Enhancing Factorization Machines with Generalized Metric Learning (Extended Abstract).
ICDE(2023)
摘要
Factorization Machines (FMs) have been proven effective in modeling the second-order interactions between attributes. Nevertheless, existing methods often fall short of learning the complex and rich intra-attribute feature interactions, which are abundantly exhibited in real-world recommendation data. To address this problem, we propose an FM framework equipped with generalized metric learning to better handle the essential feature correlations. In particular, based on this framework, we present a Mahalanobis distance and a deep neural network method, which can effectively model the linear and non-linear correlations between features, respectively. Besides, we design an efficient approach for simplifying the model functions. Experiments on several benchmark datasets demonstrate that our proposed framework outperforms several state-of-the-art baselines by a significant margin.
更多查看译文
关键词
complex intra-attribute feature interactions,deep neural network method,factorization machines,FM framework,generalized metric learning,nonlinear correlations,recommendation data
AI 理解论文
溯源树
样例
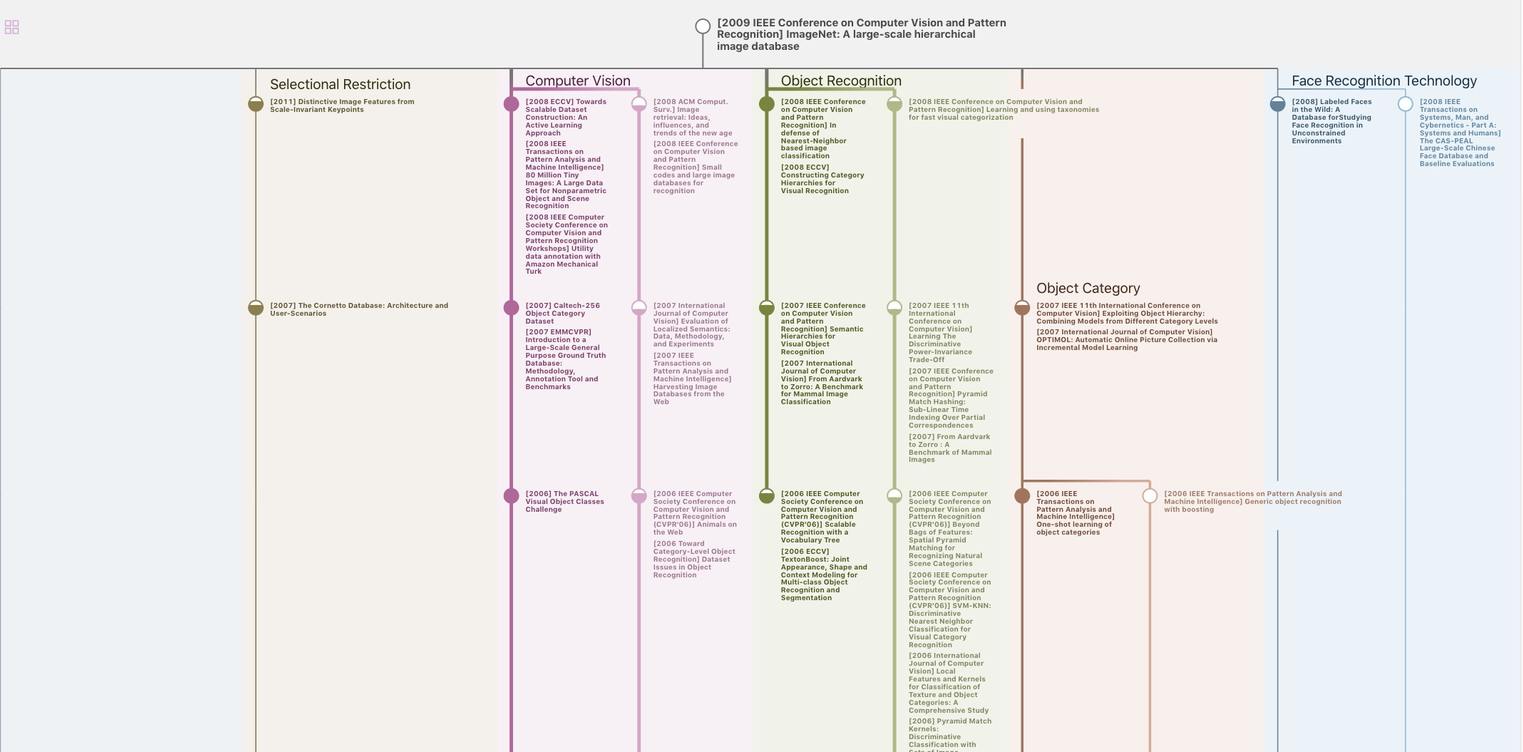
生成溯源树,研究论文发展脉络
Chat Paper
正在生成论文摘要