Exploratory Data Analysis in Electronic Health Records Graphs: Intuitive Features and Visualization Tools
2023 IEEE 36TH INTERNATIONAL SYMPOSIUM ON COMPUTER-BASED MEDICAL SYSTEMS, CBMS(2023)
摘要
Given a large, unlabeled set of Electronic Health Records (EHRs) acquired from multiple hospitals, how can we analyze the available entities and identify relationships in the data? Also, how can we perform Exploratory Data Analysis (EDA) over such EHR data? Many medical institutions generate EHRs as tabular data with entities and attributes in common. However, due to a large number of records, attributes, and high cardinality, exploring the different datasets and finding patterns and insights become laborious and prone to errors. In this work, we propose GraF-EDA for EDA over EHR data from different institutions. GraF-EDA models EHRs as time-evolving graphs, allowing the interoperability of such data into a single representation. We extract meaningful features from the graph nodes and provide intuitive visualizations to improve data explainability. We evaluate GraF-EDA with four COVID-19 datasets from hospitals of the Sao Paulo state, Brazil, resulting in million-scale graphs. Our method identified correlations, similarities and dissimilarities among medical treatments, exams, clinics, and outcomes. With the visual tools provided by GraF-EDA, we were able to spot cases of interest and check more details about them. Our results indicate that GraF-EDA is a fast, effective, open-sourced tool for EDA of EHRs from multiple institutions.
更多查看译文
关键词
Exploratory data analysis,electronic health records,graph mining,visualization,features
AI 理解论文
溯源树
样例
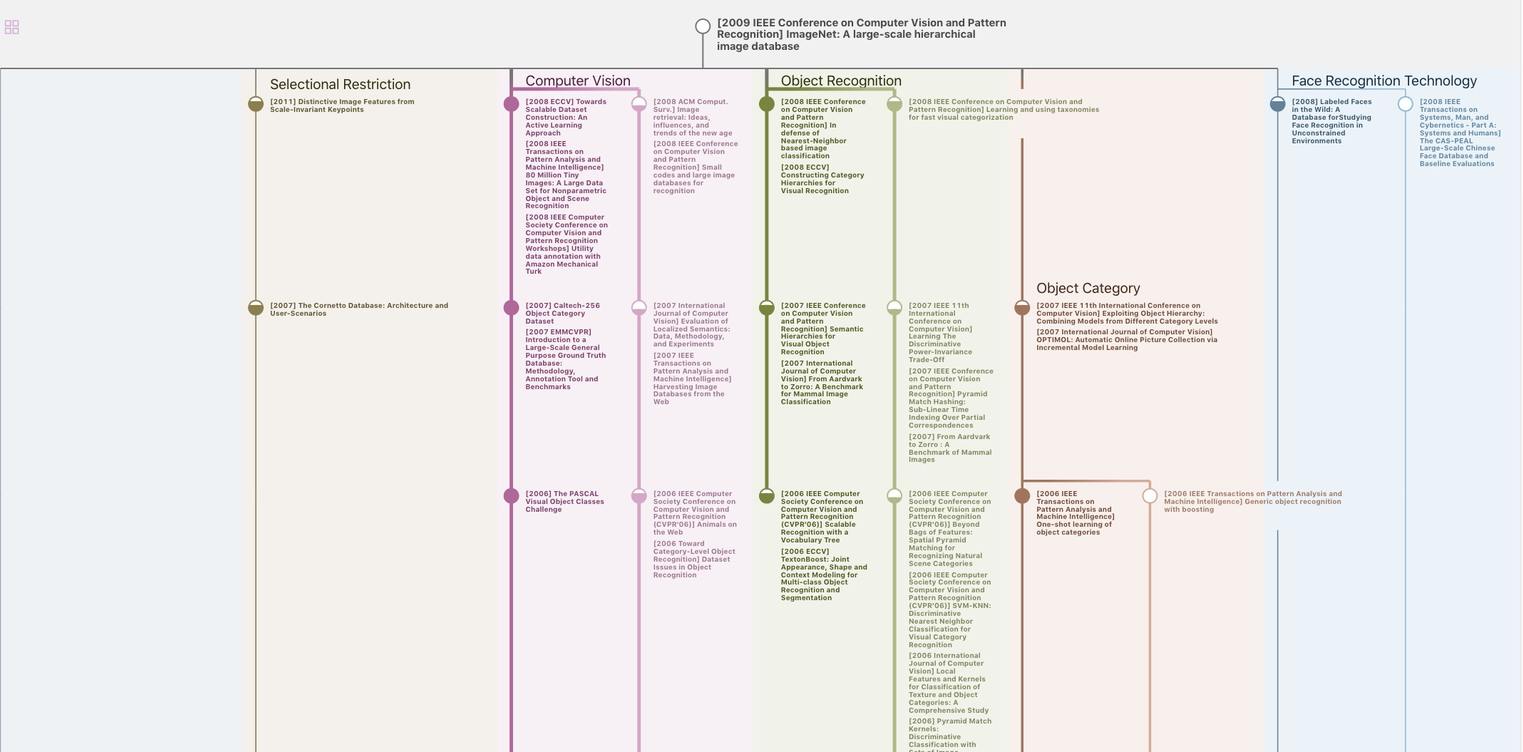
生成溯源树,研究论文发展脉络
Chat Paper
正在生成论文摘要