Discovering Temporal Patterns for Event Sequence Clustering via Policy Mixture Model (Extended Abstract).
ICDE(2023)
摘要
We focus on the problem of event sequence clustering with different temporal patterns from the view of Reinforcement Learning (RL), whereby the observed sequences are assumed to be generated from a mixture of latent policies. We propose an Expectation-Maximization (EM) based algorithm to cluster the sequences with different temporal patterns into the underlying policies while simultaneously learning each of the policy model, in E-step estimating the cluster labels for each sequence, in M-step learning the respective policy. For each policy learning, we resort to Inverse Reinforcement Learning (IRL) by decomposing the observed sequence into states (hidden embedding of event history) and actions (time interval to next event) in order to learn a reward function. Experiments on synthetic and real-world datasets show the efficacy of our method against the state-of-the-arts.
更多查看译文
关键词
n/a
AI 理解论文
溯源树
样例
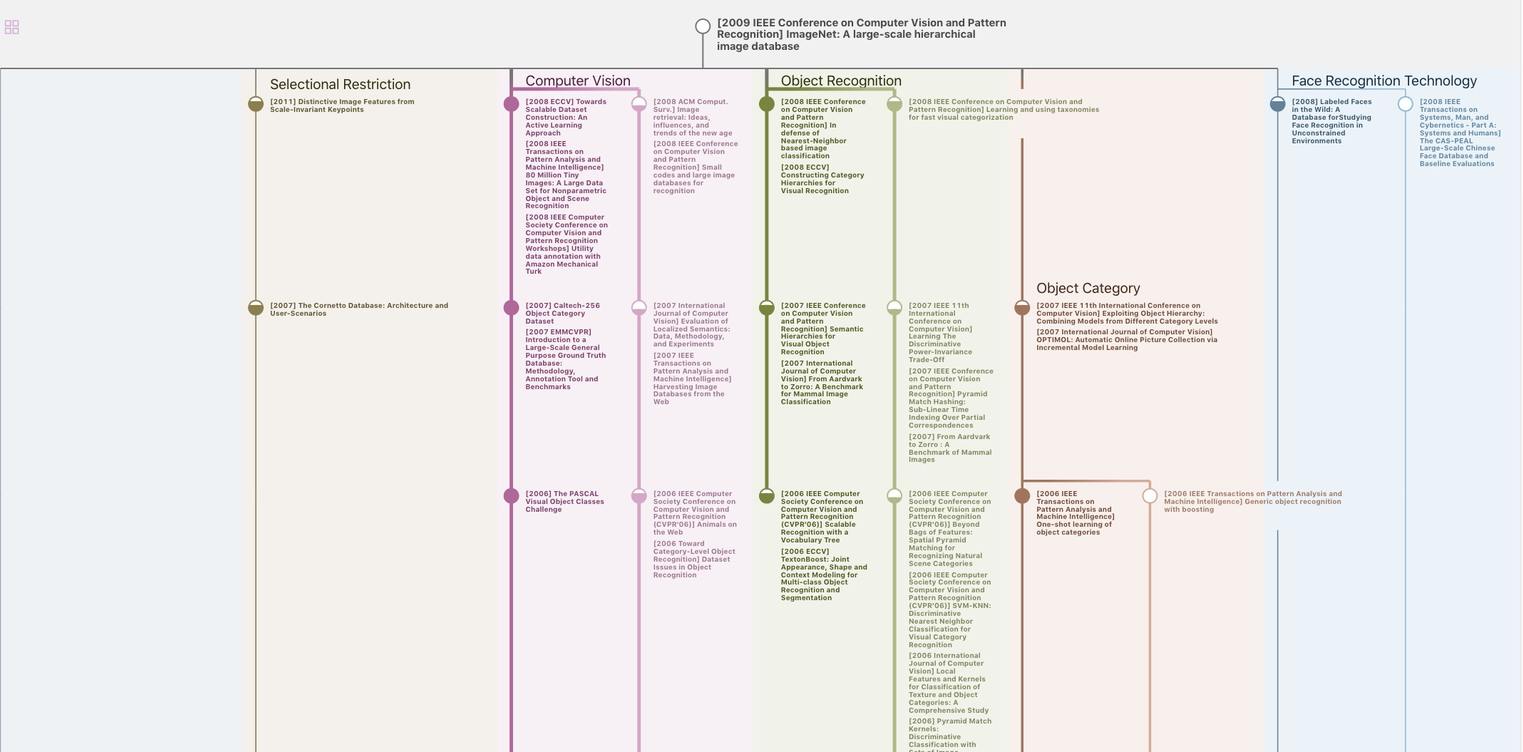
生成溯源树,研究论文发展脉络
Chat Paper
正在生成论文摘要