Learning-Based Near-Optimal Motion Planning for Intelligent Vehicles With Uncertain Dynamics
IEEE ROBOTICS AND AUTOMATION LETTERS(2024)
摘要
Motion planning has been an important research topic in achieving safe and flexible maneuvers for intelligent vehicles. However, it remains challenging to realize efficient and optimal planning in the presence of uncertain model dynamics. In this paper, a sparse kernel-based reinforcement learning (RL) algorithm with Gaussian process (GP) regression (called GP-SKRL) is proposed to realize online adaptation and near-optimal motion planning performance. In this algorithm, we design an efficient sparse GP regression method to learn the uncertain dynamics. Based on the updated model, a sparse kernel-based policy iteration algorithm with an exponential barrier function is designed to learn the near-optimal planning policies with the capability to avoid dynamic obstacles. Thereby, batch-mode GP-SKRL with online adaption capability can estimate the changing system dynamics. The converged RL policies are then deployed on vehicles efficiently under a safety-aware module. As a result, the produced driving actions are safe and less conservative, and the planning performance has been noticeably improved. Extensive simulation results show that GP-SKRL outperforms several advanced motion planning methods in terms of average cumulative cost, trajectory length, and task completion time. In particular, experiments on a Hongqi E-HS3 electric vehicle demonstrate the effectiveness of the proposed GP-SKRL approach.
更多查看译文
关键词
Collision avoidance,integrated planning and control,planning under uncertainty,reinforcement learning
AI 理解论文
溯源树
样例
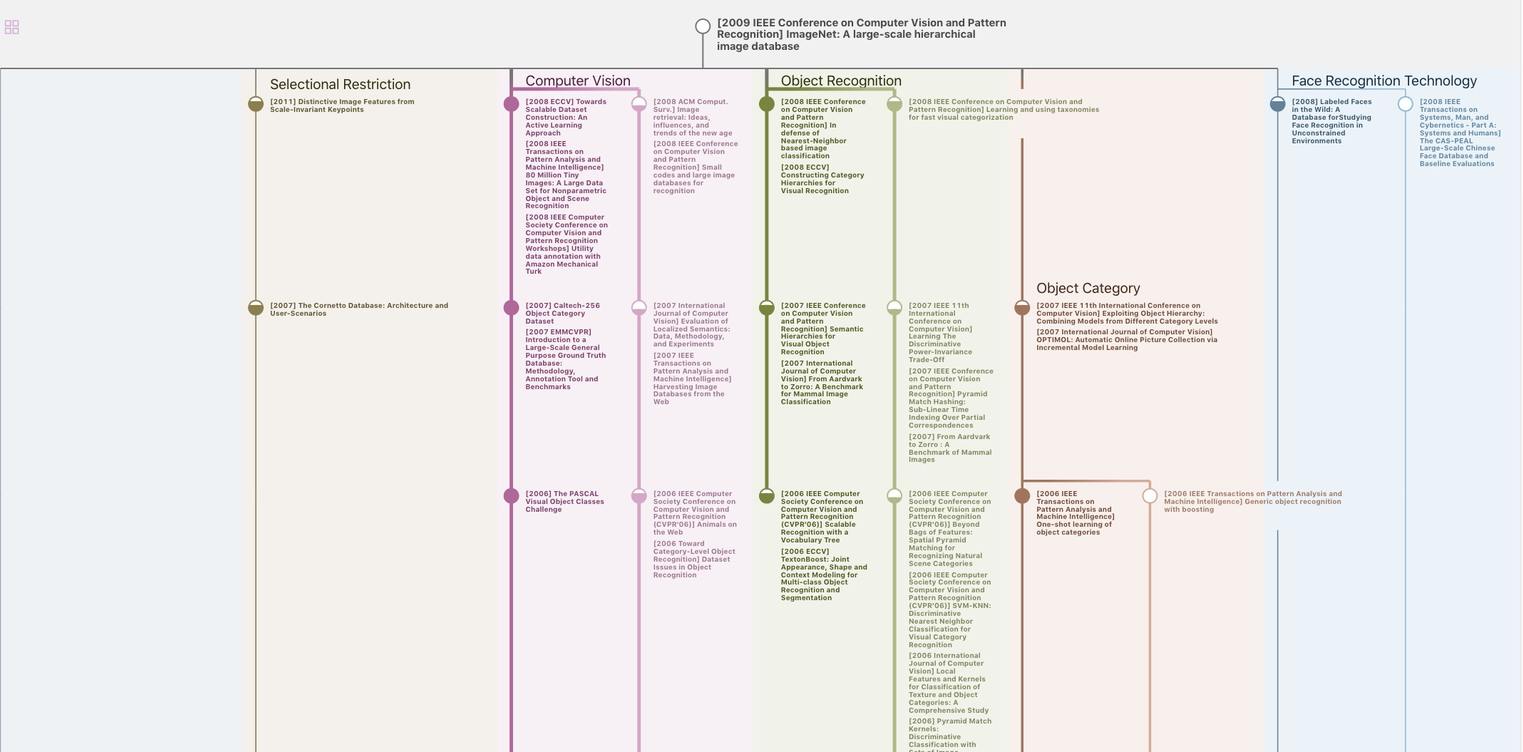
生成溯源树,研究论文发展脉络
Chat Paper
正在生成论文摘要