PASS: Personalized Advertiser-aware Sponsored Search.
KDD(2023)
摘要
The nucleus of online sponsored search systems lies in measuring the relevance between the search intents of users and the advertising purposes of advertisers. Existing conventional doublet-based (query-keyword) relevance models solely rely on short queries and keywords to uncover such intents, which ignore the diverse and personalized preferences of participants (i.e., users and advertisers), resulting in undesirable advertising performance. In this paper, we investigate the novel problem of Personalized Advertiser-aware Sponsored Search (PASS). Our motivation lies in incorporating the portraits of users and advertisers into relevance models to facilitate the modeling of intrinsic search intents and advertising purposes, leading to a quadruple-based (i.e., user-query-keyword-advertiser) task. Various types of historical behaviors are explored in the format of hypergraphs to provide abundant signals on identifying the preferences of participants. A novel heterogeneous textual hyper-graph transformer is further proposed to deeply fuse the textual semantics and the high-order hypergraph topology. Our proposal is extensively evaluated over real industry datasets, and experimental results demonstrate its superiority.
更多查看译文
关键词
Sponsored Search,Hypergraph Learning,Relevance Modeling
AI 理解论文
溯源树
样例
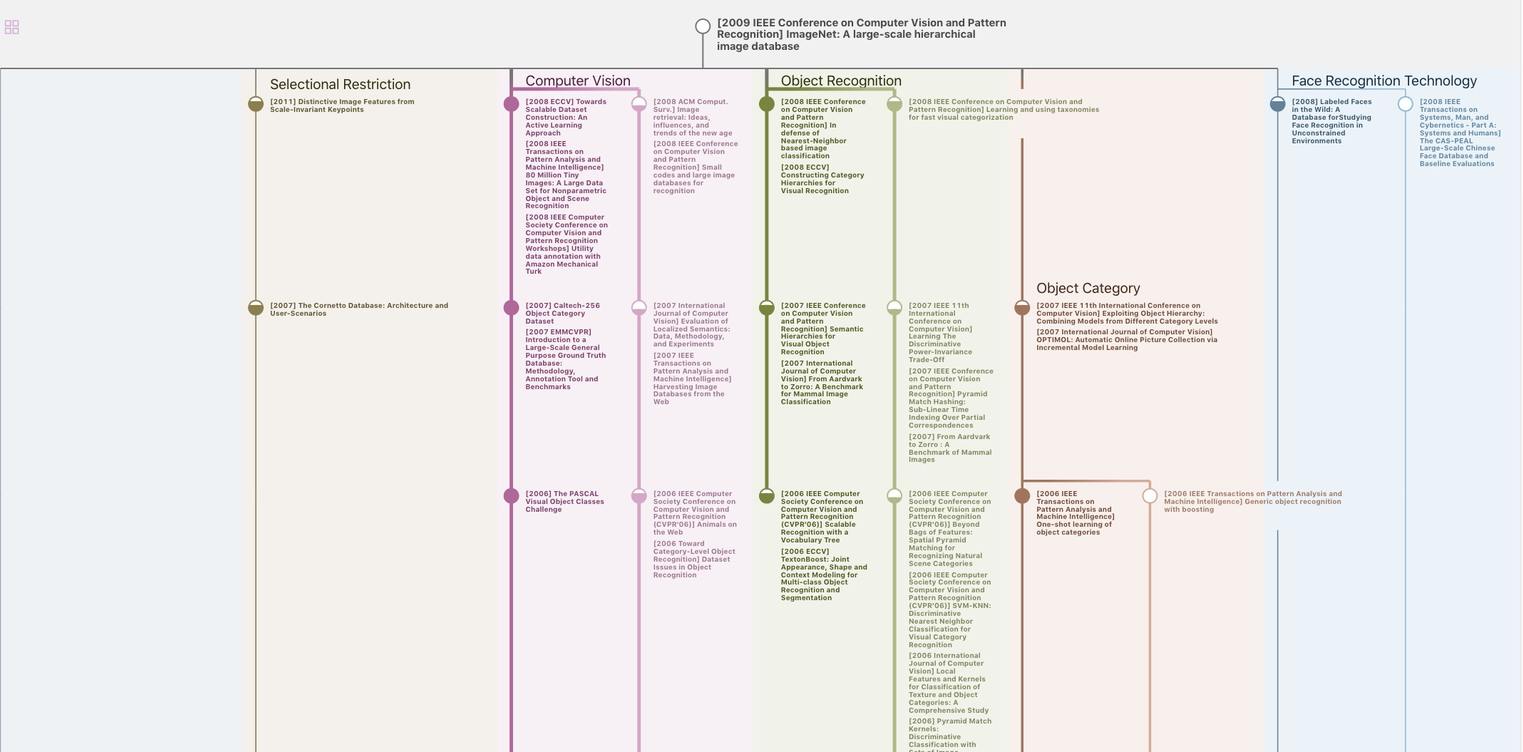
生成溯源树,研究论文发展脉络
Chat Paper
正在生成论文摘要