Application of Entropy for Automated Detection of Neurological Disorders With Electroencephalogram Signals: A Review of the Last Decade (2012-2022).
IEEE Access(2023)
摘要
An automated Neurological Disorder detection system can be considered as a cost-effective and resource efficient tool for medical and healthcare applications. In automated Neurological Disorder detection, electroencephalograms are commonly used, but their low signal intensity and nonlinear features are difficult to analyze visually. A promising approach for processing of electroencephalogram signals is the concept of entropy, a nonlinear signal processing method to measure the chaos in the signal. The aim of this study was to find out the effective entropy measures and the machine learning approaches that produced promising output. Using Preferred Reporting Items for Systematic Reviews and Meta-Analyses (PRISMA) guidelines as our method, we have identified 84 studies published between 2012 and 2022 that has investigated epilepsy, Parkinson's disease, autism, Attention Deficit Hyperactive disorder, schizophrenia, Alzheimer's disease, depression, and alcohol use disorder with machine learning approaches considering entropy measures. We show that Support Vector Machines was the most commonly used machine learning model, with consistent performance in most of the studies whereas sample entropy was the most commonly used entropy measure, followed by the approximate entropy. For epilepsy detection, the most used entropy feature was the log energy entropy, whereas the multi-scale entropy was commonly used for Alzheimer's Disease, approximate and sample entropy used for Parkinson's Disease, multi scale and Shannon entropy applied for autism, approximate and Shannon entropy used for attention deficit hyperactive disorder, sample entropy used for depression, approximate and spectral entropy adopted for schizophrenia, and the approximate and sample entropy employed for alcohol use disorder. According to the majority of the studies, there is growing concern about the increase in neuro patients and the heavy resource burden that is associated with their prevalence and diagnosis. Based on these studies, we conclude that Computer-Aided Design systems would be economically advantageous in detecting Neurological Disorders. To incorporate Computer-Aided Design system into the mainstream health care system, future research could focus on multi-modal approaches to the disorder and its interpretation and explanation. We believe this is the first review that has combined the electroencephalograms, entropy, and automated detection possibility of the 8 distinct neurological disorders. The study is limited to the papers that used accuracy as their performance evaluation metric. The findings and synthesis of previous studies provides a clear pathway that identifies the entropy approach as a practical solution for automated detection of neurological disorder using electroencephalograms with potential applications in other kinds of signal analysis.
更多查看译文
关键词
electroencephalogram signals,entropy,neurological disorders,automated detection
AI 理解论文
溯源树
样例
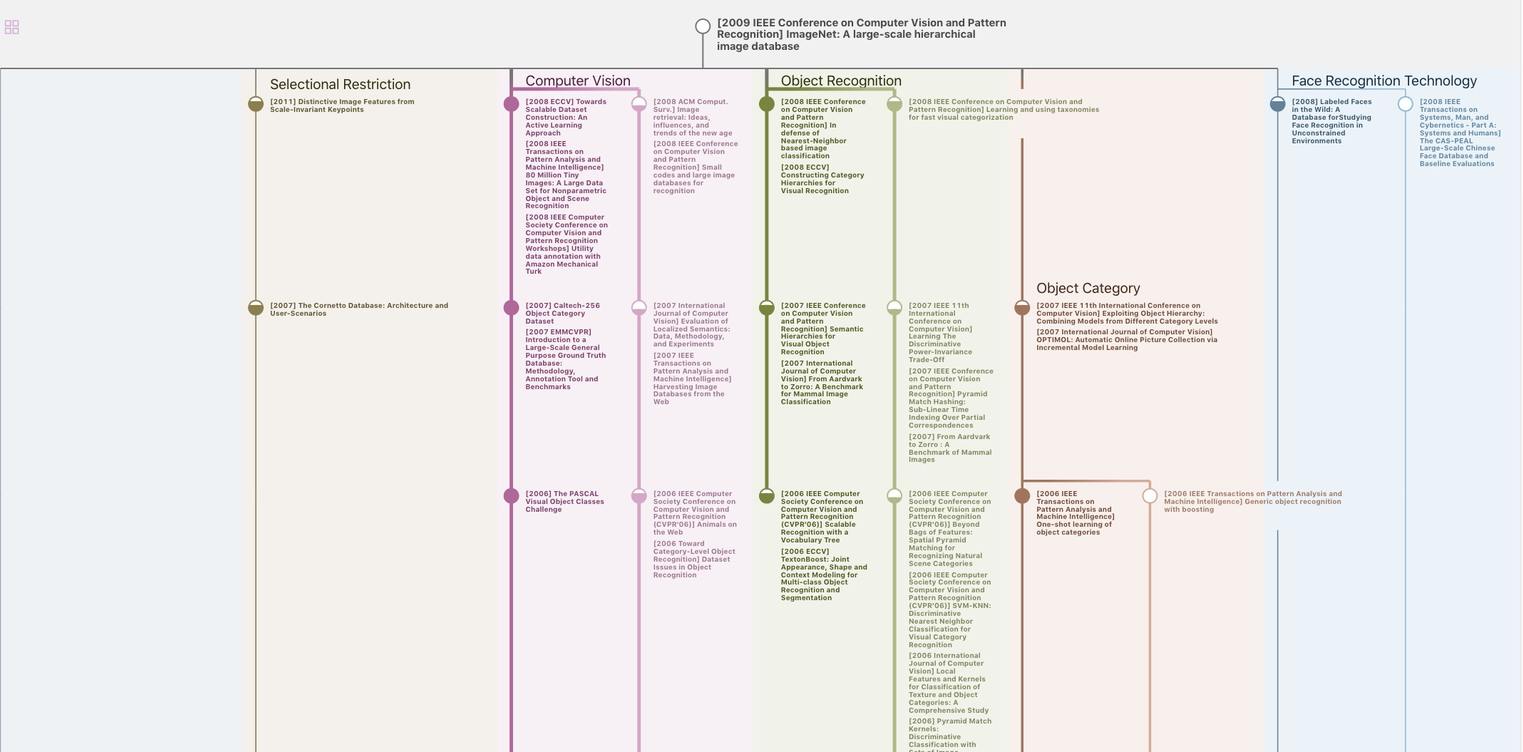
生成溯源树,研究论文发展脉络
Chat Paper
正在生成论文摘要