Identifying Selection Bias from Observational Data.
AAAI(2023)
摘要
Access to a representative sample from the population is an assumption that underpins all of machine learning. Unfortunately, selection effects can cause observations to instead come from a subpopulation, by which our inferences may be subject to bias. It is therefore essential to know whether or not a sample is affected by selection effects. We study under which conditions we can identify selection bias and give results for both parametric and non-parametric families of distributions. Based on these results, we develop two practical methods to determine whether or not an observed sample comes from a distribution subject to selection bias. Through extensive evaluation on synthetic and real-world data, we verify that our methods beat the state of the art both in detecting as well as characterizing selection bias.
更多查看译文
关键词
selection bias,observational data
AI 理解论文
溯源树
样例
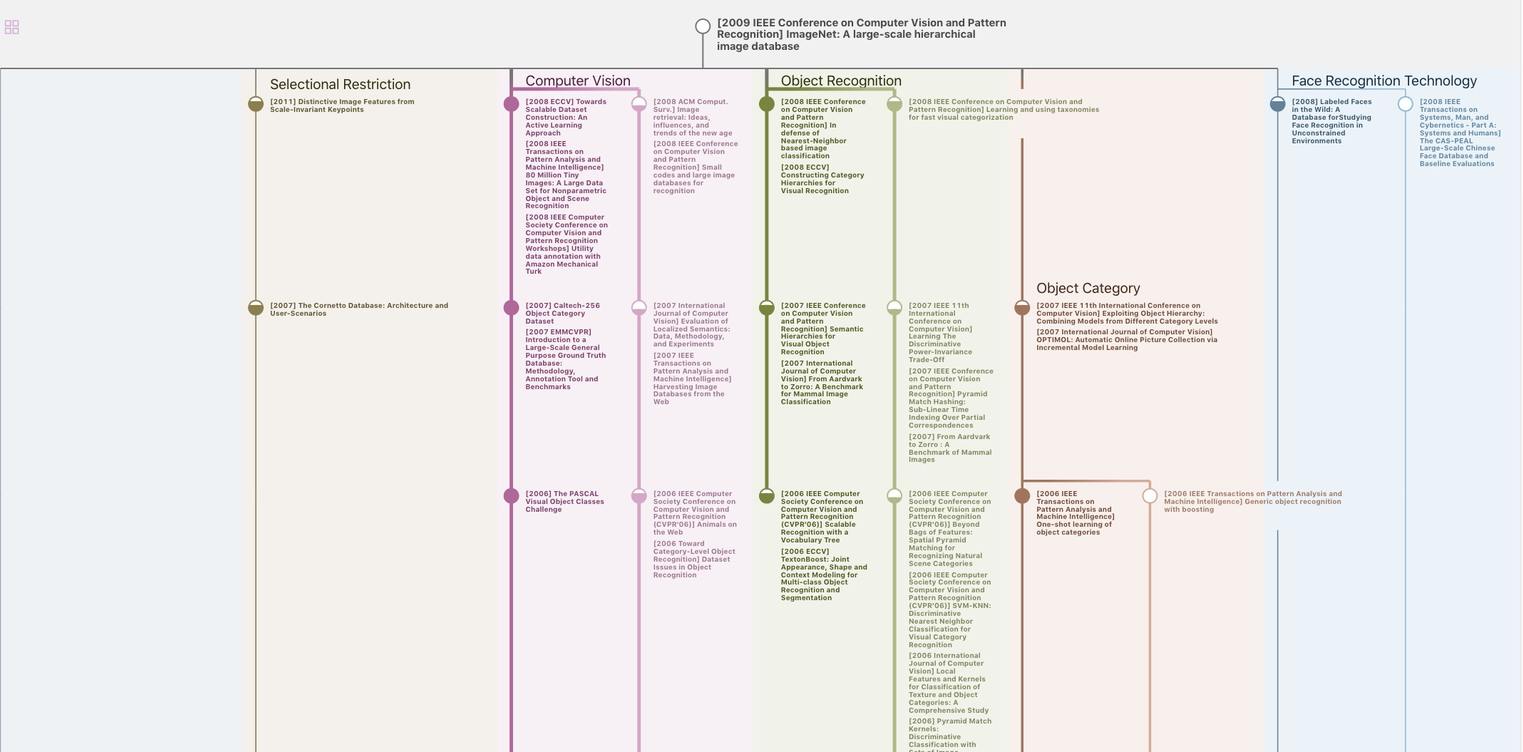
生成溯源树,研究论文发展脉络
Chat Paper
正在生成论文摘要