Concept2Box: Joint Geometric Embeddings for Learning Two-View Knowledge Graphs
conf_acl(2023)
摘要
Knowledge graph embeddings (KGE) have been extensively studied to embed large-scale relational data for many real-world applications. Existing methods have long ignored the fact many KGs contain two fundamentally different views: high-level ontology-view concepts and fine-grained instance-view entities. They usually embed all nodes as vectors in one latent space. However, a single geometric representation fails to capture the structural differences between two views and lacks probabilistic semantics towards concepts' granularity. We propose Concept2Box, a novel approach that jointly embeds the two views of a KG using dual geometric representations. We model concepts with box embeddings, which learn the hierarchy structure and complex relations such as overlap and disjoint among them. Box volumes can be interpreted as concepts' granularity. Different from concepts, we model entities as vectors. To bridge the gap between concept box embeddings and entity vector embeddings, we propose a novel vector-to-box distance metric and learn both embeddings jointly. Experiments on both the public DBpedia KG and a newly-created industrial KG showed the effectiveness of Concept2Box.
更多查看译文
关键词
joint geometric embeddings,knowledge
AI 理解论文
溯源树
样例
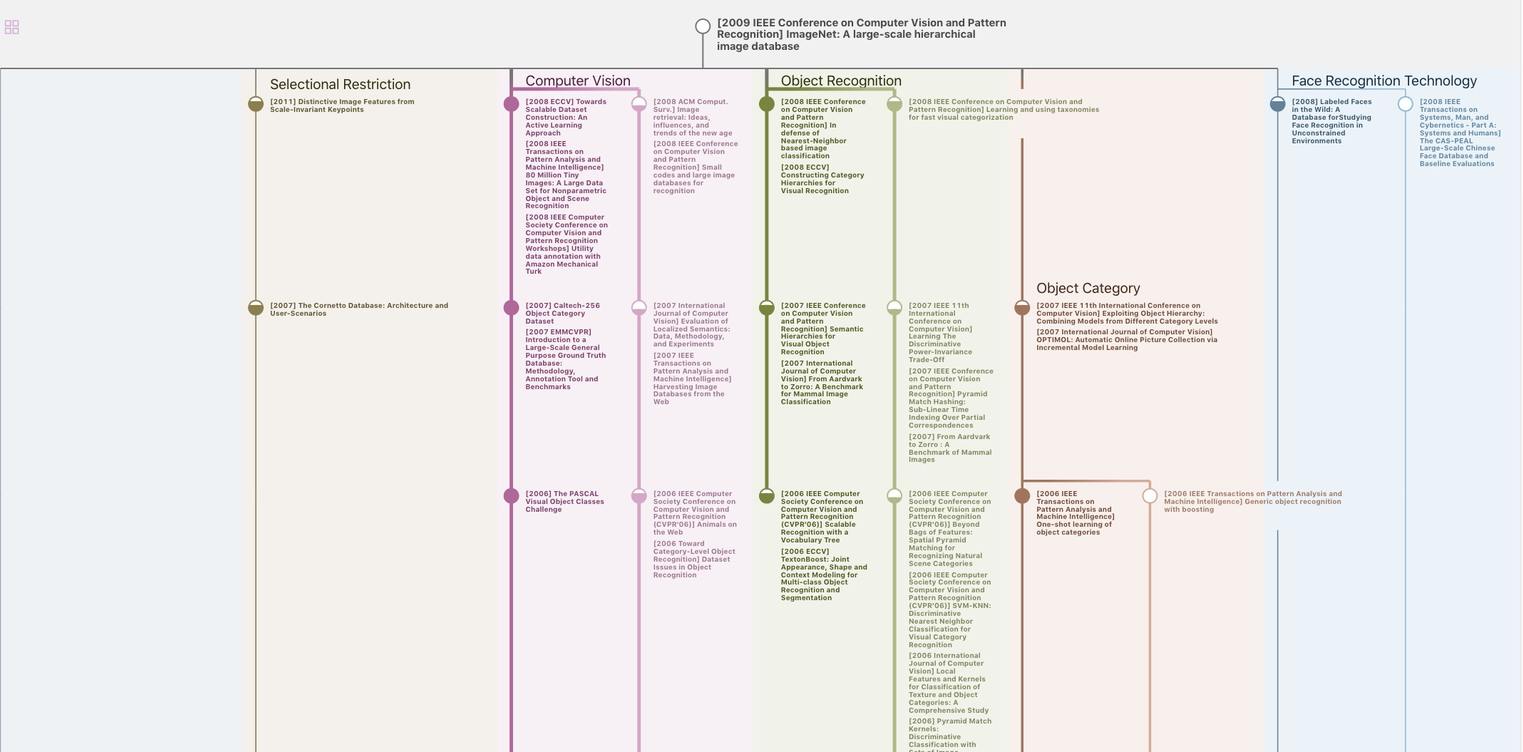
生成溯源树,研究论文发展脉络
Chat Paper
正在生成论文摘要