FunnyBirds: A Synthetic Vision Dataset for a Part-Based Analysis of Explainable AI Methods
CoRR(2023)
摘要
The field of explainable artificial intelligence (XAI) aims to uncover the inner workings of complex deep neural models. While being crucial for safety-critical domains, XAI inherently lacks ground-truth explanations, making its automatic evaluation an unsolved problem. We address this challenge by proposing a novel synthetic vision dataset, named FunnyBirds, and accompanying automatic evaluation protocols. Our dataset allows performing semantically meaningful image interventions, e.g., removing individual object parts, which has three important implications. First, it enables analyzing explanations on a part level, which is closer to human comprehension than existing methods that evaluate on a pixel level. Second, by comparing the model output for inputs with removed parts, we can estimate ground-truth part importances that should be reflected in the explanations. Third, by mapping individual explanations into a common space of part importances, we can analyze a variety of different explanation types in a single common framework. Using our tools, we report results for 24 different combinations of neural models and XAI methods, demonstrating the strengths and weaknesses of the assessed methods in a fully automatic and systematic manner.
更多查看译文
关键词
synthetic vision dataset,part-based
AI 理解论文
溯源树
样例
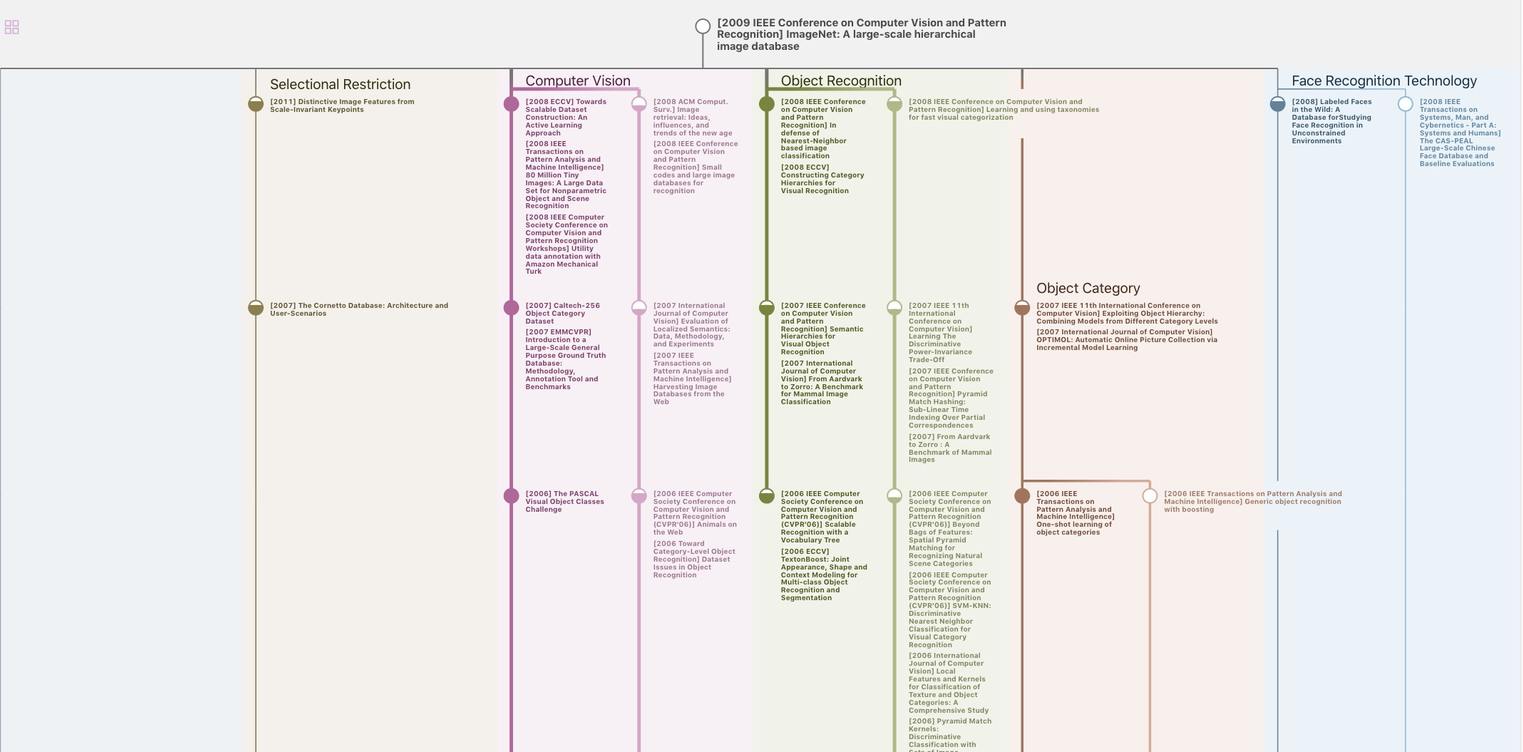
生成溯源树,研究论文发展脉络
Chat Paper
正在生成论文摘要