A Stochastic Covariance Shrinkage Approach in Ensemble Transform Kalman Filtering
TELLUS SERIES A-DYNAMIC METEOROLOGY AND OCEANOGRAPHY(2023)
摘要
The Ensemble Kalman Filters (EnKF) employ a Monte-Carlo approach to represent covariance information, and are affected by sampling errors in operational settings where the number of model realizations is much smaller than the model state dimension. To alleviate the effects of these errors EnKF relies on model-specific heuristics such as covariance localization, which takes advantage of the spatial locality of correlations among the model variables. This work proposes an approach to alleviate sampling errors that utilizes a locally averaged-in-time dynamics of the model, described in terms of a climatological covariance of the dynamical system. We use this covariance as the target matrix in covariance shrinkage methods, and develop a stochastic covariance shrinkage approach where synthetic ensemble members are drawn to enrich both the ensemble subspace and the ensemble transformation. We additionally provide for a way in which this methodology can be localized similar to the state-of-the-art LETKF method, and that for a certain model setup, our methodology significantly outperforms it.
更多查看译文
关键词
ensemble Kalman filter, covariance shrinkage, data assimilation, synthetic ensemble, localization
AI 理解论文
溯源树
样例
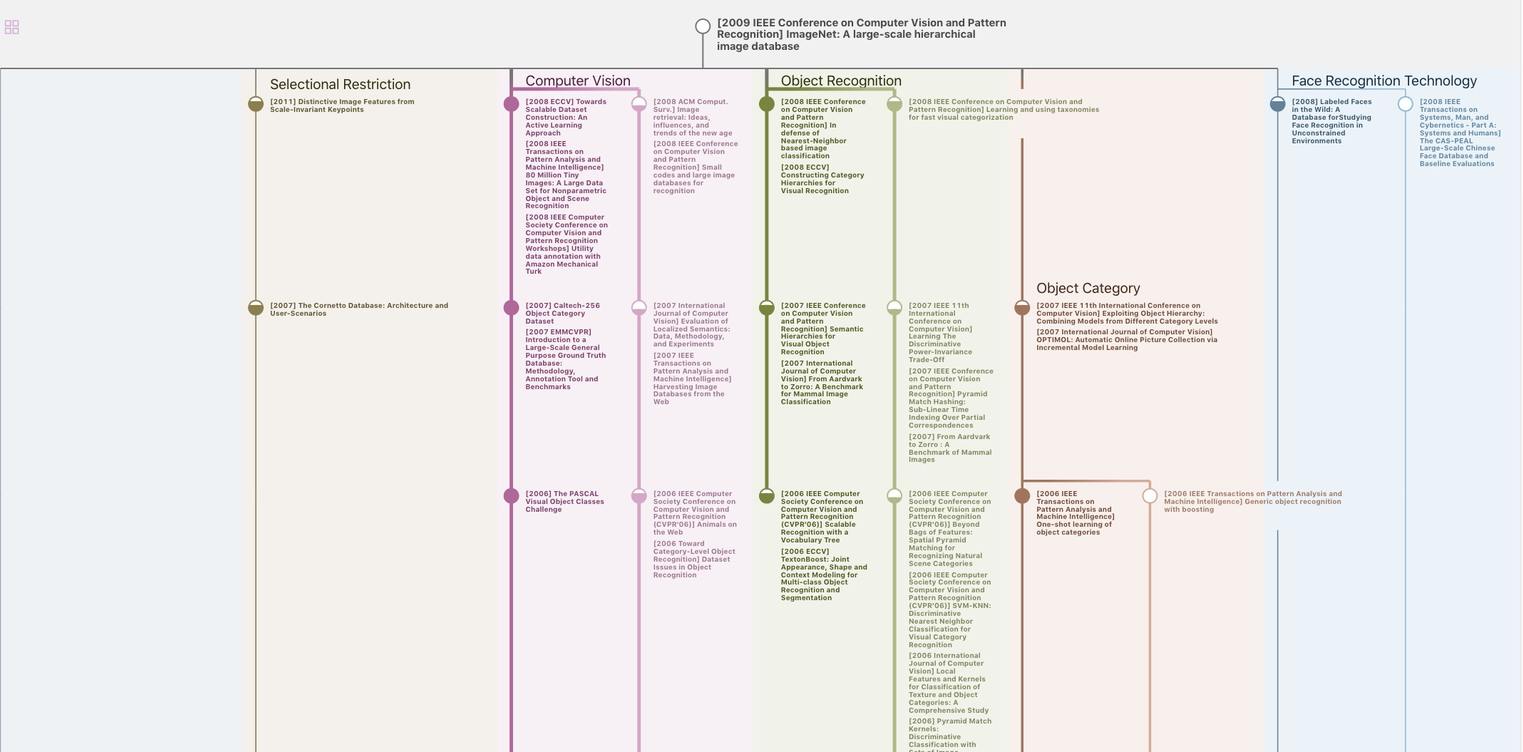
生成溯源树,研究论文发展脉络
Chat Paper
正在生成论文摘要