Self-supervised Classification of Clinical Multivariate Time Series using Time Series Dynamics.
KDD(2023)
摘要
To improve the accuracy of clinical multivariate time series (MTS) classification (such as EEG and ECG) by a novel self-supervised paradigm that directly captures the dynamics between the different time series learned together to optimize the classification task. Labels in clinical datasets are very often insufficient. One way to address this challenge is leveraging self-supervision. This paradigm attempts to identify a supervisory signal inherent within a dataset to serve as a surrogate label. We present a novel form of self-supervision: dynamics of clinical MTS. Unlike other self-supervision methods, such as masking, that are intuitive but still heuristic, we suggest to learn a representation justified by Koopman theory. The latter was shown useful for representing clinical time series and can be used as a form of surrogate task to improve the clinical MTS classification. In the ECG task, we show that our proposed framework achieved higher sensitivity and specificity than the state-of-the-art (SOTA) baseline over numerous common diagnoses. For EEG abnormality classification, our proposed framework also achieved higher sensitivity and specificity than the SOTA baseline. All results are statistically significant. Our technique yields reliable clinical diagnosis in an empirical study employing signals from thousands of patients in multiple clinical tasks employing two types of clinical-grade sensors (ECG and EEG) as compared to the state-of-the-art machine learning. Leveraging time-series-dynamics self-supervision can help mitigate the lack of labels in clinical datasets used for training machine learning algorithms and significantly improve their performance. Specifically, the ECG system presented in this work is being trialed in hospitals, used by top cardiologists for patient diagnosis and treatment. We believe that the deployment of such cutting-edge technology will significantly improve the accuracy and speed of cardiac assessments.
更多查看译文
关键词
Clinical Multivariate Time Series,Koopman Operator,ECG classification,EEG classification
AI 理解论文
溯源树
样例
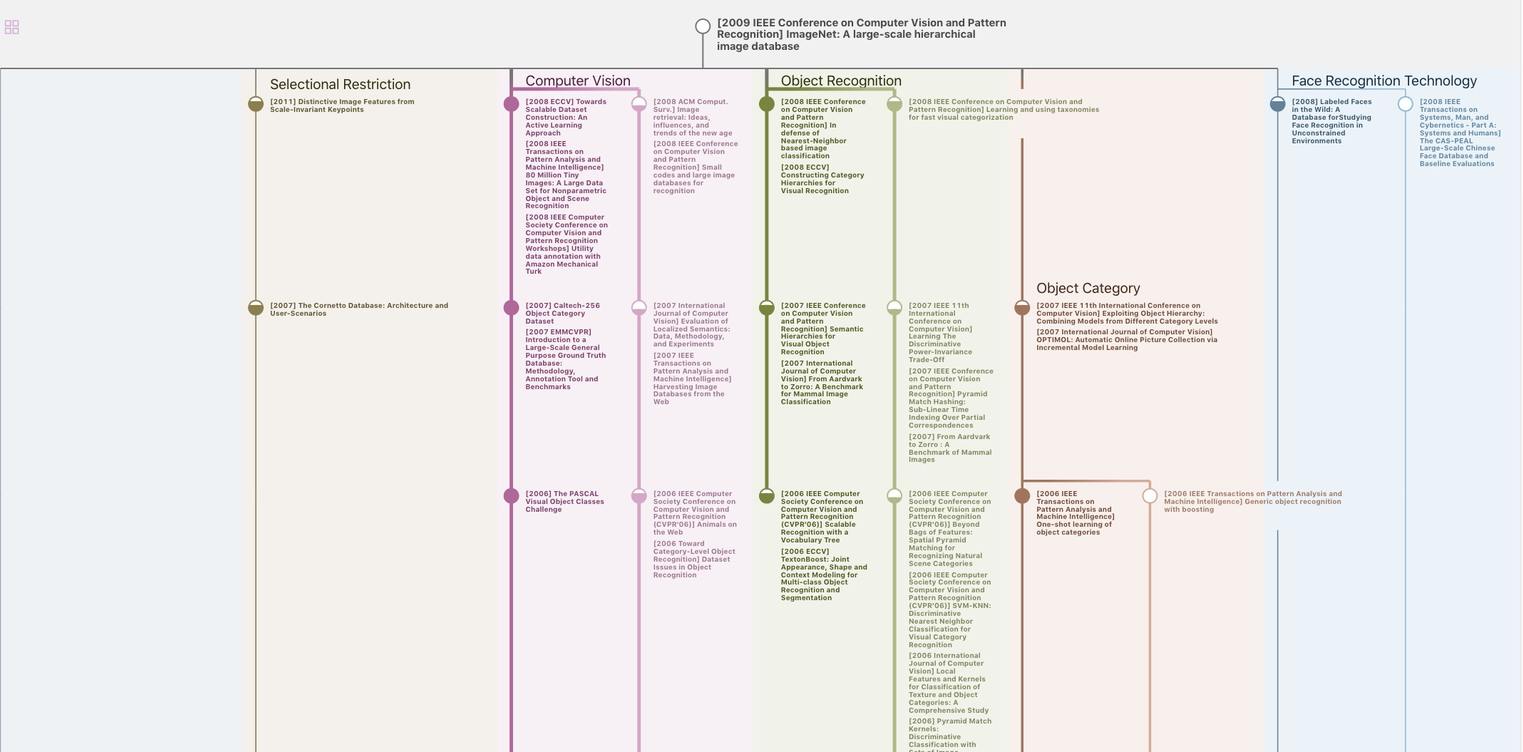
生成溯源树,研究论文发展脉络
Chat Paper
正在生成论文摘要