Data-Driven Robust Optimal Iterative Learning Control of Linear Systems with Strong Cross-Axis Coupling.
ACC(2023)
摘要
In this paper, a data-driven iterative learning control approach to multi-input-multi-output (MIMO) systems with strong cross-axis coupling is proposed. Iterative learning control (ILC) of MIMO systems with strong cross-axis coupling effect is challenging as model-based ILC to MIMO systems is complicated by the modeling process of MIMO systems being involved and time-consuming, the trade-off between the model accuracy and the performance, and the limitation to systems with weak-cross-coupling. Contrarily, constant gain ILC methods are mainly effective for tracking at low-speed, and suffer from slow convergence, particularly in the presence of the random disturbances. Thus, the aim of this paper is to develop a data-driven robust optimal ILC (DDRO-ILC) approach to MIMO systems of strong cross-axis coupling under random output disturbance. The iteration gain is constructed and updated by using past input and output data to capture the dynamics of the system via the singular value decomposition (SVD) technique. It is shown that monotonic convergence in the presence of random disturbance is guaranteed, and an optimal gain can be obtained to maximize the convergence rate and minimize the residual error. The proposed DDRO-ILC technique is illustrated through a numerical simulation on a three-input three-output linear time invariant system model, and compared to the multi-axis inversion-based iterative control (MAIIC) technique. The simulation shows that the proposed DDRO-ILC outperformed the MAIIC method when the cross-axis coupling is strong, and achieved precision tacking with rapid convergence in the presence of random disturbance.
更多查看译文
AI 理解论文
溯源树
样例
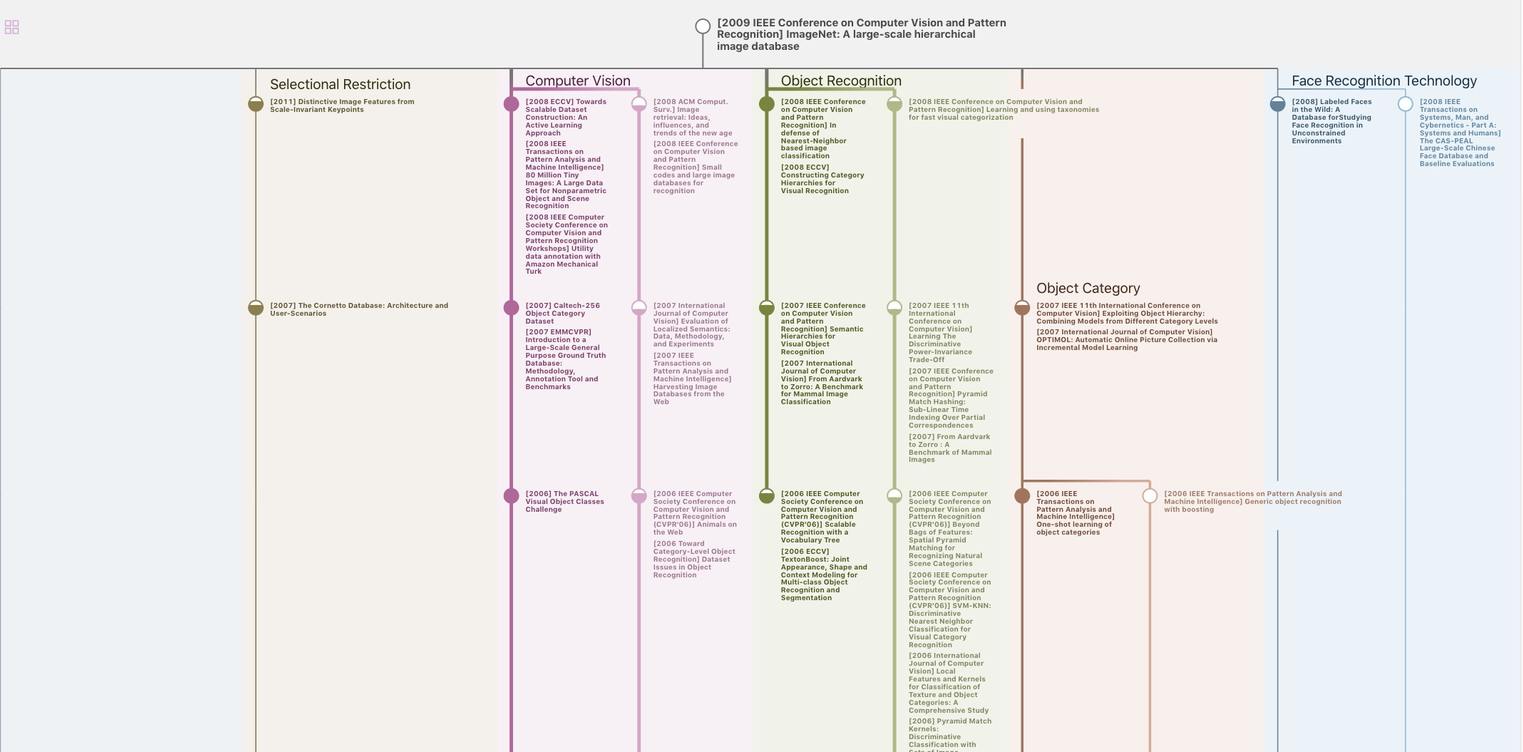
生成溯源树,研究论文发展脉络
Chat Paper
正在生成论文摘要