Multi-modal deep learning improves grain yield prediction in wheat breeding by fusing genomics and phenomics
Bioinformatics (Oxford, England)(2023)
摘要
Motivation: Developing new crop varieties with superior performance is highly important to ensure robust and sustainable global food security. The speed of variety development is limited by long field cycles and advanced generation selections in plant breeding programs. While methods to predict yield from genotype or phenotype data have been proposed, improved performance and integrated models are needed. Results: We propose a machine learning model that leverages both genotype and phenotype measurements by fusing genetic variants with multiple data sources collected by unmanned aerial systems. We use a deep multiple instance learning framework with an attention mechanism that sheds light on the importance given to each input during prediction, enhancing interpretability. Our model reaches 0.75460.024 Pearson correlation coefficient when predicting yield in similar environmental conditions; a 34.8% improvement over the genotype-only linear baseline (0.55960.050). We further predict yield on new lines in an unseen environment using only genotypes, obtaining a prediction accuracy of 0.38660.010, a 13.5% improvement over the linear baseline. Our multi-modal deep learning architecture efficiently accounts for plant health and environment, distilling the genetic contribution and providing excellent predictions. Yield prediction algorithms leveraging phenotypic observations during training therefore promise to improve breeding programs, ultimately speeding up delivery of improved varieties.
更多查看译文
关键词
grain yield prediction,grain yield,wheat,deep learning,multi-modal
AI 理解论文
溯源树
样例
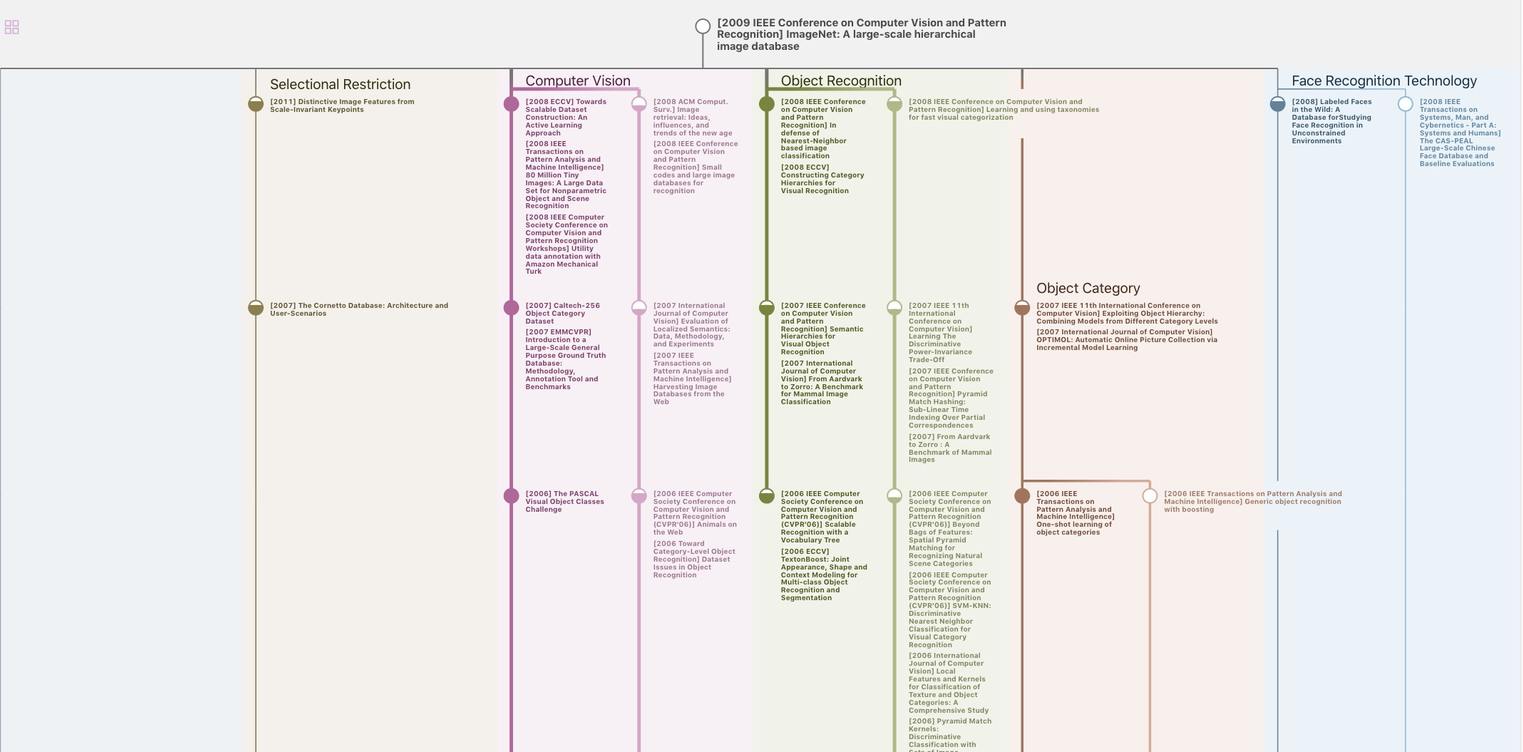
生成溯源树,研究论文发展脉络
Chat Paper
正在生成论文摘要