“The Actual Model Itself…It’s Actually Not That Important”: A Multi-Stakeholder Qualitative Analysis of a Machine Learning-Algorithm Integration (Preprint)
crossref(2022)
摘要
BACKGROUND Machine-learning (ML) driven computerized decision support (CDS) continues to draw wide interest and investment as a means of improving care quality and value, despite mixed real-world implementation outcomes. OBJECTIVE This study aims to explore barriers to and facilitators of the integration of a peripheral arterial disease (PAD) identification algorithm to implement timely guideline-based care. METHODS Twelve semi-structured interviews were completed with individuals from three stakeholder groups during the first four weeks of integration of a ML CDS-enabled workflow in which an interdisciplinary team used the ML-driven CDS to identify patients with PAD, develop a recommended action plan, and send this recommendation to the patient’s primary care provider (PCP). Pseudonymized transcripts were coded and thematic analysis was completed by a multidisciplinary research team. RESULTS Three themes were identified: Facilitators of translating in-silico performance to real-world efficacy, Organizational and data structure barriers to clinical impact, and Potential barriers to advancing equity. Success criteria for each stakeholder group were also characterized to better understand how teams work together to integrate ML-driven CDS. CONCLUSIONS Longitudinal multi-stakeholder engagement in the development and integration of ML-driven CDS supports effective translation into real-world care. This more holistic perspective also permits more effective detection of context-driven healthcare inequities, which are uncovered or exacerbated through ML-driven CDS integration. Many of the solutions to these inequities lie outside the scope of ML and require coordinated systematic solutions for mitigation. CLINICALTRIAL None.
更多查看译文
AI 理解论文
溯源树
样例
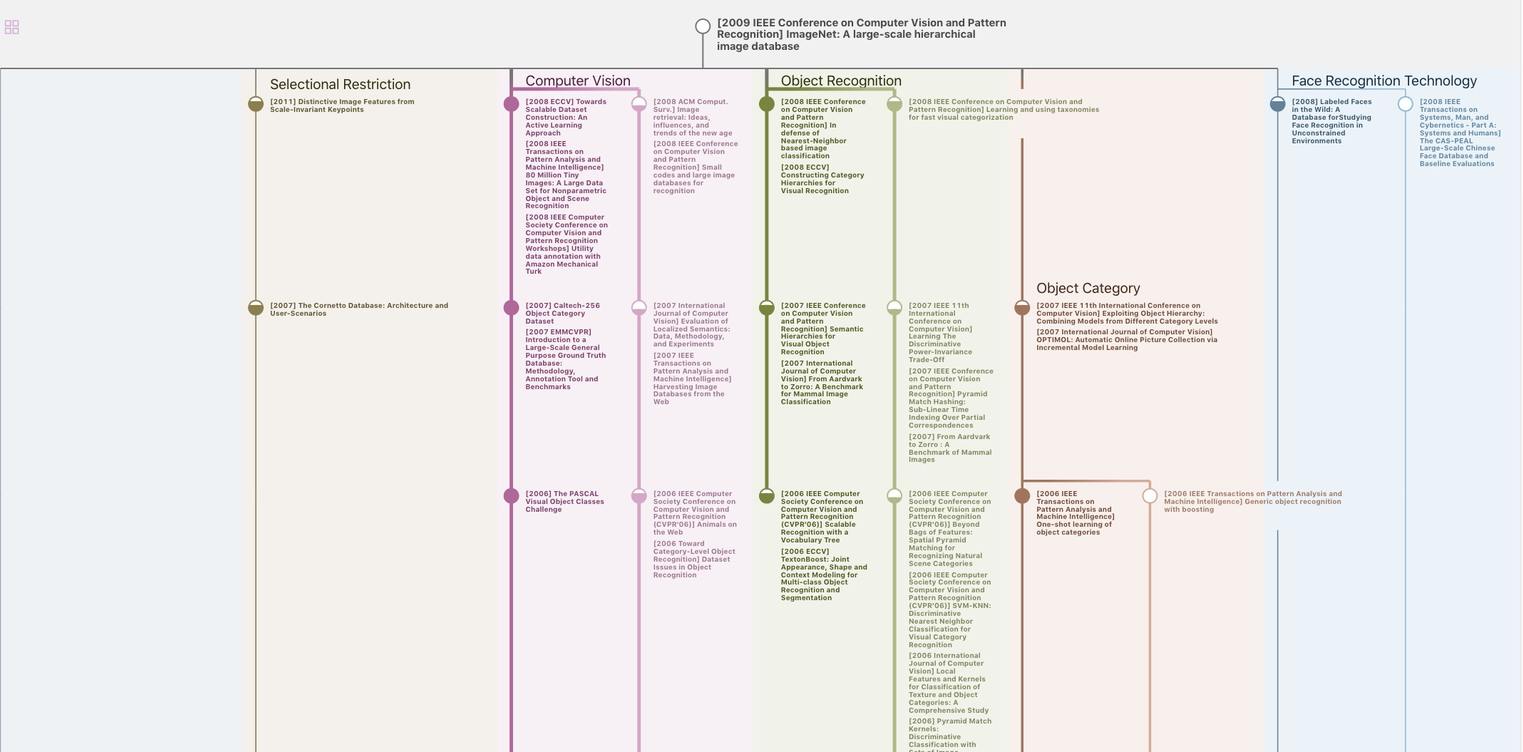
生成溯源树,研究论文发展脉络
Chat Paper
正在生成论文摘要