DEcancer: Machine learning framework tailored to liquid biopsy based cancer detection and biomarker signature selection
ISCIENCE(2023)
摘要
Cancer is a leading cause of mortality worldwide. Over 50% of cancers are diagnosed late, rendering many treatments ineffective. Existing liquid biopsy studies demonstrate a minimally invasive and inexpensive approach for dis-ease detection but lack parsimonious biomarker selection, exhibit poor cancer detection performance and lack appropriate validation and testing. We estab-lished a tailored machine learning pipeline, DEcancer, for liquid biopsy anal-ysis that addresses these limitations and improved performance. In a test set from a published cohort of 1,005 patients including 8 cancer types and 812 cancer-free individuals, DEcancer increased stage 1 cancer detection sensitivity across cancer types from 48 to 90%. In addition, with a test set cohort of patients from a high dimensional proteomics dataset of 61 lung can-cer patients and 80 cancer-free individuals, DEcancer's performance using a 14-43 protein panel was comparable to 1,000 original proteins. DEcancer is a promising tool which may facilitate improved cancer detection and management.
更多查看译文
关键词
Diagnostics,Cancer,Machine learning
AI 理解论文
溯源树
样例
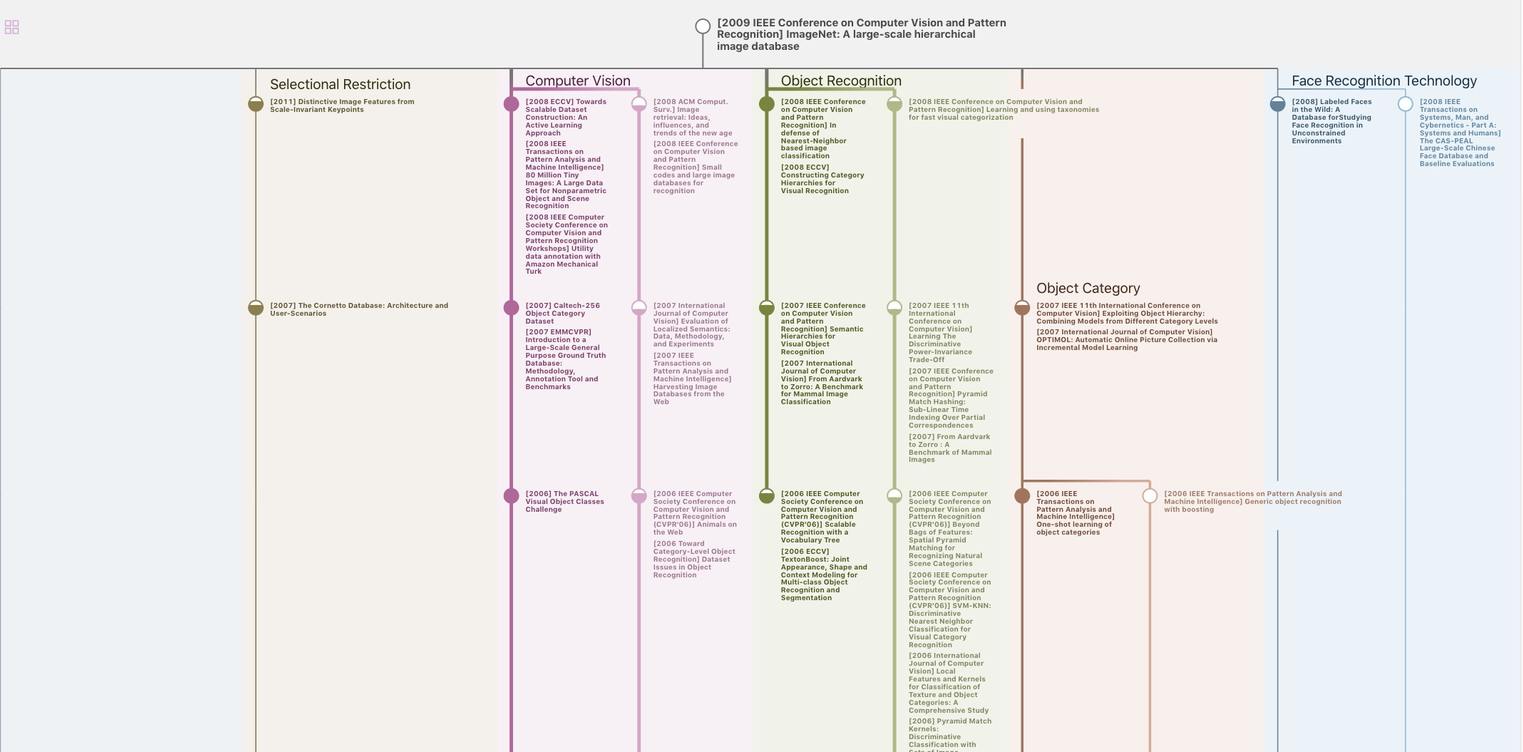
生成溯源树,研究论文发展脉络
Chat Paper
正在生成论文摘要