Uncertainty-aware and explainable machine learning for early prediction of battery degradation
crossref(2022)
摘要
Enhancing lifetime is a vital aspect in battery design and development. Lifetime evaluation requires prolonged cycling experiments. Early prediction of battery cell ageing can accelerate the development timeline as well as optimal charging schedule planning, and battery cell and pack production towards an extended lifetime. We demonstrate that an autoregressive model can be trained with limited data for early prediction capacity. Our approach robustly models the capacity degradation over the entire lifetime and outperforms previous approaches in accuracy for EOL (end of life) prediction. Our model captures the uncertainty in the prediction, allowing appropriate and reliable deployment. Explainability analysis of the proposed deep model provides cognizance of the interplay between multiple cell degradation mechanisms. Finally, we show that the model aligns with existing chemical insights into the rationale for early EOL despite not being trained for this or having received prior chemical knowledge.
更多查看译文
AI 理解论文
溯源树
样例
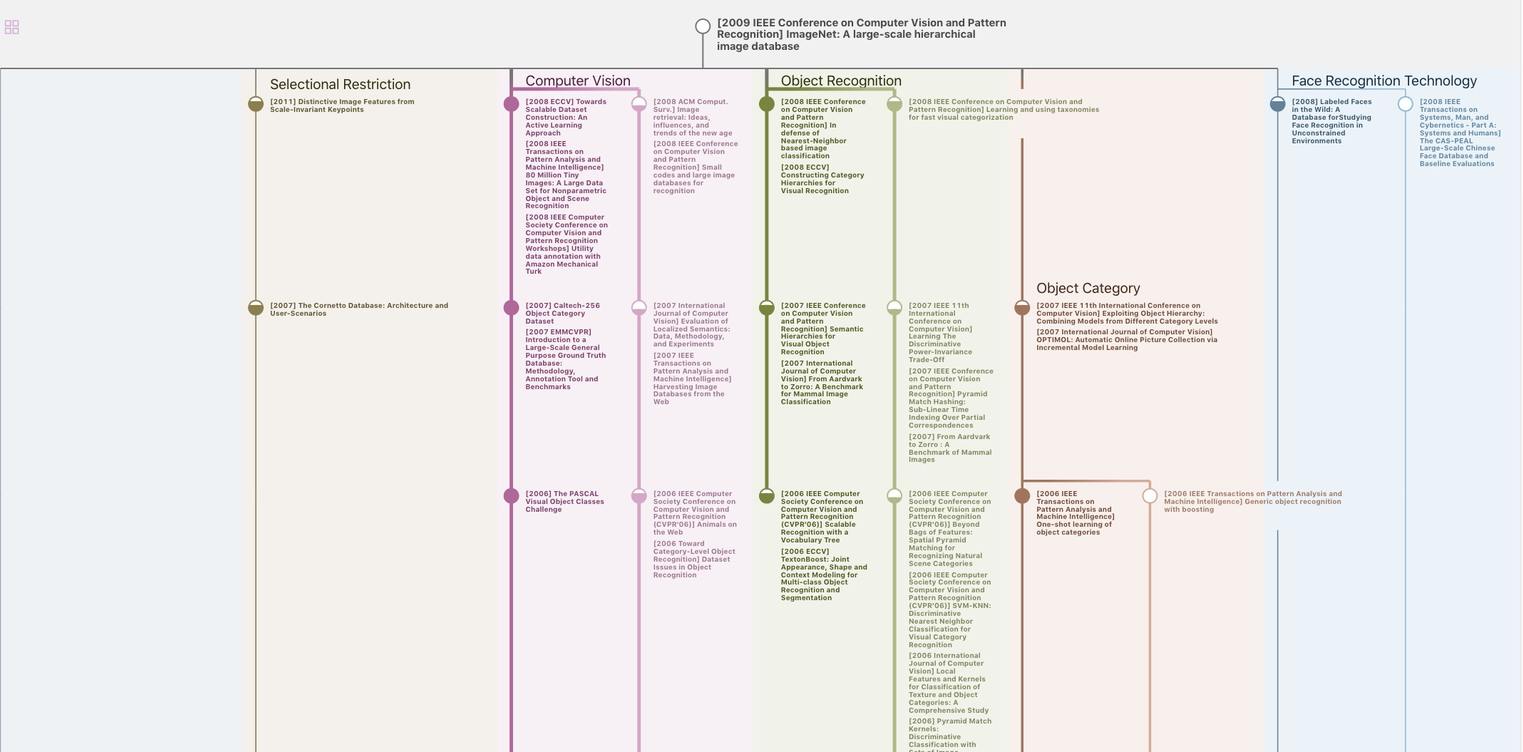
生成溯源树,研究论文发展脉络
Chat Paper
正在生成论文摘要