Projected Tensor Power Method for Hypergraph Community Recovery
ICML 2023(2023)
摘要
This paper investigates the problem of exact community recovery in the symmetric $d$-uniform $(d \geq 2)$ hypergraph stochastic block model ($d$-HSBM). In this model, a $d$-uniform hypergraph with $n$ nodes is generated by first partitioning the $n$ nodes into $K\geq 2$ equal-sized disjoint communities and then generating hyperedges with a probability that depends on the community memberships of $d$ nodes. Despite the non-convex and discrete nature of the maximum likelihood estimation problem, we develop a simple yet efficient iterative method, called the \emph{projected tensor power method}, to tackle it. As long as the initialization satisfies a partial recovery condition in the logarithmic degree regime of the problem, we show that our proposed method can exactly recover the hidden community structure down to the information-theoretic limit with high probability. Moreover, our proposed method exhibits a competitive time complexity of $\mathcal{O}(n\log^2n/\log\log n)$ when the aforementioned initialization condition is met. We also conduct numerical experiments to validate our theoretical findings.
更多查看译文
关键词
hypergraph community recovery
AI 理解论文
溯源树
样例
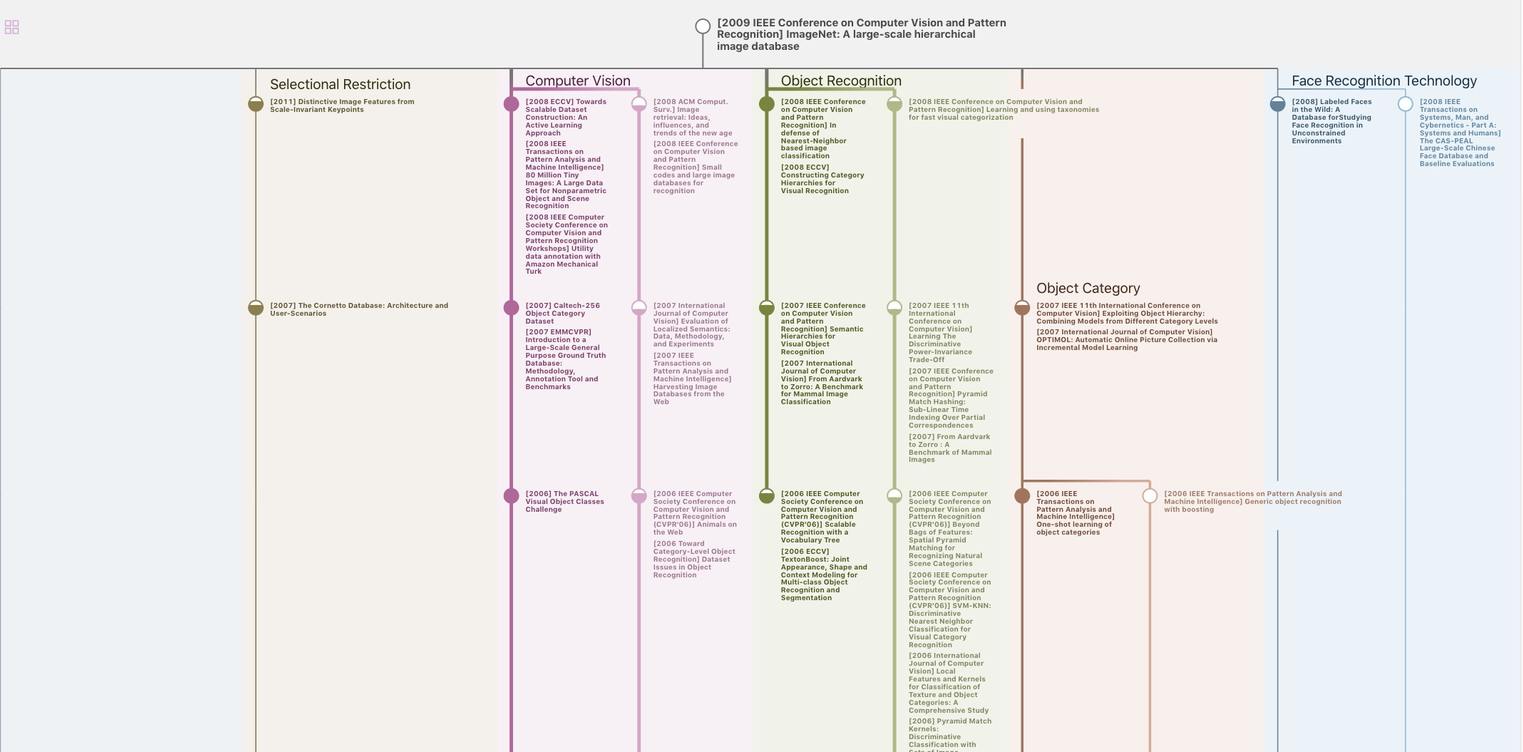
生成溯源树,研究论文发展脉络
Chat Paper
正在生成论文摘要