Differentially private knowledge transfer for federated learning
Nature Communications(2023)
摘要
Extracting useful knowledge from big data is important for machine learning. When data is privacy-sensitive and cannot be directly collected, federated learning is a promising option that extracts knowledge from decentralized data by learning and exchanging model parameters, rather than raw data. However, model parameters may encode not only non-private knowledge but also private information of local data, thereby transferring knowledge via model parameters is not privacy-secure. Here, we present a knowledge transfer method named PrivateKT, which uses actively selected small public data to transfer high-quality knowledge in federated learning with privacy guarantees. We verify PrivateKT on three different datasets, and results show that PrivateKT can maximally reduce 84% of the performance gap between centralized learning and existing federated learning methods under strict differential privacy restrictions. PrivateKT provides a potential direction to effective and privacy-preserving knowledge transfer in machine intelligent systems.
更多查看译文
关键词
private knowledge transfer,learning
AI 理解论文
溯源树
样例
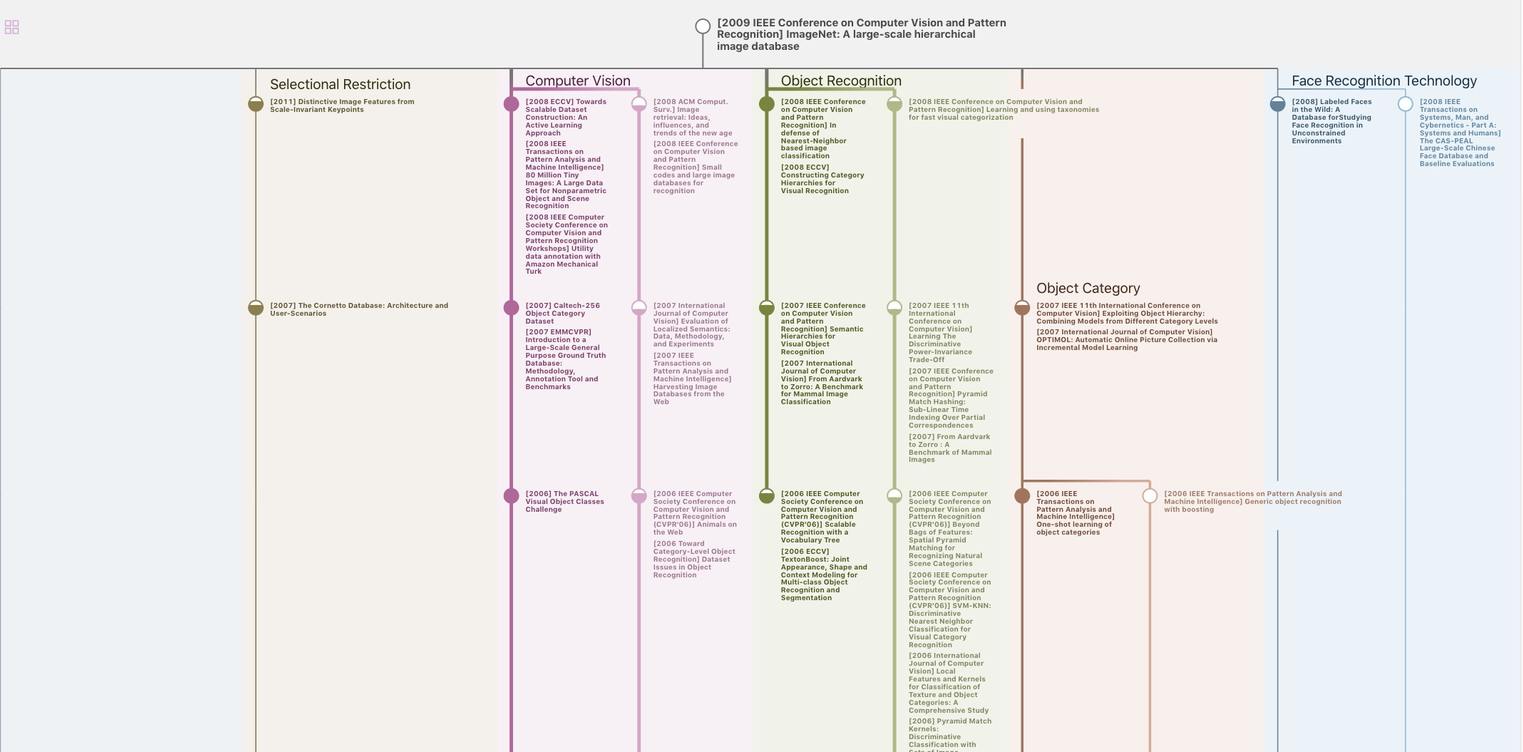
生成溯源树,研究论文发展脉络
Chat Paper
正在生成论文摘要