A Task-Driven Scene-Aware LiDAR Point Cloud Coding Framework for Autonomous Vehicles
IEEE Transactions on Industrial Informatics(2022)
摘要
LiDAR sensors are almost indispensable for autonomous robots to perceive the surrounding environment. However, the transmission of large-scale LiDAR point clouds is highly bandwidth-intensive, which can easily lead to transmission problems, especially for unstable communication networks. Meanwhile, existing LiDAR data compression is mainly based on rate-distortion optimization, which ignores the semantic information of ordered point clouds and the task requirements of autonomous robots. To address these challenges, this article presents a task-driven Scene-Aware LiDAR Point Clouds Coding (SA-LPCC) framework for autonomous vehicles. Specifically, a semantic segmentation model is developed based on multidimension information, in which both 2-D texture and 3-D topology information are fully utilized to segment movable objects. Furthermore, a prediction-based deep network is explored to remove the spatial–temporal redundancy. The experimental results on the benchmark semantic KITTI dataset validate that our SA-LPCC achieves state-of-the-art performance in terms of the reconstruction quality and storage space for downstream tasks. We believe that SA-LPCC jointly considers the scene-aware characteristics of movable objects and removes the spatial–temporal redundancy from an end-to-end learning mechanism, which will boost the related applications from algorithm optimization to industrial products.
更多查看译文
关键词
Autonomous vehicles,LiDAR point clouds,semantic segmentation
AI 理解论文
溯源树
样例
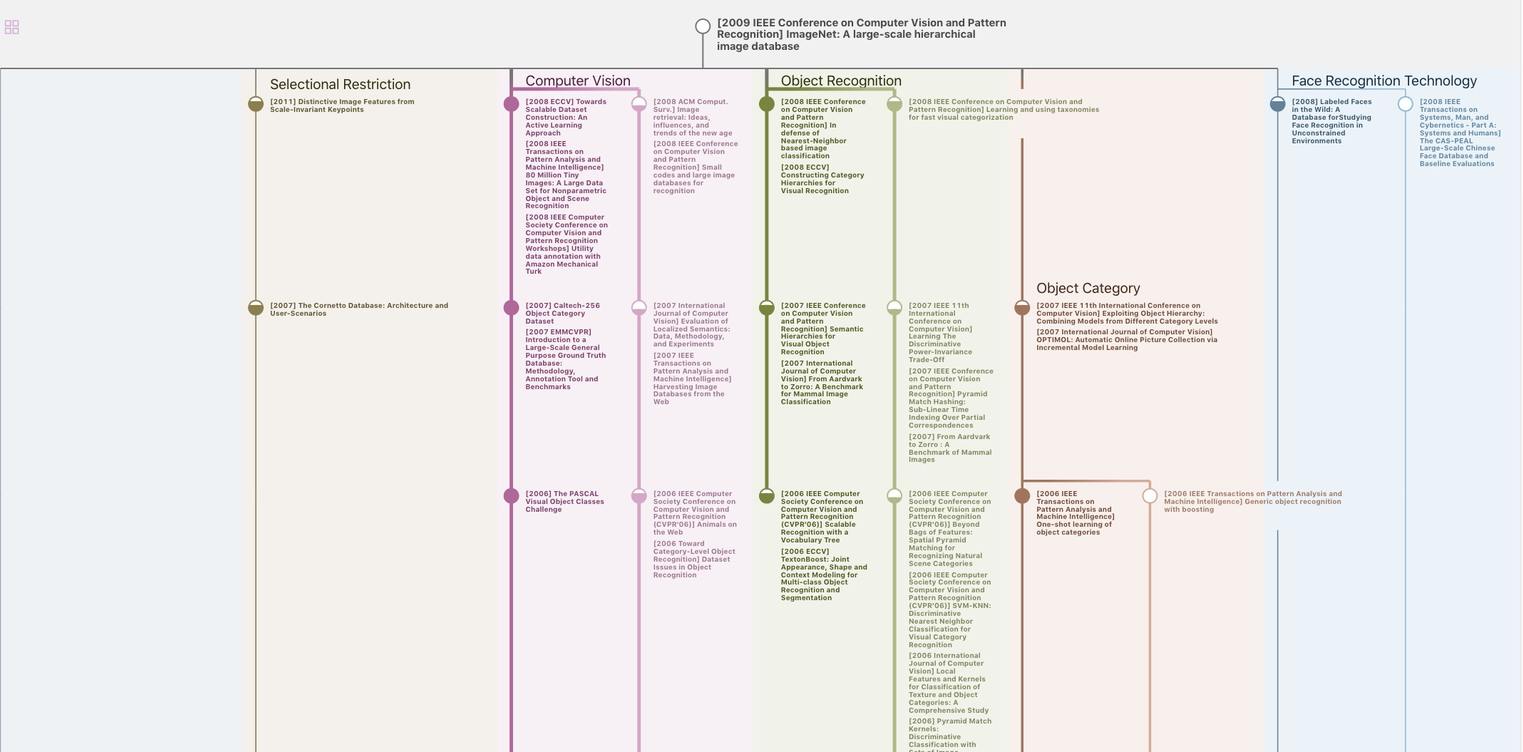
生成溯源树,研究论文发展脉络
Chat Paper
正在生成论文摘要