robustness of the system. Experiments demonstrate that our system well exploits the signals of light-weight ToF sensors and achieves competitive results both on camera tracking and dense scene reconstruction. Project page: \url{https://zju3dv.github.io/tof_slam/}.
更多查看译文
关键词
monocular dense slam,multi-modal,light-weight
AI 理解论文
溯源树
样例
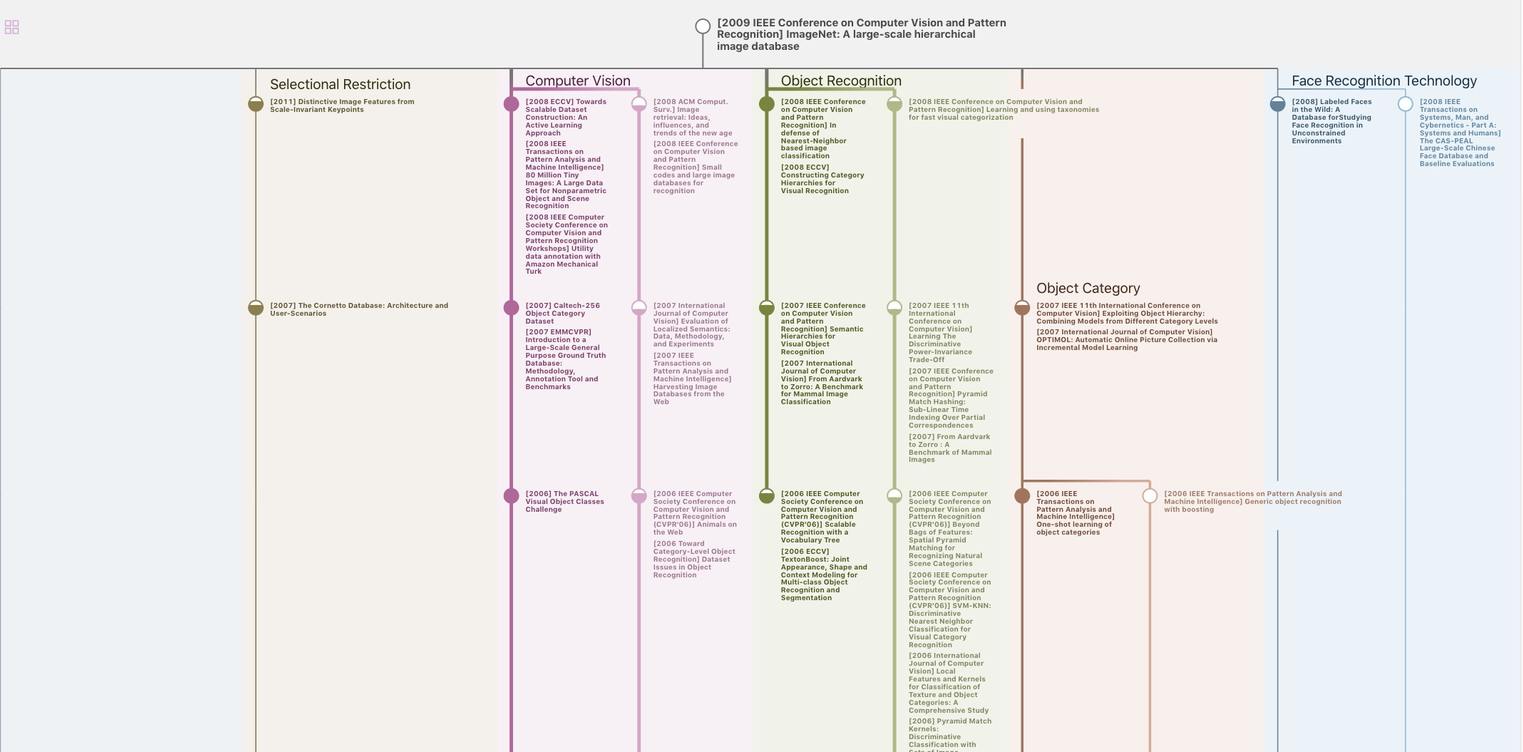
生成溯源树,研究论文发展脉络
Chat Paper
正在生成论文摘要