Thompson Sampling for Partially Observable Linear-Quadratic Control
2023 AMERICAN CONTROL CONFERENCE, ACC(2023)
摘要
Thompson Sampling (TS) is a popular method for decision-making under uncertainty, where an action is sampled from a carefully constructed distribution based on the data collected. In this work, we study the problem of adaptive control in partially observable linear quadratic Gaussian, i.e., LQG, control systems using TS, when the model dynamics are unknown. Prior works have established an (O) over bar(root T) regret upper bound for the adaptive control of such systems, after T time steps. However, the algorithms that achieve this result employ computationally intractable policies. We propose an efficient TS-based adaptive control algorithm, Thompson Sampling under Partial Observability TSPO, to effectively balance the exploration vs. exploitation trade-off and minimize the overall control cost in epochs. TSPO utilizes closed-loop system identification to estimate the underlying model parameters up to their confidence intervals. It then deploys the optimal policy of a sampled system, which is selected at random from the distribution constructed with the model estimates and their confidence intervals. We show that using only logarithmic policy updates, TSPO attains (O) over bar (root T) regret against the optimal control policy that knows the system dynamics. To the best of our knowledge, TSPO is the first computationally efficient algorithm that achieves (O) over bar (root T) regret in adaptive control of unknown partially observable LQG control systems with convex cost. Further, we empirically study the performance of TSPO in an adaptive measurement-feedback control problem.
更多查看译文
AI 理解论文
溯源树
样例
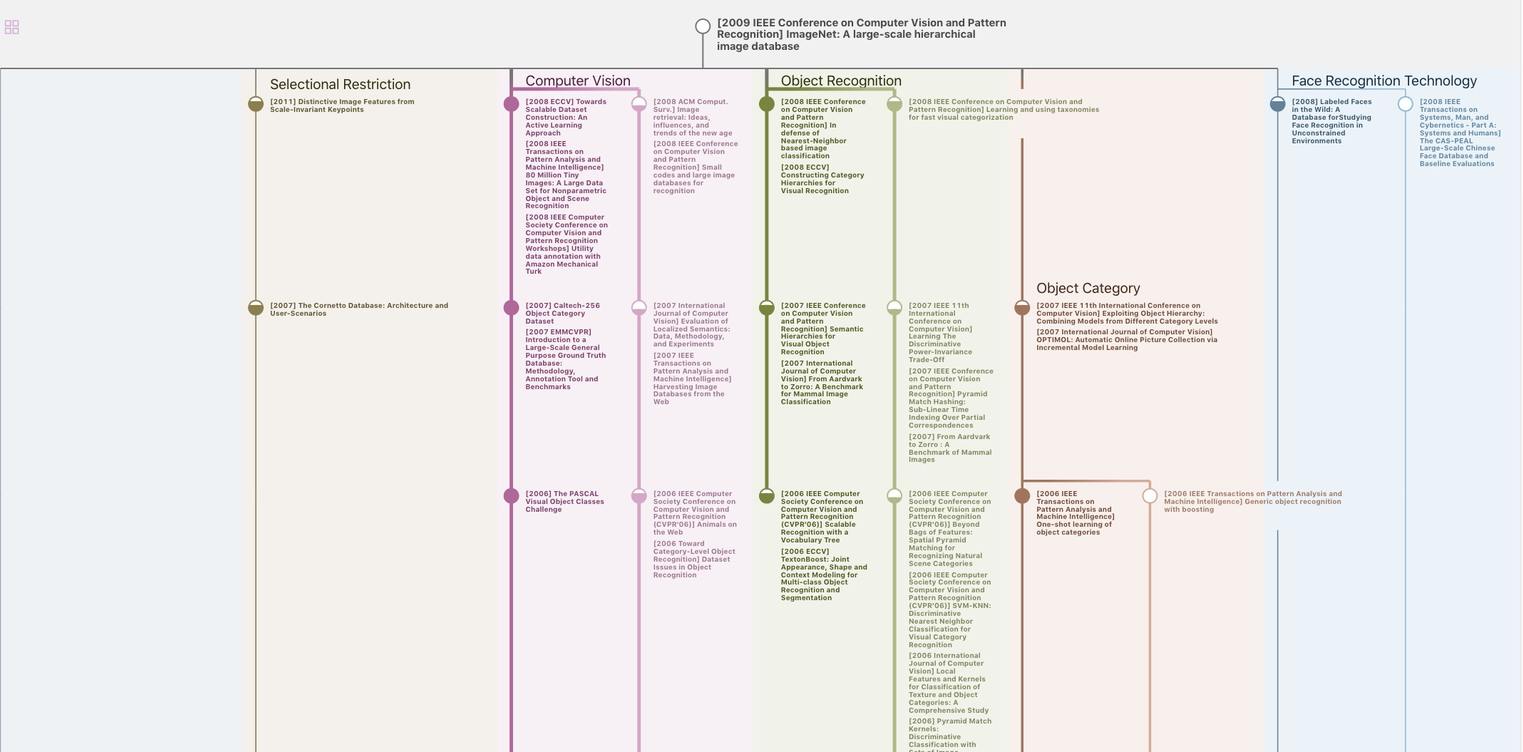
生成溯源树,研究论文发展脉络
Chat Paper
正在生成论文摘要